Short-long temporal graph convolution network for grade monitoring in a first zinc rougher
MINERALS ENGINEERING(2024)
摘要
With the rapid development of image-processing technology and neural networks, machine vision systems are being extensively used to monitor pulp grades in the froth flotation process. Specifically, an increasing number of researchers are obtaining temporal information from videos to monitor pulp grades. However, the relationship between the visual features of each video frame has not been sufficiently mined from a temporal perspective. To overcome this limitation, a short-long temporal graph convolution network (SLTGCN) trained with multiple froth videos is proposed herein to monitor the tailings grade of the first rougher layer in a zinc flotation circuit. First, the visual features of each video frame and the similarity graphs of images are treated as the nodes and edges of a graph, respectively. Thus, each froth video corresponds to a graph. Then, multiple graphs representing multiple froth videos are sent to a graph convolutional network (GCN) to predict the tailings grade. In particular, a temporal synchronous auxiliary network is proposed to ensure that the similarity graphs are consistent with the hidden features. The proposed model makes full use of the temporal information of froth videos and of the image frames in a froth video. The experimental results demonstrated the effectiveness of the proposed model. The root mean square error of the proposed model was at least 13.84% lower and its R-squared score was at least 8.62% greater than those of existing grade monitoring methods.
更多查看译文
关键词
Froth flotation,Graph convolution network,Grade monitoring,Short-long term temporal information
AI 理解论文
溯源树
样例
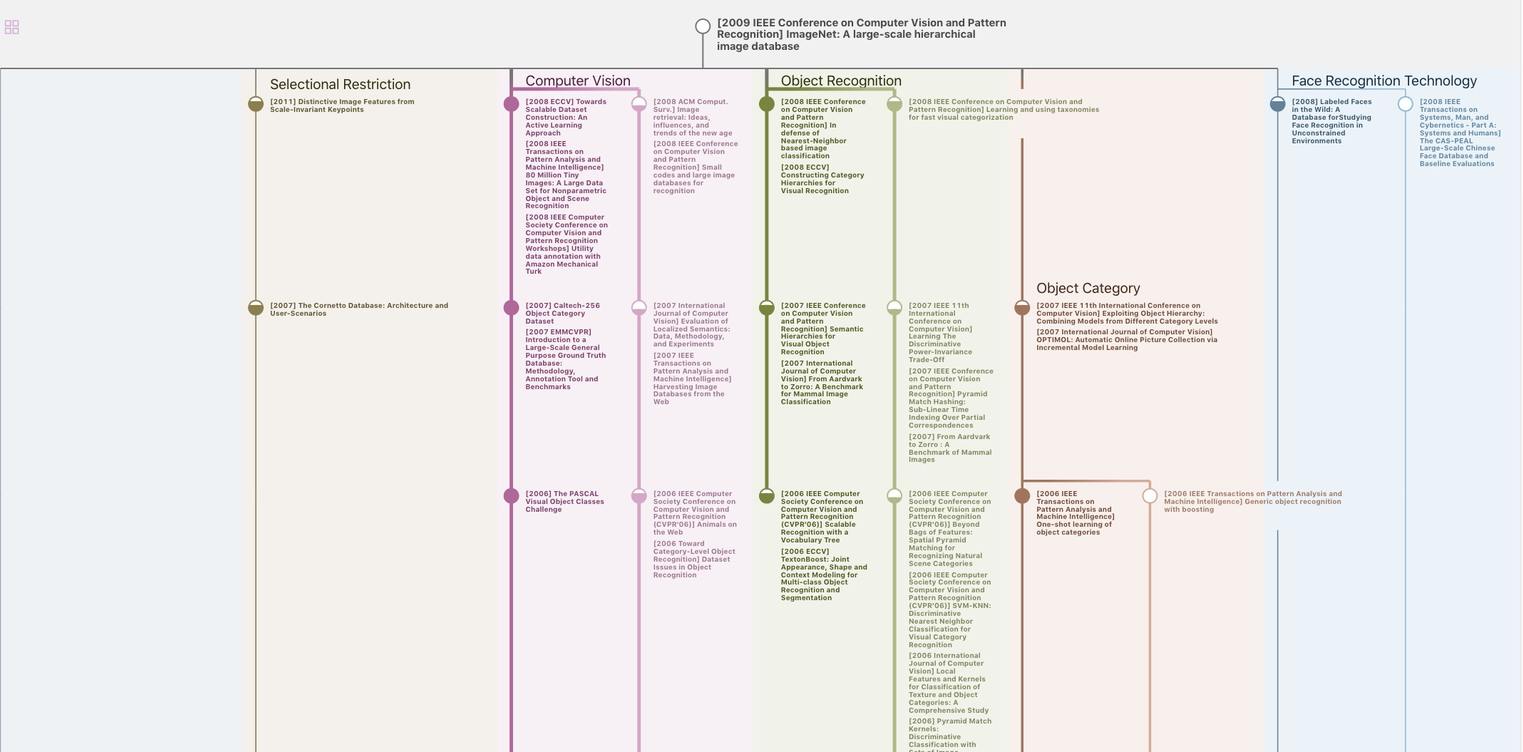
生成溯源树,研究论文发展脉络
Chat Paper
正在生成论文摘要