Spatial Domain Image Fusion with Particle Swarm Optimization and Lightweight AlexNet for Robotic Fish Sensor Fault Diagnosis
Biomimetics (Basel, Switzerland)(2023)
Abstract
Safety and reliability are vital for robotic fish, which can be improved through fault diagnosis. In this study, a method for diagnosing sensor faults is proposed, which involves using Gramian angular field fusion with particle swarm optimization and lightweight AlexNet. Initially, one-dimensional time series sensor signals are converted into two-dimensional images using the Gramian angular field method with sliding window augmentation. Next, weighted fusion methods are employed to combine Gramian angular summation field images and Gramian angular difference field images, allowing for the full utilization of image information. Subsequently, a lightweight AlexNet is developed to extract features and classify fused images for fault diagnosis with fewer parameters and a shorter running time. To improve diagnosis accuracy, the particle swarm optimization algorithm is used to optimize the weighted fusion coefficient. The results indicate that the proposed method achieves a fault diagnosis accuracy of 99.72% when the weighted fusion coefficient is 0.276. These findings demonstrate the effectiveness of the proposed method for diagnosing depth sensor faults in robotic fish.
MoreTranslated text
Key words
spatial domain image fusion,fault diagnosis,particle swarm optimization,lightweight alexnet
AI Read Science
Must-Reading Tree
Example
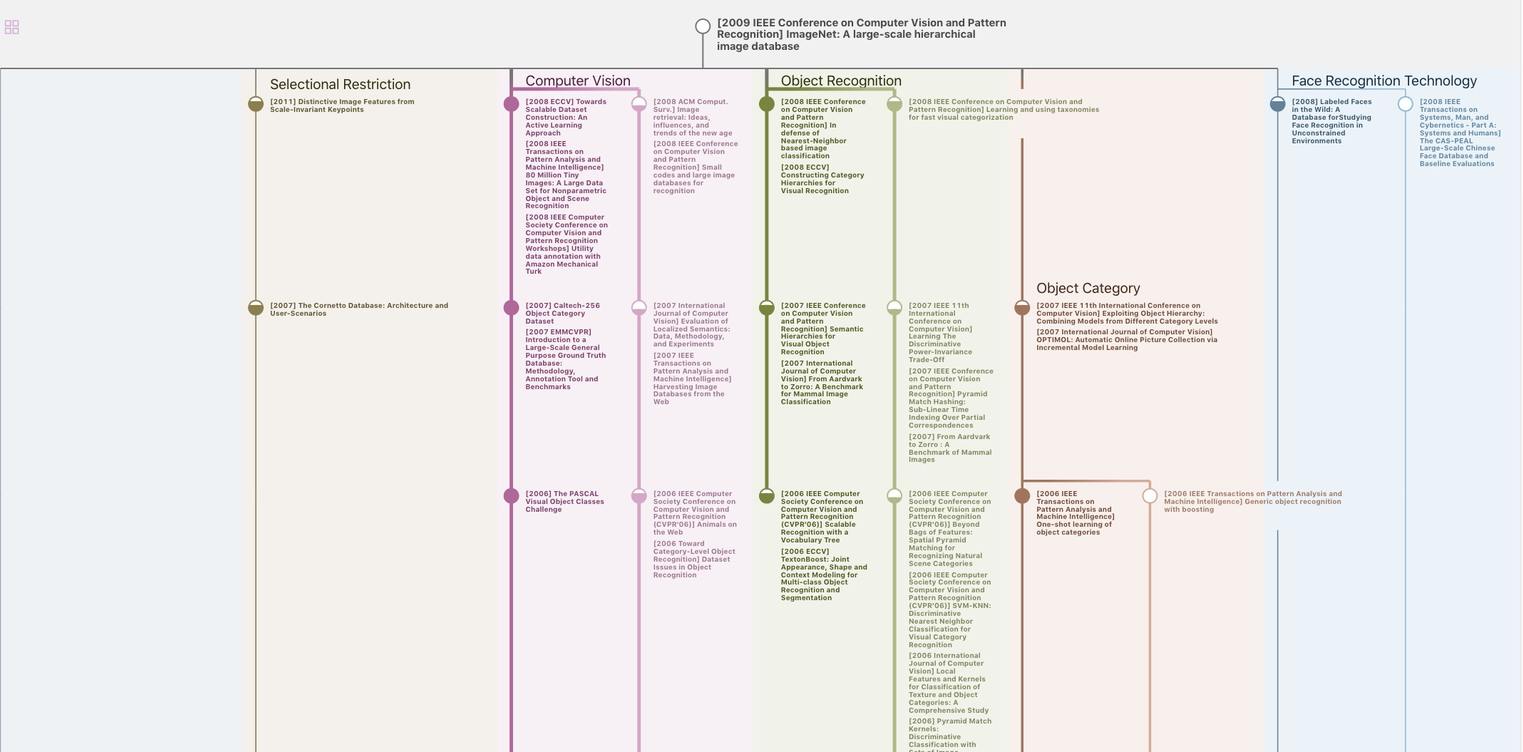
Generate MRT to find the research sequence of this paper
Chat Paper
Summary is being generated by the instructions you defined