Underwater Source Counting with Local-Confidence-Level-Enhanced Density Clustering.
Sensors (Basel, Switzerland)(2023)
摘要
Source counting is the key procedure of autonomous detection for underwater unmanned platforms. A source counting method with local-confidence-level-enhanced density clustering using a single acoustic vector sensor (AVS) is proposed in this paper. The short-time Fourier transforms (STFT) of the sound pressure and vibration velocity measured by the AVS are first calculated, and a data set is established with the direction of arrivals (DOAs) estimated from all of the time-frequency points. Then, the density clustering algorithm is used to classify the DOAs in the data set, with which the number of the clusters and the cluster centers are obtained as the source number and the DOA estimations, respectively. In particular, the local confidence level is adopted to weigh the density of each DOA data point to highlight samples with the dominant sources and downplay those without, so that the differences in densities for the cluster centers and sidelobes are increased. Therefore, the performance of the density clustering algorithm is improved, leading to an improved source counting accuracy. Experimental results reveal that the enhanced source counting method achieves a better source counting performance than that of basic density clustering.
更多查看译文
关键词
acoustic vector sensor,source counting,DOA estimation,density clustering,local confidence level
AI 理解论文
溯源树
样例
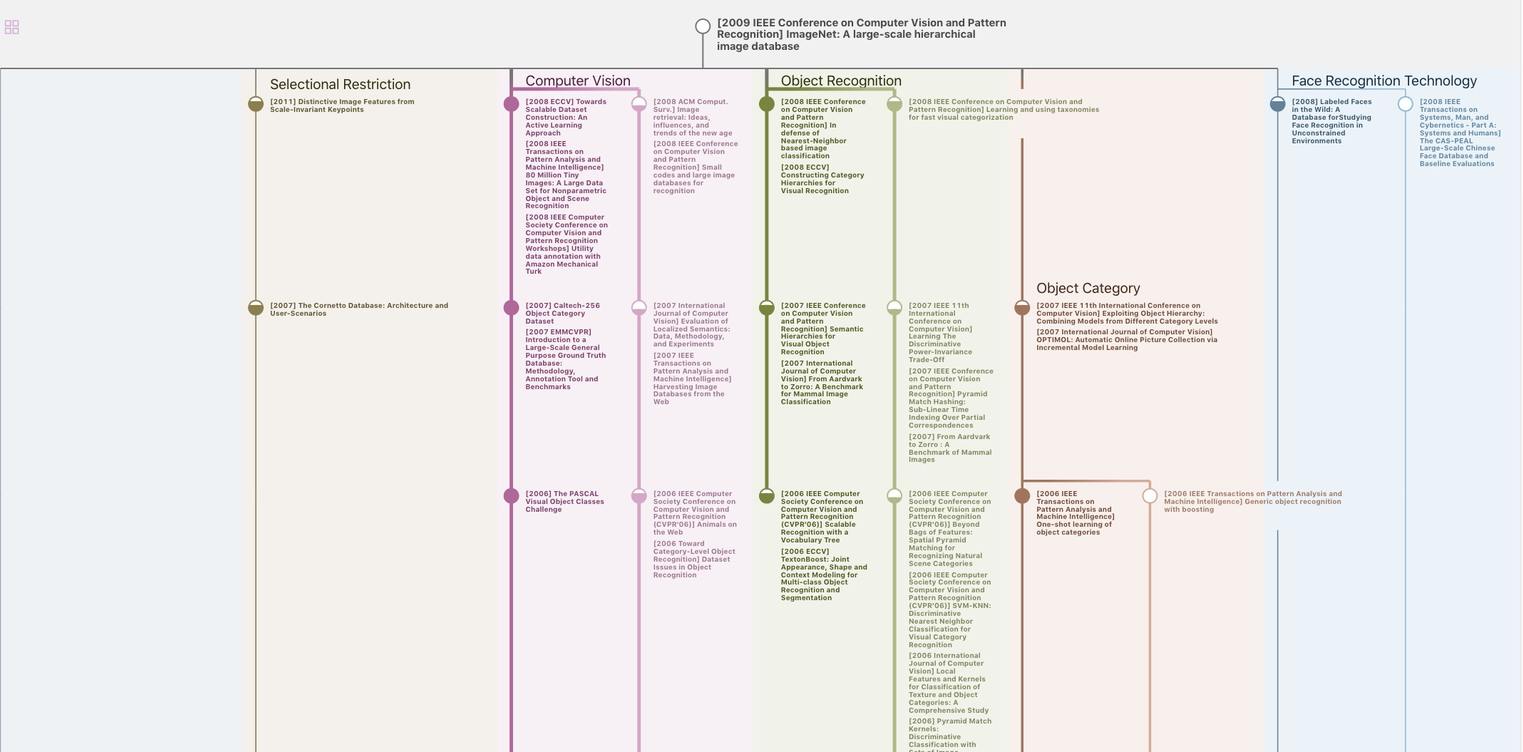
生成溯源树,研究论文发展脉络
Chat Paper
正在生成论文摘要