Deep-Learning-Based Classification of Cyclic-Alternating-Pattern Sleep Phases
Entropy (Basel, Switzerland)(2023)
摘要
Determining the cyclic-alternating-pattern (CAP) phases in sleep using electroencephalography (EEG) signals is crucial for assessing sleep quality. However, most current methods for CAP classification primarily rely on classical machine learning techniques, with limited implementation of deep-learning-based tools. Furthermore, these methods often require manual feature extraction. Herein, we propose a fully automatic deep-learning-based algorithm that leverages convolutional neural network architectures to classify the EEG signals via their time-frequency representations. Through our investigation, we explored using time-frequency analysis techniques and found that Wigner-based representations outperform the commonly used short-time Fourier transform for CAP classification. Additionally, our algorithm incorporates contextual information of the EEG signals and employs data augmentation techniques specifically designed to preserve the time-frequency structure. The model is developed using EEG signals of healthy subjects from the publicly available CAP sleep database (CAPSLPDB) on Physionet. An experimental study demonstrates that our algorithm surpasses existing machine-learning-based methods, achieving an accuracy of 77.5% on a balanced test set and 81.8% when evaluated on an unbalanced test set. Notably, the proposed algorithm exhibits efficiency and scalability, making it suitable for on-device implementation to enhance CAP identification procedures.
更多查看译文
关键词
cyclic alternating pattern (CAP),time-frequency analysis,deep neural networks,convolutional neural network (CNN),CAP sleep database (CAPSLPDB),electroencephalography (EEG)
AI 理解论文
溯源树
样例
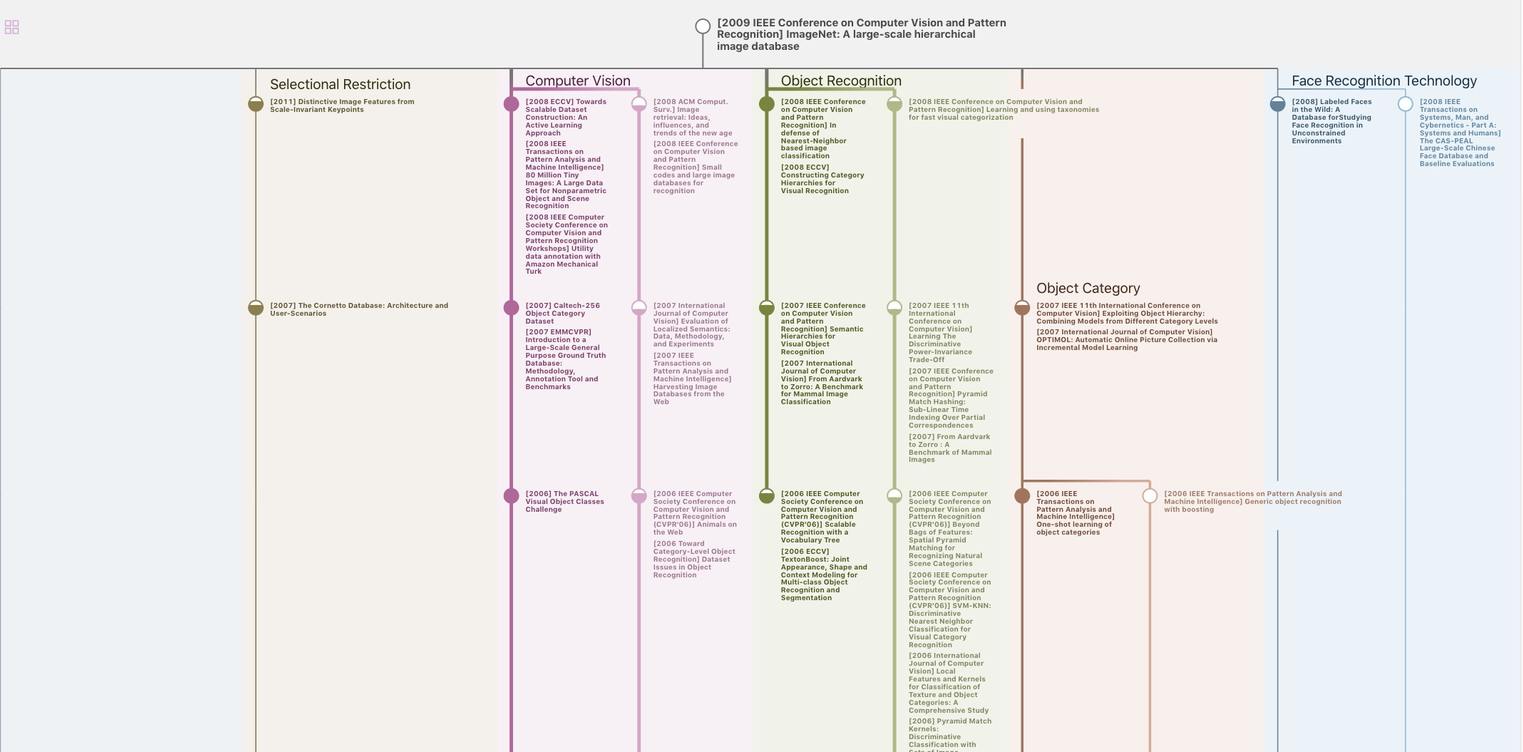
生成溯源树,研究论文发展脉络
Chat Paper
正在生成论文摘要