Space-invariant projection in streaming network embedding
Information Sciences(2023)
摘要
The introduction of new nodes in dynamic networks gradually leads to drift in the node embedding space, and the retraining of node embeddings and downstream models is indispensable. The exact threshold of these new nodes, below which the node embedding space will be maintained, however, is rarely considered from theoretical or experimental perspectives. When considering the matrix perturbation theory, the threshold for the maximum number of new nodes to keep the node embedding space approximately equivalent is analytically provided and empirically validated. It is therefore theoretically guaranteed that when the number of new nodes is below this threshold, the embeddings of these new nodes can be quickly derived from the embeddings of the original nodes. A generation framework, space-invariant projection (SIP), is accordingly proposed to enable arbitrary static matrix factorization-based embedding schemes to quickly embed new nodes in dynamic networks. The time complexity of the SIP framework is linear with the network size. By combining SIP with four state-of-the-art MF (matrix factorization) -based schemes, we show that SIP exhibits not only wide adaptability but also strong empirical performance in terms of efficiency and efficacy in both the node classification and link prediction tasks with different real-world datasets.
更多查看译文
关键词
Dynamic network embedding,Node embedding,Matrix factorization,Matrix perturbation theory,Node classification,Link prediction
AI 理解论文
溯源树
样例
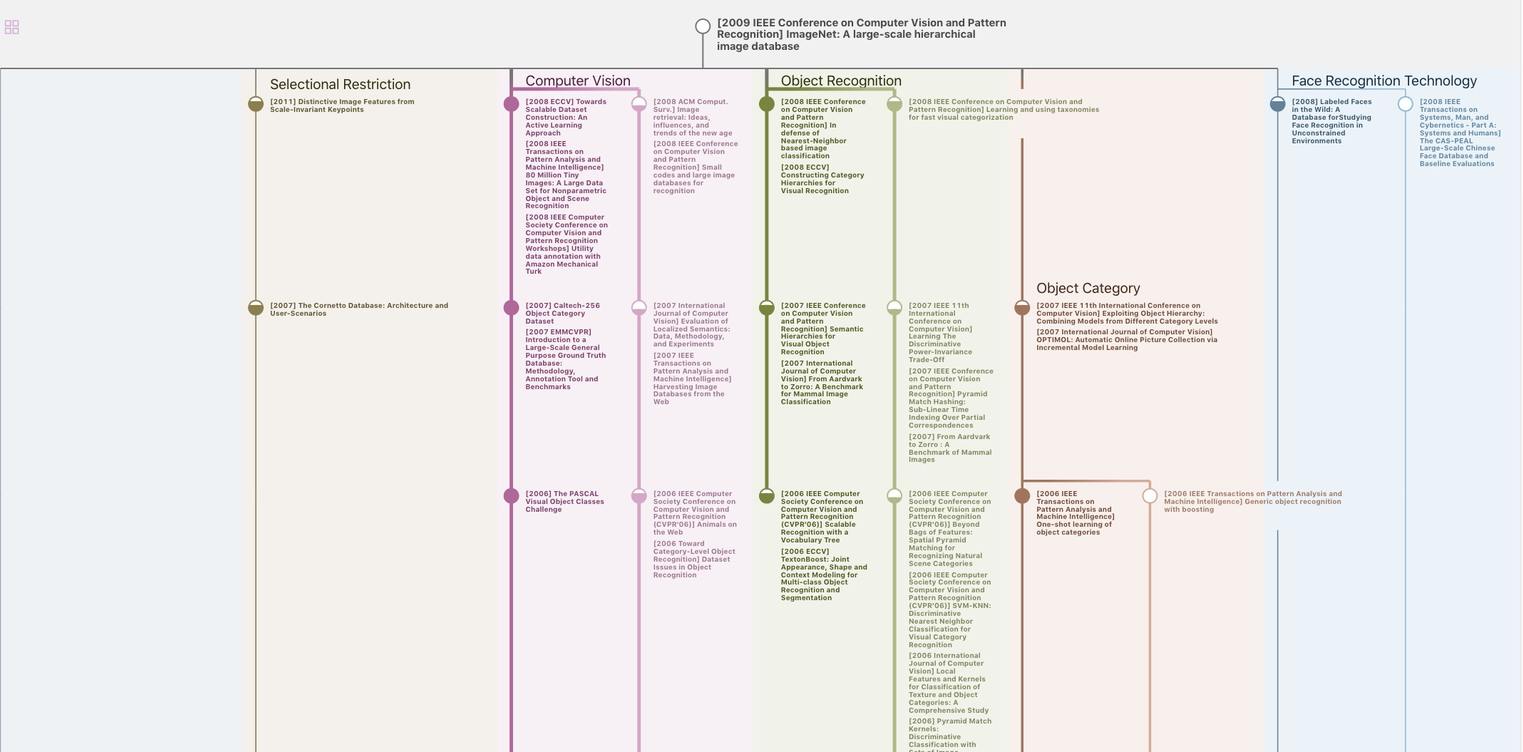
生成溯源树,研究论文发展脉络
Chat Paper
正在生成论文摘要