A Needle in a Haystack: Distinguishable Deep Neural Network Features for Domain-Agnostic Device Fingerprinting
2023 IEEE Conference on Communications and Network Security (CNS)(2023)
摘要
Deep learning (DL)-based RF fingerprinting (RFFP) methods emerged as powerful physical-layer security mechanisms, enabling automated identification of wireless devices based on unique features extracted from the RF signals. However, it is widely known that these methods fail to adapt to domain changes. This work proposes a novel IQ data representation/feature design that overcomes the domain adaption problems significantly. By accurately capturing device-specific hardware impairments, our proposed approach improves the deep learning feature selection process significantly. Extensive experimental evaluations show that the proposed approach can achieve a testing accuracy of over 99% in same-domain (day, location and channel) scenarios and of up to 95% in cross-domain scenarios, as opposed to only 55% accuracy when using the conventional IQ representation in cross-domain scenarios. The proposed representation significantly enhances the accuracy and generalizability of DL-based RFFP methods, thereby offering a transformative solution to RF data-driven device fingerprinting and identification.
更多查看译文
关键词
Device fingerprinting, datasets, domain adaptation, deep learning features, oscillator/hardware impairments
AI 理解论文
溯源树
样例
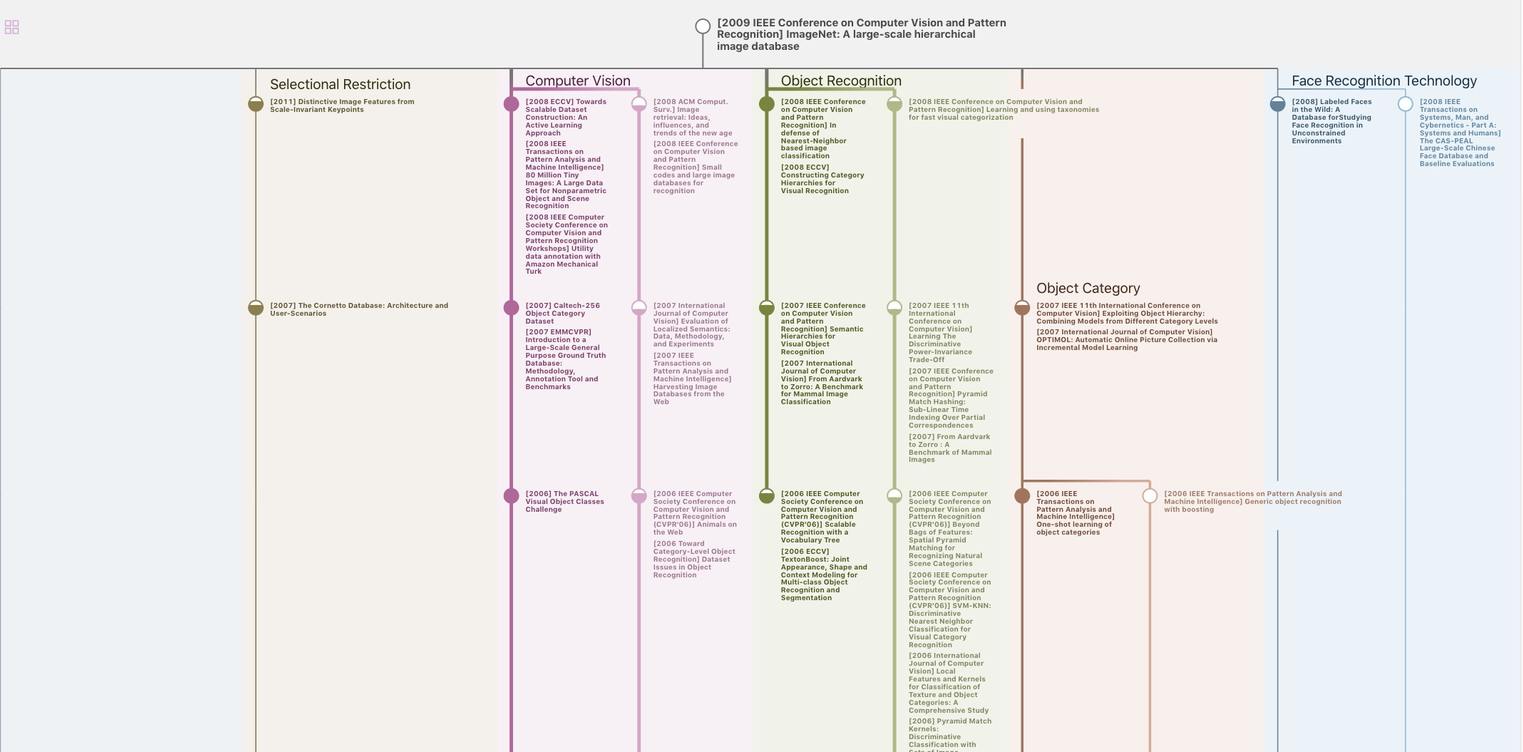
生成溯源树,研究论文发展脉络
Chat Paper
正在生成论文摘要