Preliminary Investigation of Balanced Stratified Reduction (BSR) for Imbalanced Datasets
2023 IEEE International Conference on Artificial Intelligence in Engineering and Technology (IICAIET)(2023)
摘要
The prevalence of imbalanced datasets in machine learning poses significant challenges, often leading to models with suboptimal performance, especially in recognizing underrepresented classes. This paper introduces a novel technique, Balanced Stratified Reduction (BSR), aiming to address these challenges by optimizing the sampling process. BSR utilizes horizontal stratification uniquely, drawing inspiration from the quintessence of boxplots. Preliminary results, based on two medical datasets - PIMA Indian Diabetes and Haberman's Survival, showcase BSR's potential in not only managing the class imbalance but also in retaining crucial information from the majority class. The paper outlines the foundational principles of BSR, the motivation behind its inception, a detailed experimental setup for its broader application, and preliminary findings. While the initial outcomes are promising, comprehensive evaluation and application across diverse datasets remain a focal point for future work. BSR's approach, grounded in its methodological rigor, holds promise for more effective handling of imbalanced datasets in real-world scenarios.
更多查看译文
关键词
Imbalanced Datasets,Balanced Stratified Reduction (BSR),Sampling Techniques,Quartile-based Stratification,Machine Learning,Data Preprocessing,PIMA Indian Diabetes,Haberman's Survival,Class Imbalance,Evaluation Metrics
AI 理解论文
溯源树
样例
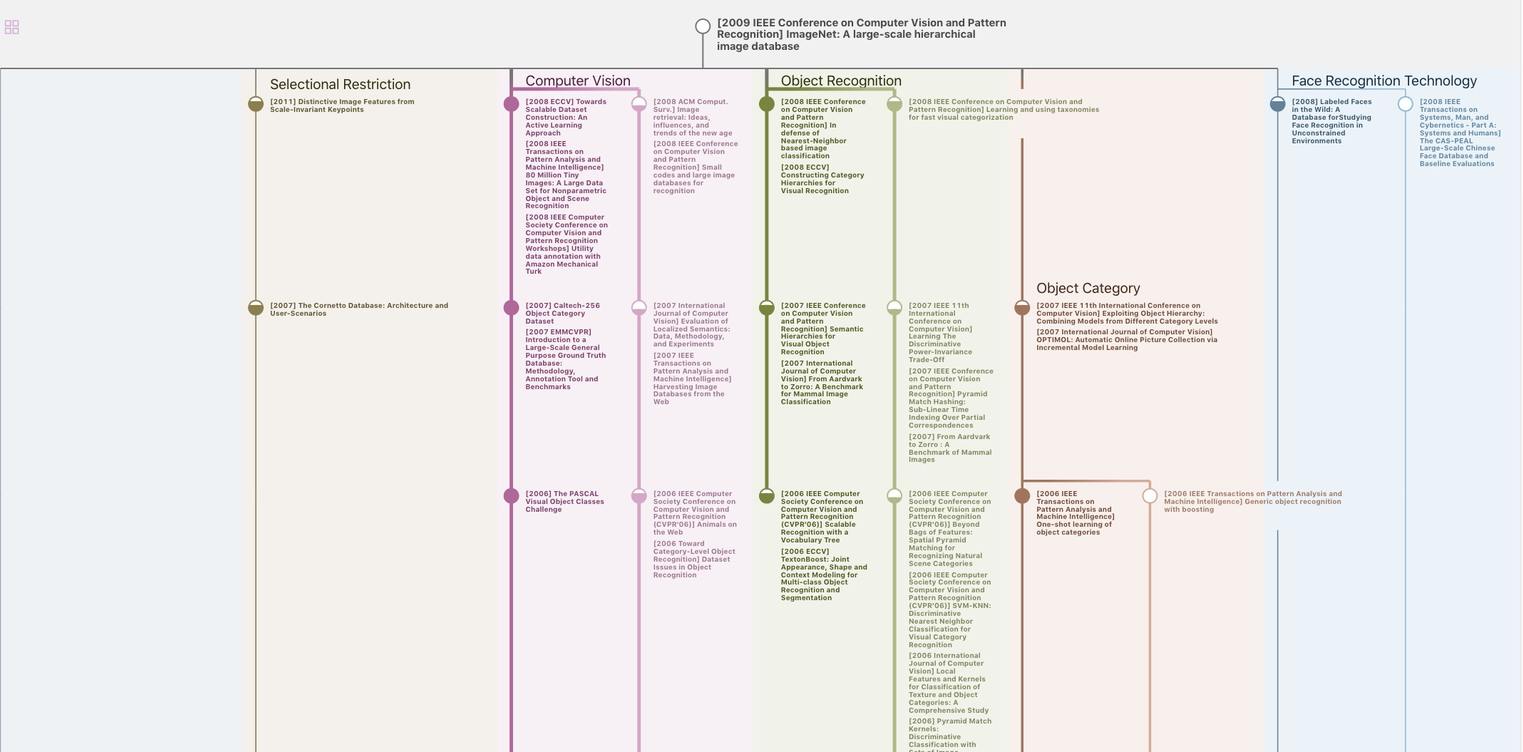
生成溯源树,研究论文发展脉络
Chat Paper
正在生成论文摘要