Proportion of Similar Neighbours and Optimal Weight of Neighbourhood Contribution in Learning Dynamics of Networked MARL
2023 7th Scientific School Dynamics of Complex Networks and their Applications (DCNA)(2023)
Abstract
Structure of interaction between intelligent agents in networked multi-agent reinforcement learning process is one of important components, affecting learning process and convergence. Interactions of agents, having different objective functions may negatively affect each others learning speed. Network topology was shown to affect learning dynamics. In addition, correlations between nodes were shown to affect optimal proportion of information, assimilated from neighbours, and network correlations were shown to change during learning process. In current paper we explore a correlated network of RL agents interaction, built with respect to a graph density and a proportion of similar agents. The effect of these graph parameters on optimal information volume, used from neighbours, was explored for the environment with three types of users and corresponding three types of messages, sent by RL agents. Learning process maximises users satisfaction. Experiments show 1) the more similar neighbours, the less own information agent needs; 2) increase in density (or in the number of neighbours) may be compensated by their opinion weights, affecting agent learning process.
MoreTranslated text
Key words
networked MARL,topology,learning speed,similarity,neighbours
AI Read Science
Must-Reading Tree
Example
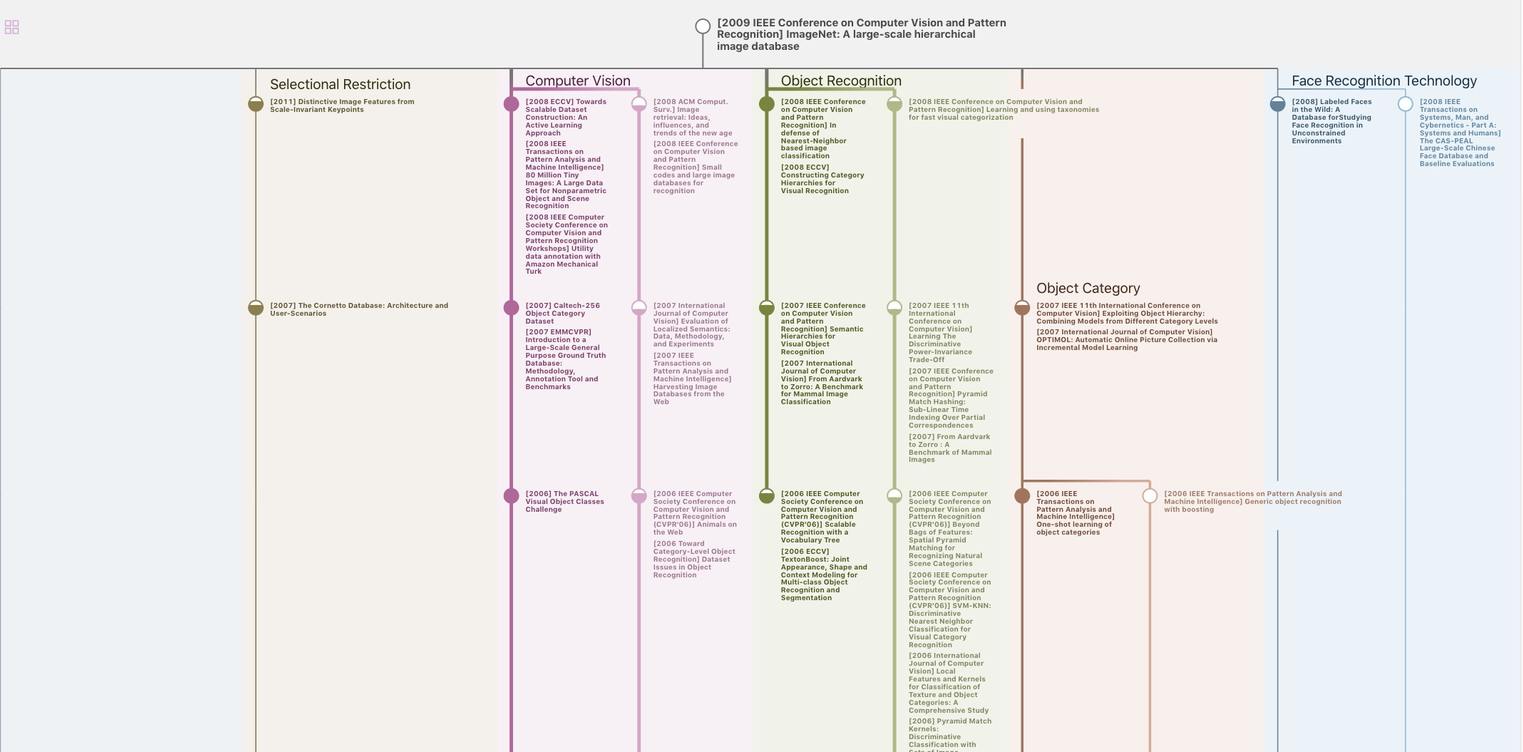
Generate MRT to find the research sequence of this paper
Chat Paper
Summary is being generated by the instructions you defined