A machine learning model to predict the need for conversion of operative approach in patients undergoing colectomy for neoplasm
CANCER REPORTS(2024)
摘要
Background: Studies comparing conversion from laparoscopic to open approaches to colectomy have found an association between conversion and morbidity, mortality, and length of stay, suggesting that certain patients may benefit from an open approach "up-front." Aim: The objective of this study was to use machine learning algorithms to develop a model enabling the prediction of which patients are likely to require conversion. Methods and results: We used ACS NSQIP data to identify patients undergoing colectomy (2014-2019). We included patients undergoing elective colectomy for colorectal neoplasm via a minimally invasive approach or a converted approach. The outcome of interest was conversion. Variables were included in the model based on their correlation with conversion by logistic regression (p < .05). Two models were used: weighted logistic regression with regularization, and Random Forest classifier. The data was randomly split into training (70%) and test (30%) cohorts, and prediction performance was calculated. 24 327 cases were included (17 028 training, 7299 test). When applied to the test cohort, the models had an accuracy of 0.675 (range 0.65-0.70) in predicting conversion; c-index ranged from 0.62-0.63. This machine learning model achieved a moderate area under the curve and a high negative predictive value, but a low positive predictive value; therefore, this model can predict (with 95% accuracy) whether a colectomy for neoplasm can be successfully completed using a minimally invasive approach. Conclusion: This model can be used to reassure surgeons of the appropriateness of a minimally invasive approach when planning for an elective colectomy.
更多查看译文
关键词
colectomy,colorectal cancer,conversion,machine learning,surgical approach
AI 理解论文
溯源树
样例
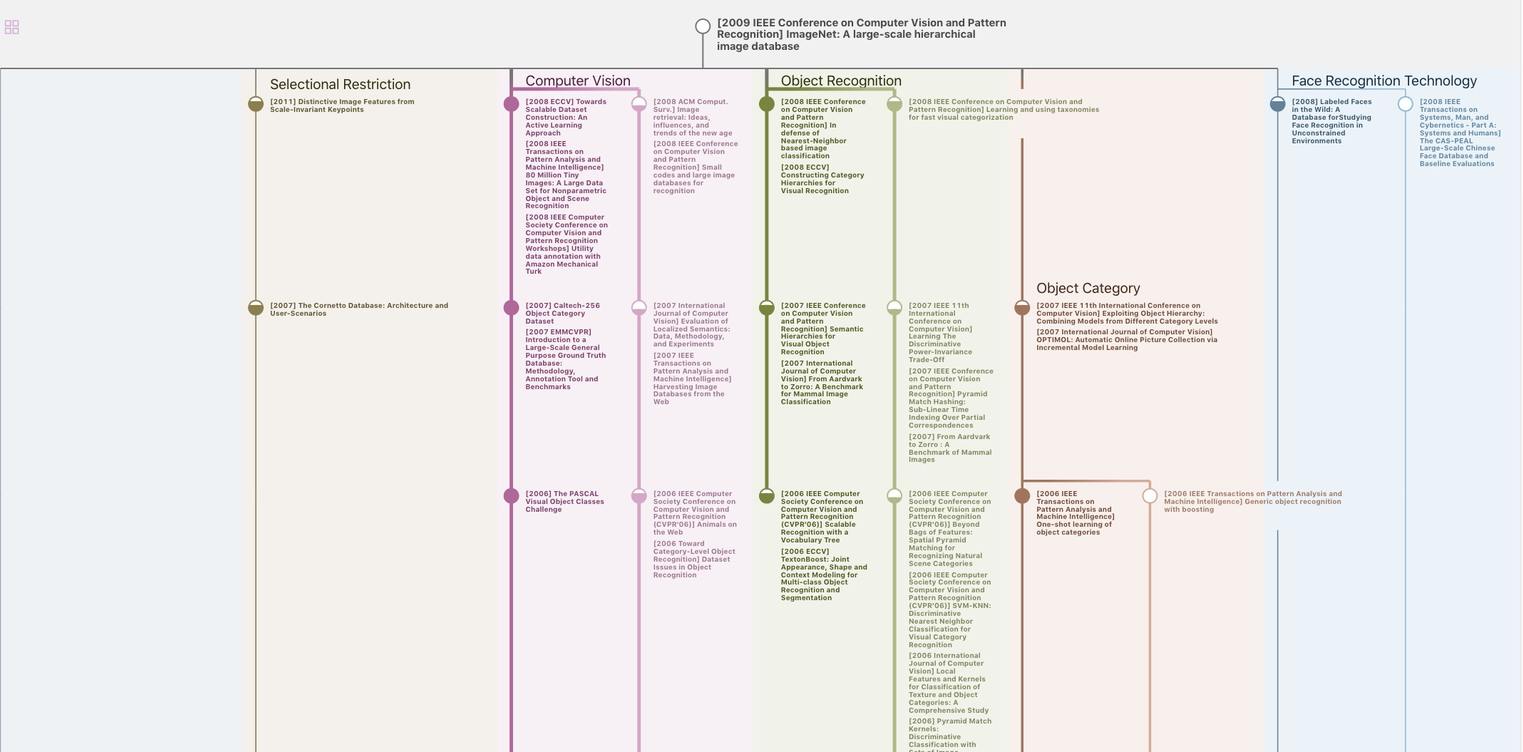
生成溯源树,研究论文发展脉络
Chat Paper
正在生成论文摘要