CtFPPN: A coarse-to-fine pattern parser for dealing with distribution imbalance of pixels
KNOWLEDGE-BASED SYSTEMS(2023)
摘要
Unbalanced pixel distribution has always plagued pattern parsing tasks. The consequence of this is that the saliency of tiny semantic components is overshadowed by large components, resulting in insufficient graph attentions from the model. Recent attempts typically crop tiny patches and predict masks for each semantic part. However, those strategies consist of separate stages and lack interactions, thus cannot be jointly optimized for collaborative perception. To compensate for this flaw, a coarse-to-fine pattern parsing network (CtFPPN) is proposed based on capsule network (CapsNet). Its coarse-grained parser submodel predicts and binaries coarse scaled parsing masks for large components. Given the coarse contexts as references, fine-grained parser submodel conducts fine-scaled parsing for tiny components. To connect two parsing phases, the discretization attention fragmentation mechanism (DAFM) and multi-head attention expectation-maximum routing agreement (MhAEMRA) are customized. DAFM balances the model's attention to large and small semantic components. MhAEMRA receives attention tendencies from DAFM and updates learnable parameters. With DAFM and MhAEMRA, CtFPPN gradually deconstruct patterns by clustering highly associated secondary entities in a bottom-up "part backtracking" manner. Quantitative and ablation experiments of face and human parsing demonstrate the superiority of CtFPPN over the state-of-the-arts, especially for the definition of fine-grained semantic boundaries of components.
更多查看译文
关键词
Pattern parsing,Unbalanced pixel distribution,Multi-head attention mechanism,Capsule network,Expectation-maximum routing agreement
AI 理解论文
溯源树
样例
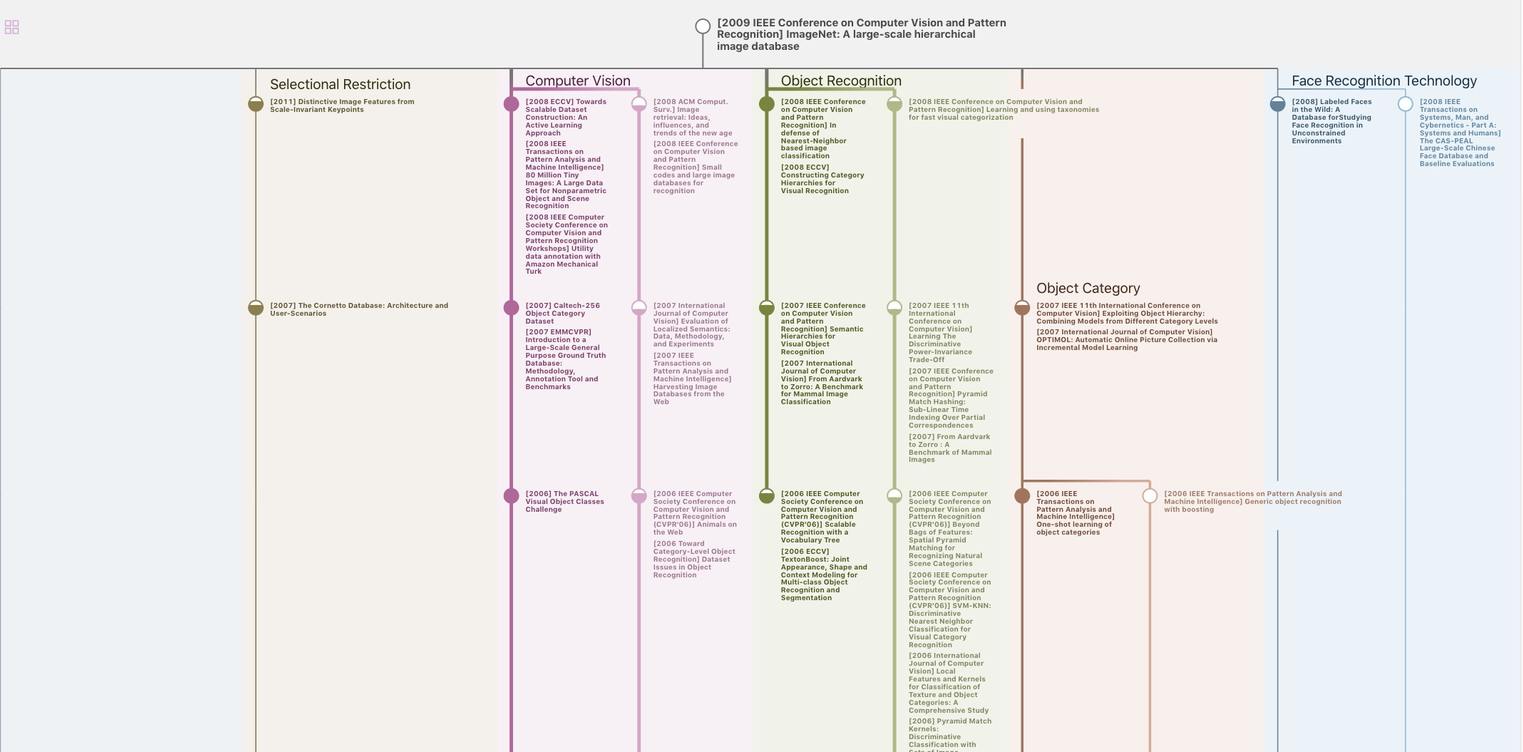
生成溯源树,研究论文发展脉络
Chat Paper
正在生成论文摘要