Estimating ammonium changes in pilot and full-scale constructed wetlands using kinetic model, linear regression, and machine learning
SCIENCE OF THE TOTAL ENVIRONMENT(2024)
摘要
Constructed wetlands (CWs) are a widely utilized nature-based wastewater treatment method for various effluents. However, their application has been more focused on pilot and full-scale CWs with substantial surface areas and extended operation times, which hold greater relevance in practical scenarios. This study used kinetics, linear regression (LR), and machine learning (ML) models to estimate effluent ammonium in pilot and full-scale CWs. From screening 1476 papers, 24 pilot and full-scale CW studies were selected to extract data containing 15 features and 975 data points. Nine models were fit to this data, revealing that linear models were less effective in capturing CW effluent compared to nonlinear ML algorithms. For training data, the Monod kinetic model predicted the poorest performance with an RMSE of 41.84 mg/L and R2 of 0.34, followed by simple LR (RMSE 24.29 mg/L and R2 0.77) and multiple LR (RMSE 22.63 mg/L and R2 0.80). In contrast, Cubist and Random Forest achieved high performances, with an average RMSE of 12.01 +/- 5.38 and an average R2 of 0.93 +/- 0.07 for Cubist, and an average RMSE of 15.94 +/- 10.69 and an average R2 of 0.91 +/- 0.08 for RF. The trained Random Forest performed the best for new data, with an R2 of 0.93 and RMSE of 13.48 mg/L. This ML-based model is a valuable tool for efficiently estimating effluent ammonium concentration in pilot and full-scale CWs, thereby facilitating the design of systems.
更多查看译文
关键词
Ammonium,Full-scale constructed wetland,Machine learning,Pilot,Kinetics,linear regression
AI 理解论文
溯源树
样例
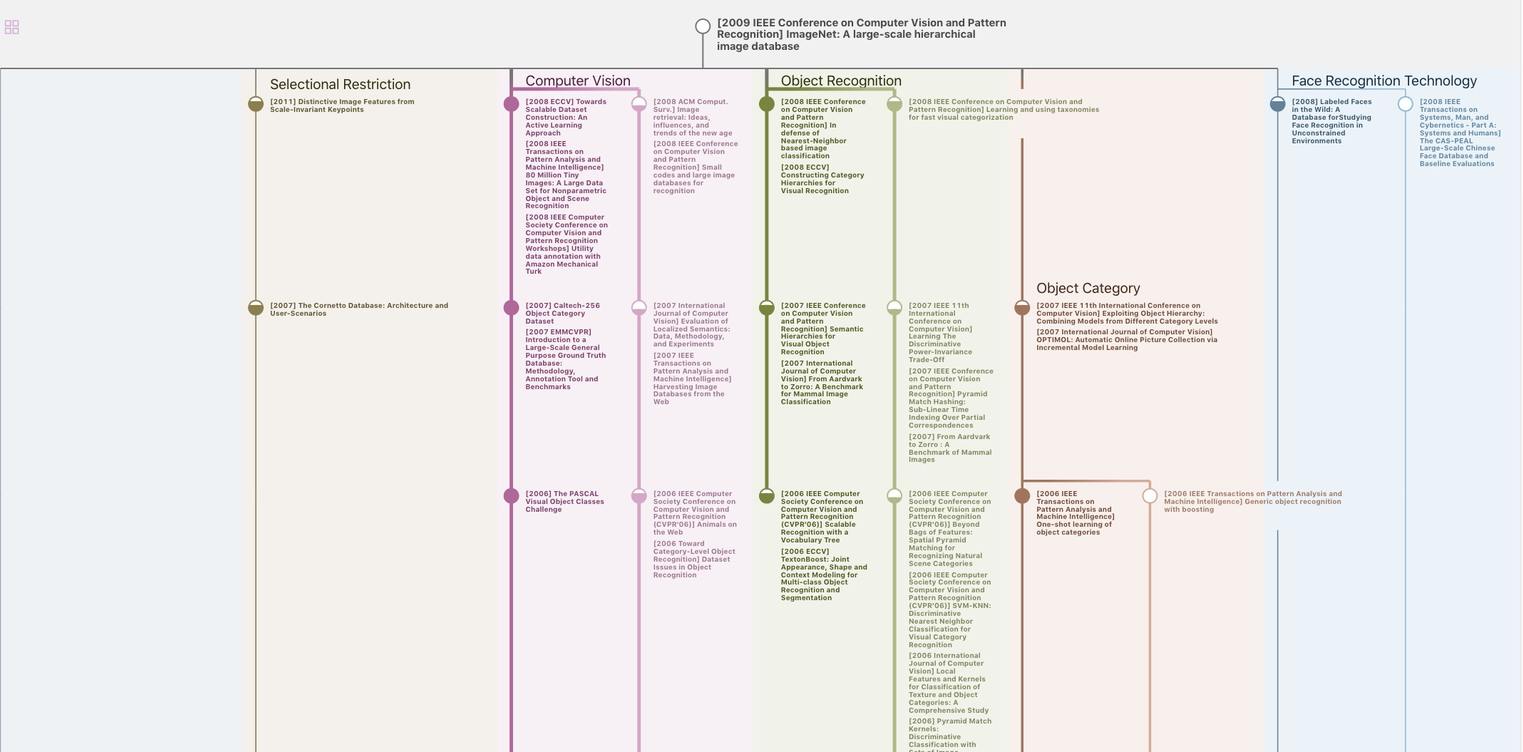
生成溯源树,研究论文发展脉络
Chat Paper
正在生成论文摘要