Encoder-Decoder Contrast for Unsupervised Anomaly Detection in Medical Images
IEEE TRANSACTIONS ON MEDICAL IMAGING(2024)
摘要
Unsupervised anomaly detection (UAD) aims to recognize anomalous images based on the training set that contains only normal images. In medical image analysis, UAD benefits from leveraging the easily obtained normal (healthy) images, avoiding the costly collecting and labeling of anomalous (unhealthy) images. Most advanced UAD methods rely on frozen encoder networks pre-trained using ImageNet for extracting feature representations. However, the features extracted from the frozen encoders that are borrowed from natural image domains coincide little with the features required in the target medical image domain. Moreover, optimizing encoders usually causes pattern collapse in UAD. In this paper, we propose a novel UAD method, namely Encoder-Decoder Contrast (EDC), which optimizes the entire network to reduce biases towards pre-trained image domain and orient the network in the target medical domain. We start from feature reconstruction approach that detects anomalies from reconstruction errors. Essentially, a contrastive learning paradigm is introduced to tackle the problem of pattern collapsing while optimizing the encoder and the reconstruction decoder simultaneously. In addition, to prevent instability and further improve performances, we propose to bring globality into the contrastive objective function. Extensive experiments are conducted across four medical image modalities including optical coherence tomography, color fundus image, brain MRI, and skin lesion image, where our method outperforms all current state-of-the-art UAD methods.
更多查看译文
关键词
Image reconstruction,Feature extraction,Biomedical imaging,Training,Decoding,Anomaly detection,Generative adversarial networks,Medical anomaly detection,unsupervised learning,feature reconstruction,contrastive learning,anomaly localization
AI 理解论文
溯源树
样例
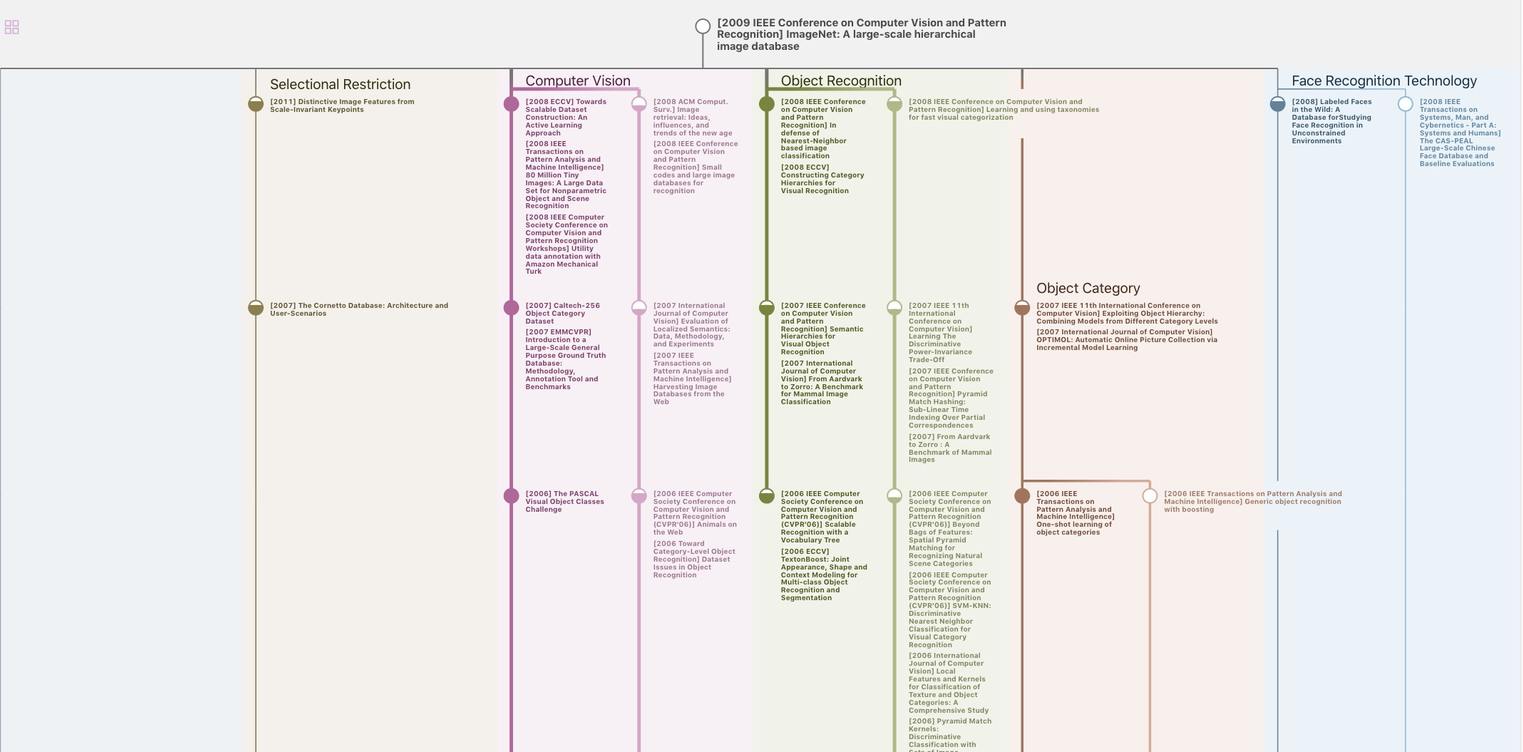
生成溯源树,研究论文发展脉络
Chat Paper
正在生成论文摘要