Lane change trajectory prediction considering driving style uncertainty for autonomous vehicles
MECHANICAL SYSTEMS AND SIGNAL PROCESSING(2024)
摘要
Lane change trajectory prediction is crucial for autonomous vehicles (AVs) to assess their own driving safety in advance. However, there are significant uncertainties in the implementation of such prediction, including different behaviors caused by agent-agent interaction and driving styles of drivers. While prototype trajectories can serve as a means to represent typical motion patterns and enhance trajectory prediction performance, their utilization in modeling motion patterns tends to overlook the influence of agent-agent interactions and vehicle dynamics. This paper proposes a fusion algorithm that considers driving style and vehicle dynamics to address these uncertainties. The algorithm involves a long short-term memory (LSTM) lane change behavior recognition model that mines key features of agent-agent interaction through the attention mechanism. The Gaussian process (GP) motion modeling trajectory prediction (GPMM-TP) algorithm considers the driving style of the prototype trajectory based on the behavior recognition results. To further improve short-term and long-term prediction, the interactive multi-model (IMM) algorithm is used to assign probability weights to the GP model and the Extended Kalman Filter (EKF) model based on prediction accuracy, taking into account the driving styles and the vehicle dynamics. The proposed algorithm provides a promising approach to improving the accuracy of lane change trajectory prediction for AVs, and its effectiveness is demonstrated using the HighD dataset.
更多查看译文
关键词
Attention mechanisms,Lane change behavioral recognition,Driving style,Gaussian processes,Interactive multi-model,Trajectory prediction
AI 理解论文
溯源树
样例
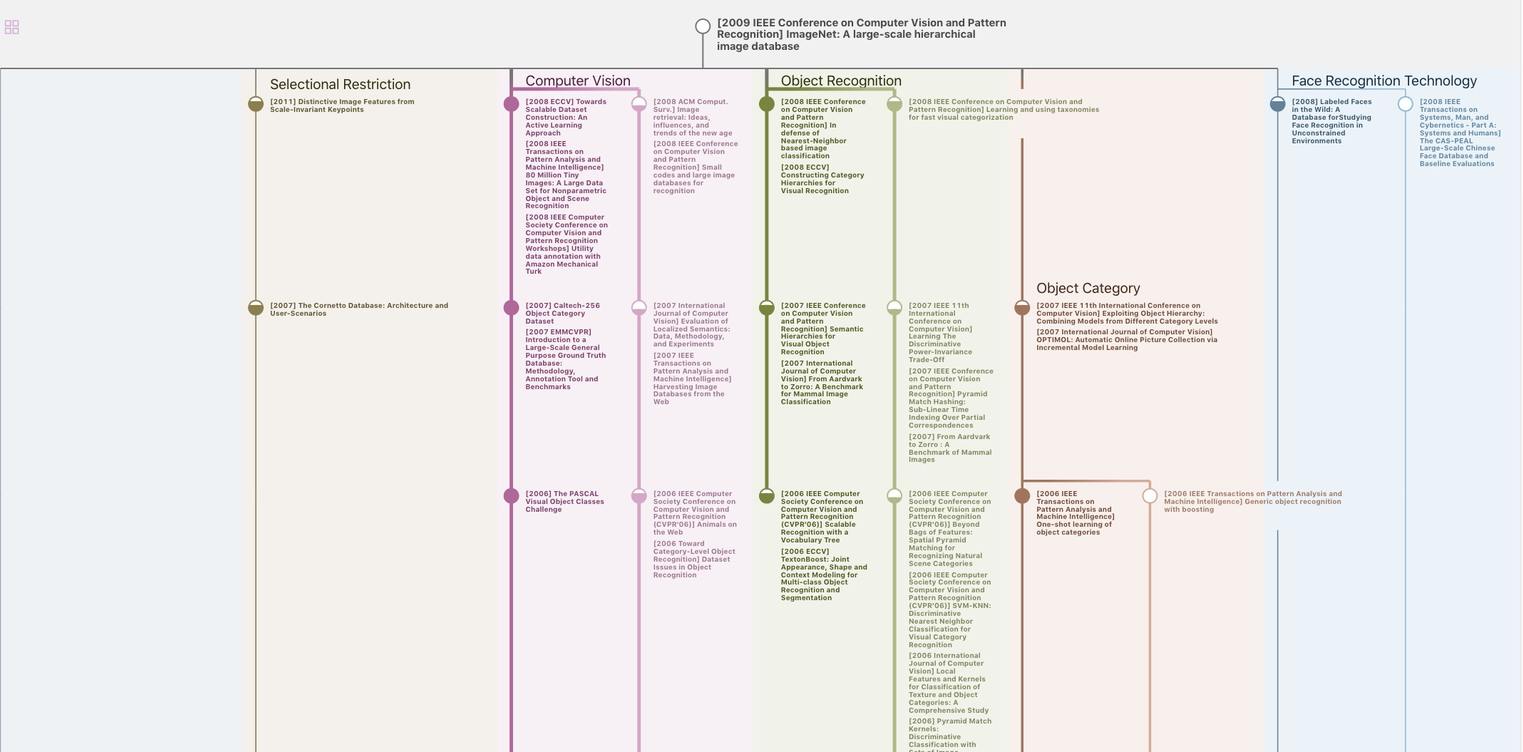
生成溯源树,研究论文发展脉络
Chat Paper
正在生成论文摘要