Multi-Label Classification of Chest X-ray Abnormalities Using Transfer Learning Techniques
Journal of personalized medicine(2023)
摘要
In recent years, deep neural networks have enabled countless innovations in the field of image classification. Encouraged by success in this field, researchers worldwide have demonstrated how to use Convolutional Neural Network techniques in medical imaging problems. In this article, the results were obtained through the use of the EfficientNet in the task of classifying 14 different diseases based on chest X-ray images coming from the NIH (National Institutes of Health) ChestX-ray14 dataset. The approach addresses dataset imbalances by introducing a custom split to ensure fair representation. Binary cross entropy loss is utilized to handle the multi-label difficulty. The model architecture comprises an EfficientNet backbone for feature extraction, succeeded by sequential layers including GlobalAveragePooling, Dense, and BatchNormalization. The main contribution of this paper is a proposed solution that outperforms previous state-of-the-art deep learning models average area under the receiver operating characteristic curve-AUC-ROC (score: 84.28%). The usage of the transfer-learning technique and traditional deep learning engineering techniques was shown to enable us to obtain such results on consumer-class GPUs (graphics processing units).
更多查看译文
关键词
deep learning, machine learning, radiology, X-ray, diagnostic classification, CNN, chest X-ray
AI 理解论文
溯源树
样例
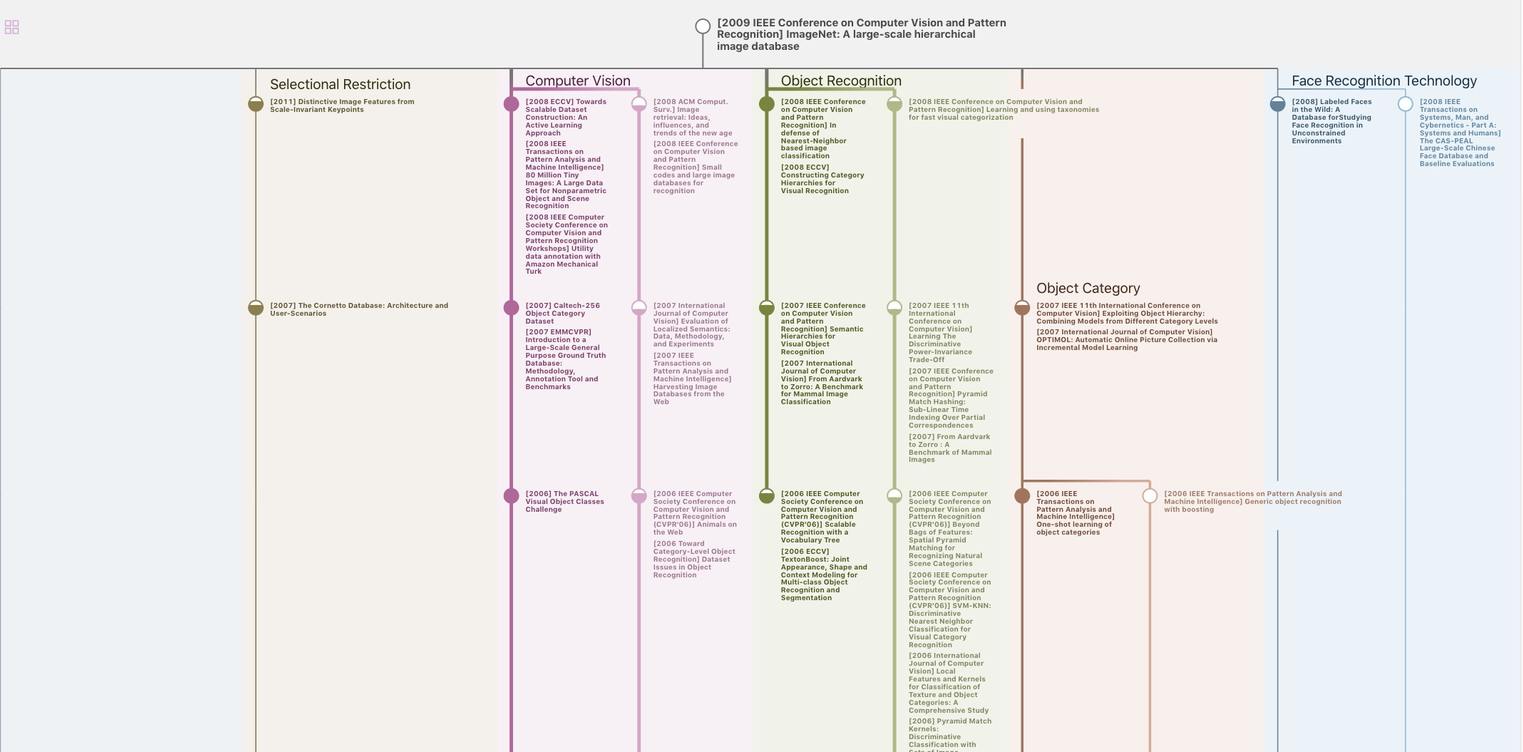
生成溯源树,研究论文发展脉络
Chat Paper
正在生成论文摘要