Predicting dental caries outcomes in young adults using machine learning approach
BMC Oral Health(2024)
摘要
To predict the dental caries outcomes in young adults from a set of longitudinally-obtained predictor variables and identify the most important predictors using machine learning techniques. This study was conducted using the Iowa Fluoride Study dataset. The predictor variables - sex, mother’s education, family income, composite socio-economic status (SES), caries experience at ages 9, 13, and 17, and the cumulative estimates of risk and protective factors, including fluoride, dietary, and behavioral variables from ages 5–9, 9–13, 13–17, and 17–23 were used to predict the age 23 D2+MFS count. The following machine learning models (LASSO regression, generalized boosting machines (GBM), negative binomial (NegGLM), and extreme gradient boosting models (XGBOOST)) were compared under 5-fold cross validation with nested resampling techniques. The prevalence of cavitated level caries experience at age 23 (mean D2+MFS count) was 4.75. The predictive analysis found LASSO to be the best performing model (compared to GBM, NegGLM, and XGBOOST), with a root mean square error (RMSE) of 0.70, and coefficient of determination (R2) of 0.44. After dichotomization of the predicted and observed values of the LASSO regression, the classification results showed accuracy, precision, recall, and ROC AUC of 83.7
更多查看译文
关键词
Machine learning,Dental,Caries,Longitudinal,Artificial intelliegence,Prediction
AI 理解论文
溯源树
样例
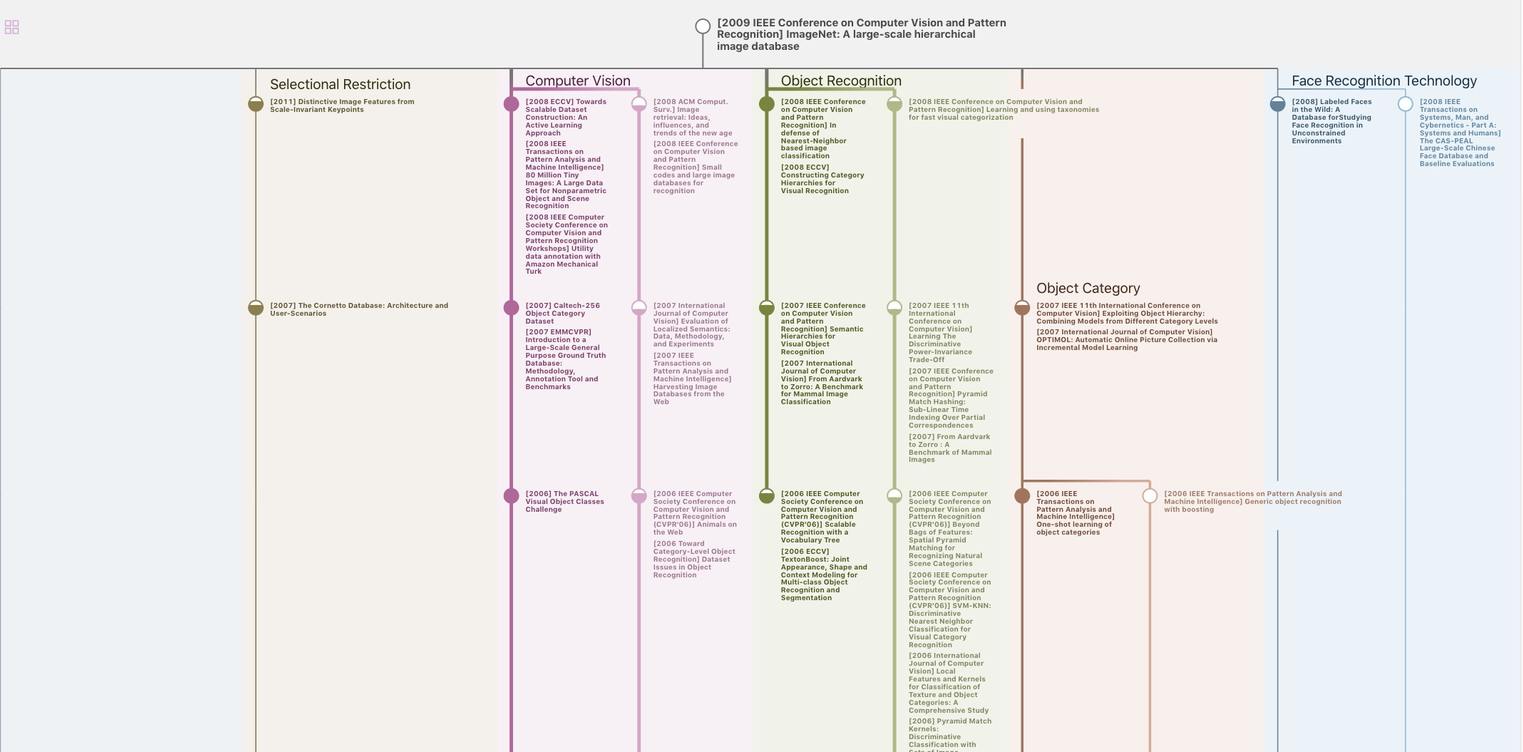
生成溯源树,研究论文发展脉络
Chat Paper
正在生成论文摘要