Generative Diffusion Models for Antibody Design, Docking, and Optimization
bioRxiv (Cold Spring Harbor Laboratory)(2023)
摘要
Abstract In recent years, optimizing antibody binding affinity for biomedical applications has become increasingly important. However, traditional wet-experiment-based approaches are time-consuming and inefficient. To address this issue, we propose a diffusion model-based antibody optimization pipeline to improve binding affinity. Our approach involves two key models: AbDesign for designing antibody sequences and structures, and AbDock, a paratope-epitope docking model, used for screening designed CDRs. On an independent test set, our AbDesign demonstrates the exceptional performance of an RMSD of 2.56Å in structure design and an amino acid recovery of 36.47% in sequence design. In a paratope-epitope docking test set, our AbDock achieves a state-of-the-art performance of DockQ 0.44, irms 2.71Å, fnat 0.40, and Lrms 6.29Å. The effectiveness of the optimization pipeline is further experimentally validated by optimizing a flaviviruse antibody 1G5.3, resulting in a broad-spectrum antibody that demonstrates improved binding to 6 out of the nine tested flaviviruses. This research offers a general-purpose methodology to enhance antibody functionality without training on data from specific antigens.
更多查看译文
关键词
antibody design,diffusion,docking,optimization
AI 理解论文
溯源树
样例
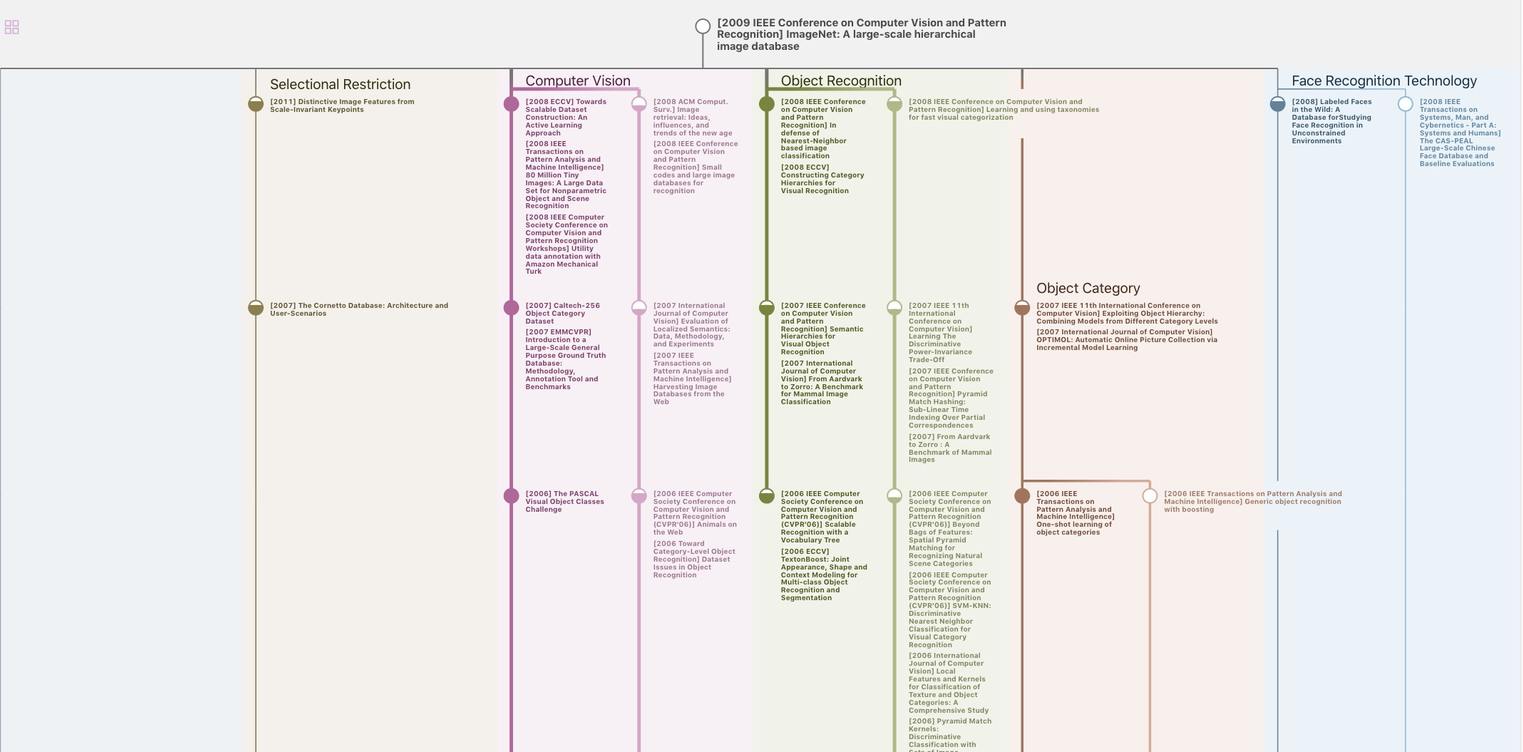
生成溯源树,研究论文发展脉络
Chat Paper
正在生成论文摘要