Multi-Modal Representation via Contrastive Learning with Attention Bottleneck Fusion and Attentive Statistics Features.
Entropy (Basel, Switzerland)(2023)
摘要
The integration of information from multiple modalities is a highly active area of research. Previous techniques have predominantly focused on fusing shallow features or high-level representations generated by deep unimodal networks, which only capture a subset of the hierarchical relationships across modalities. However, previous methods are often limited to exploiting the fine-grained statistical features inherent in multimodal data. This paper proposes an approach that densely integrates representations by computing image features' means and standard deviations. The global statistics of features afford a holistic perspective, capturing the overarching distribution and trends inherent in the data, thereby facilitating enhanced comprehension and characterization of multimodal data. We also leverage a Transformer-based fusion encoder to effectively capture global variations in multimodal features. To further enhance the learning process, we incorporate a contrastive loss function that encourages the discovery of shared information across different modalities. To validate the effectiveness of our approach, we conduct experiments on three widely used multimodal sentiment analysis datasets. The results demonstrate the efficacy of our proposed method, achieving significant performance improvements compared to existing approaches.
更多查看译文
关键词
attention bottleneck fusion,attentive statistics features,contrastive learning,multi-modal
AI 理解论文
溯源树
样例
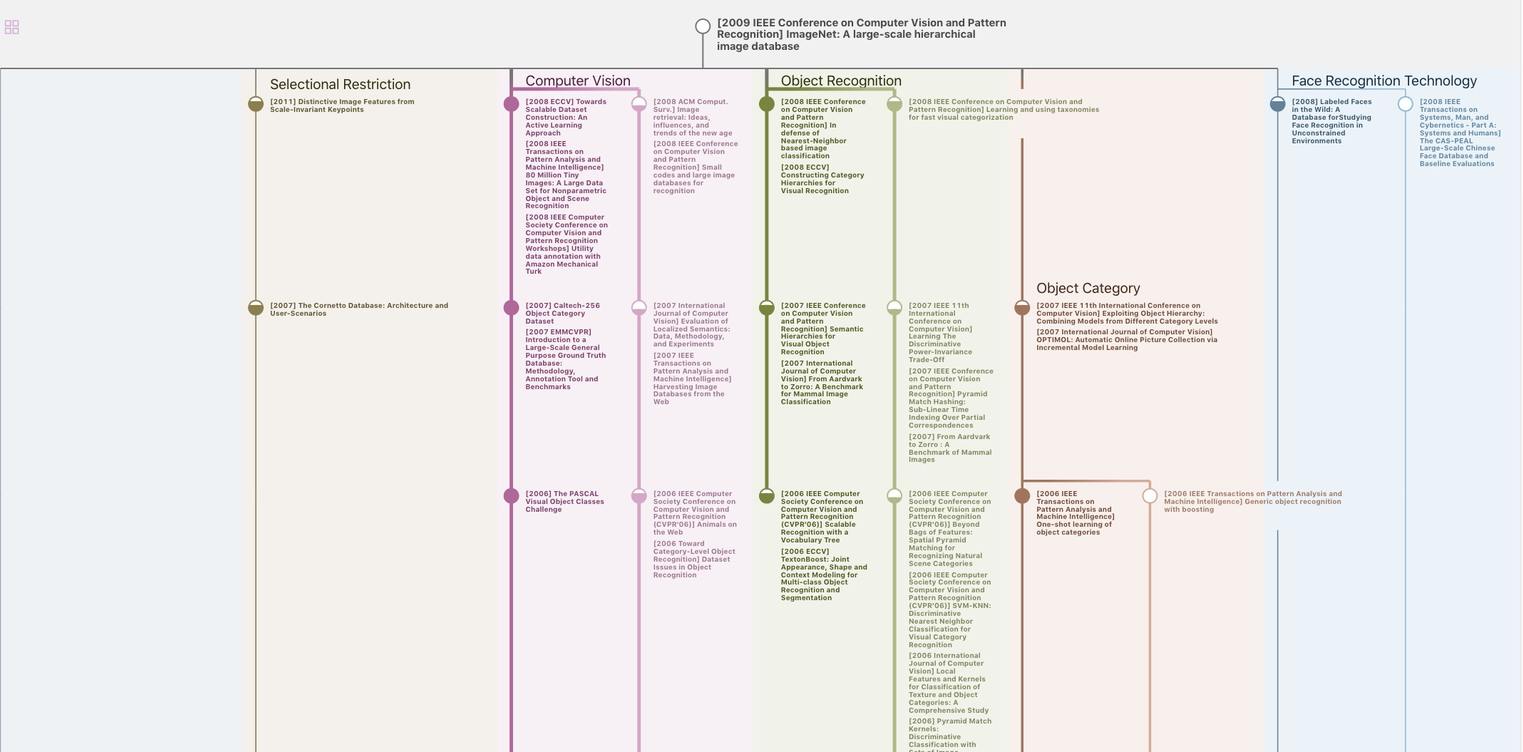
生成溯源树,研究论文发展脉络
Chat Paper
正在生成论文摘要