A Chebyshev Confidence Guided Source-Free Domain Adaptation Framework for Medical Image Segmentation.
IEEE journal of biomedical and health informatics(2024)
Abstract
Source-free domain adaptation (SFDA) aims to adapt models trained on a labeled source domain to an unlabeled target domain without access to source data. In medical imaging scenarios, the practical significance of SFDA methods has been emphasized due to data heterogeneity and privacy concerns. Recent state-of-the-art SFDA methods primarily rely on self-training based on pseudo-labels (PLs). Unfortunately, the accuracy of PLs may deteriorate due to domain shift, thus limiting the effectiveness of the adaptation process. To address this issue, we propose a Chebyshev confidence guided SFDA framework to accurately assess the reliability of PLs and generate self-improving PLs for self-training. The Chebyshev confidence is estimated by calculating the probability lower bound of PL confidence, given the prediction and the corresponding uncertainty. Leveraging the Chebyshev confidence, we introduce two confidence-guided denoising methods: direct denoising and prototypical denoising. Additionally, we propose a novel teacher-student joint training scheme (TJTS) that incorporates a confidence weighting module to iteratively improve PLs' accuracy. The TJTS, in collaboration with the denoising methods, effectively prevents the propagation of noise and enhances the accuracy of PLs. Extensive experiments in diverse domain scenarios validate the effectiveness of our proposed framework and establish its superiority over state-of-the-art SFDA methods. Our paper contributes to the field of SFDA by providing a novel approach for precisely estimating the reliability of PLs and a framework for obtaining high-quality PLs, resulting in improved adaptation performance.
MoreTranslated text
Key words
Source-free domain adaptation,Image segmentation,Self-training,Pseudo-label denoising,
AI Read Science
Must-Reading Tree
Example
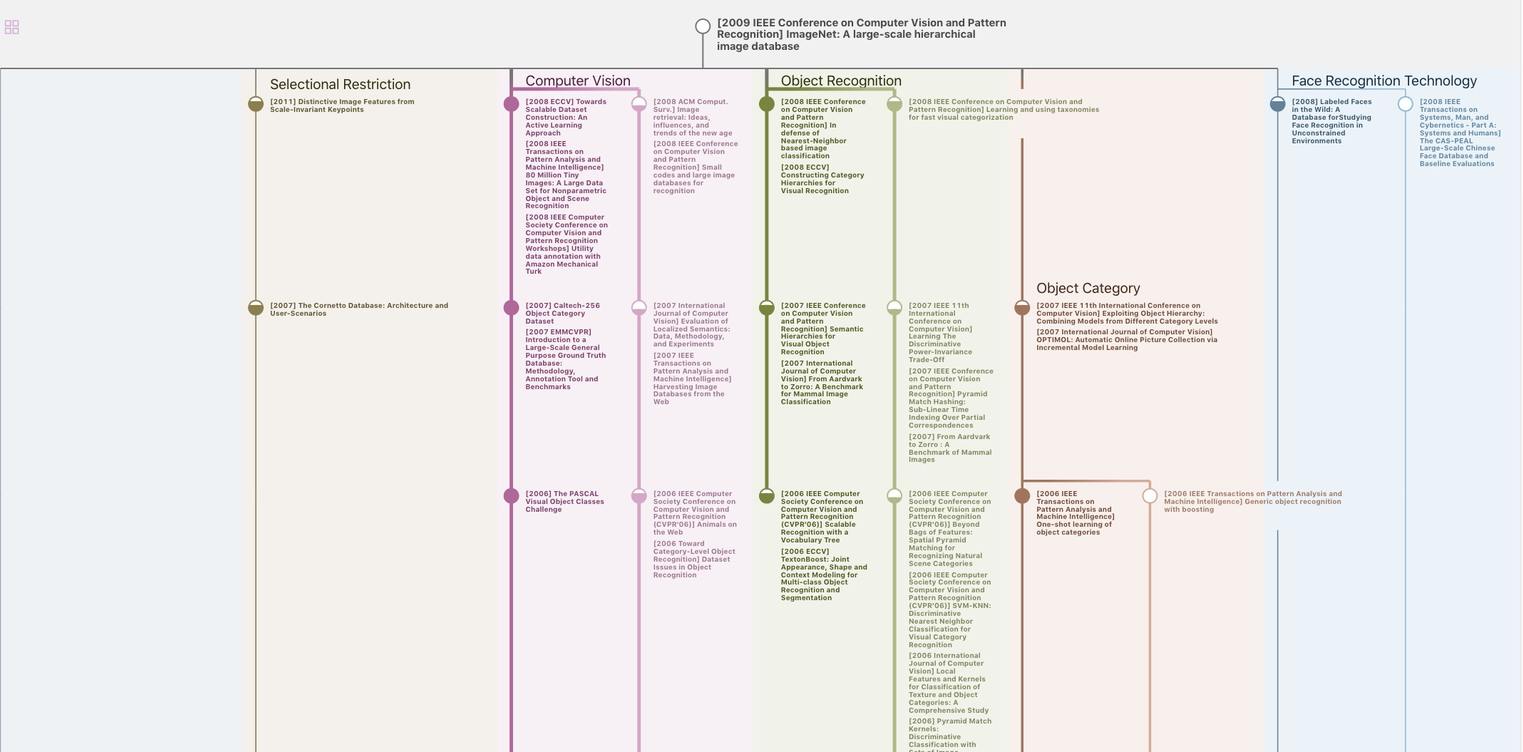
Generate MRT to find the research sequence of this paper
Chat Paper
Summary is being generated by the instructions you defined