Can LLMs Keep a Secret? Testing Privacy Implications of Language Models via Contextual Integrity Theory
ICLR 2024(2023)
摘要
The interactive use of large language models (LLMs) in AI assistants (at work, home, etc.) introduces a new set of inference-time privacy risks: LLMs are fed different types of information from multiple sources in their inputs and are expected to reason about what to share in their outputs, for what purpose and with whom, within a given context. In this work, we draw attention to the highly critical yet overlooked notion of contextual privacy by proposing ConfAIde, a benchmark designed to identify critical weaknesses in the privacy reasoning capabilities of instruction-tuned LLMs. Our experiments show that even the most capable models such as GPT-4 and ChatGPT reveal private information in contexts that humans would not, 39% and 57% of the time, respectively. This leakage persists even when we employ privacy-inducing prompts or chain-of-thought reasoning. Our work underscores the immediate need to explore novel inference-time privacy-preserving approaches, based on reasoning and theory of mind.
更多查看译文
关键词
Contextual Integrity,Privacy,Theory of Mind
AI 理解论文
溯源树
样例
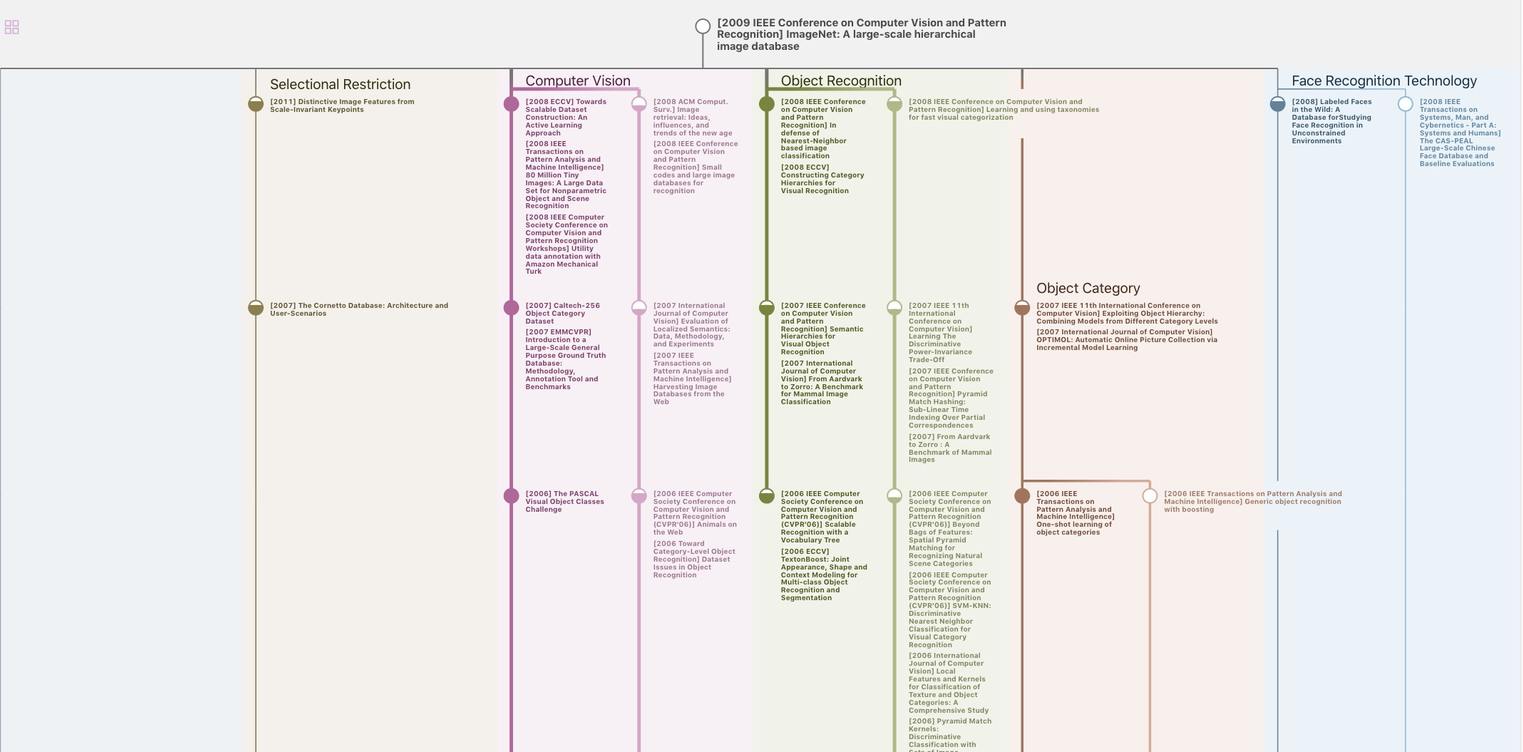
生成溯源树,研究论文发展脉络
Chat Paper
正在生成论文摘要