GenerativeMTD: A deep synthetic data generation framework for small datasets
Knowledge-Based Systems(2023)
摘要
Synthetic data generation for tabular data unlike computer vision, is an emerging challenge. When tabular data needs to be synthesized, it either faces a small dataset problem or violates privacy if the data contains sensitive information. When the data is small, any data-driven modeling leads to biased decision making. On the other hand, deep learning models that use small dataset for training are limited. Tabular data also faces a myriad of challenges, such as mixed data types, fidelity, mode collapse, etc. To eradicate small dataset problems and increase the deep learning capabilities on small data, a new generative method, GenerativeMTD, is proposed in this research. The method generates fake data by using pseudo-real data as input during the training. Pseudo-real data serves the purpose of training the deep learning model with large samples when the real dataset size is small. The pseudo-real data is generated from the real data through k-nearest neighbor mega-trend diffusion. This pseudo-real data is then translated into synthetic data that is similar and realistic to the real data. The method outperforms some of the state-of-the-art methodologies that exist in tabular data generation. The proposed method also generates quality synthetic data for the benchmark datasets in terms of pairwise correlation differences. In addition, the method surpasses the benchmark models in terms of the distance-based privacy metrics: distance to the closest record and nearest neighbor distance ratio.
更多查看译文
关键词
Small dataset,Synthetic data generation,Deep learning,Privacy-preserving
AI 理解论文
溯源树
样例
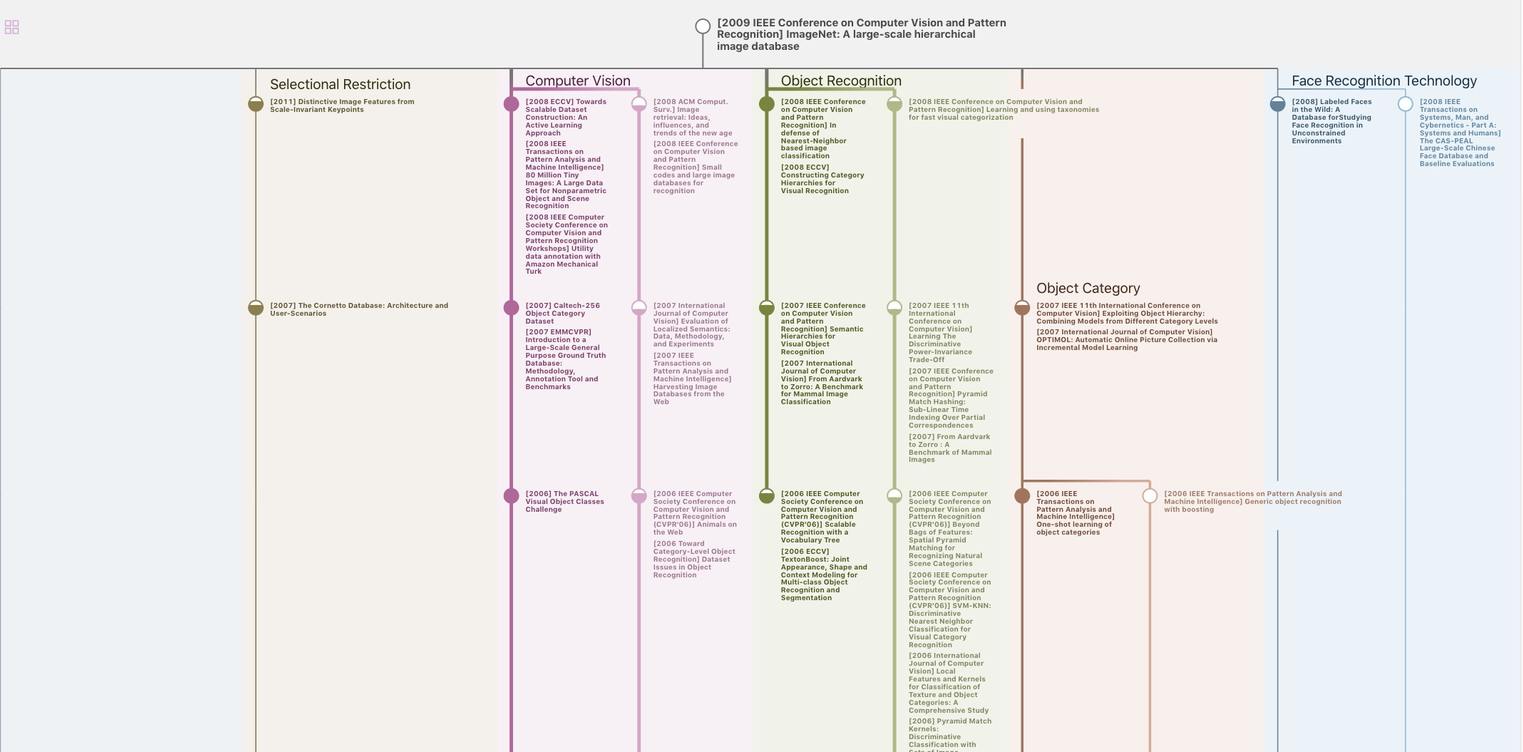
生成溯源树,研究论文发展脉络
Chat Paper
正在生成论文摘要