Artificial intelligence for enhanced flotation monitoring in the mining industry: A ConvLSTM-based approach
COMPUTERS & CHEMICAL ENGINEERING(2024)
Abstract
In the mining industry, accurate monitoring of the elemental composition in the flotation froth is crucial for efficient minerals separation. The hybrid deep learning algorithms offer powerful computational intelligence for real-time monitoring of froth quality in flotation processes. This soft sensor tool can provide valuable information for process control, including predictions of the elemental chemical composition. In this study, we propose a novel approach based on a Convolutional Long Short-Term Memory (ConvLSTM) neural network for real-time monitoring of chemical composition grades in flotation froth. The proposed model effectively extracts spatial and temporal patterns from video data, providing a better understanding of the dynamic behavior of the froth surface in flotation processes. Our proposed approach includes a deployment architecture that enables real-time monitoring of elemental concentrate grades, allowing for the adjustment of flotation parameters for accurate process efficiency.The results of our study show that The proposed approach is accurate, with a mean absolute error (MAE) of 4.52 in the cleaners of the zinc differential flotation circuit. The use of the ConvLSTM neural network provides an accurate and reliable model for monitoring the flotation froth quality. The proposed approach has the potential to improve the operational performance and efficiency of complex polymetallic flotation circuits in mineral processing. Additionally, we propose a deployment architecture in the industrial scale of a differential flotation circuit. This architecture offers advantages such as portability, scalability, consistency, and isolation for efficient use for the flotation monitoring. This study demonstrates the potential of using a deployed deep learning model to provide a practical and efficient solution for real-time monitoring of flotation froth quality in the mining industry. This approach can be used to optimize the flotation process and increase productivity, potentially leading to significant flotation performances improvement and cost saving for mining companies.
MoreTranslated text
Key words
Deep learning,Flotation froth,Mining industry 4.0,Real time,Monitoring,Chemical composition
AI Read Science
Must-Reading Tree
Example
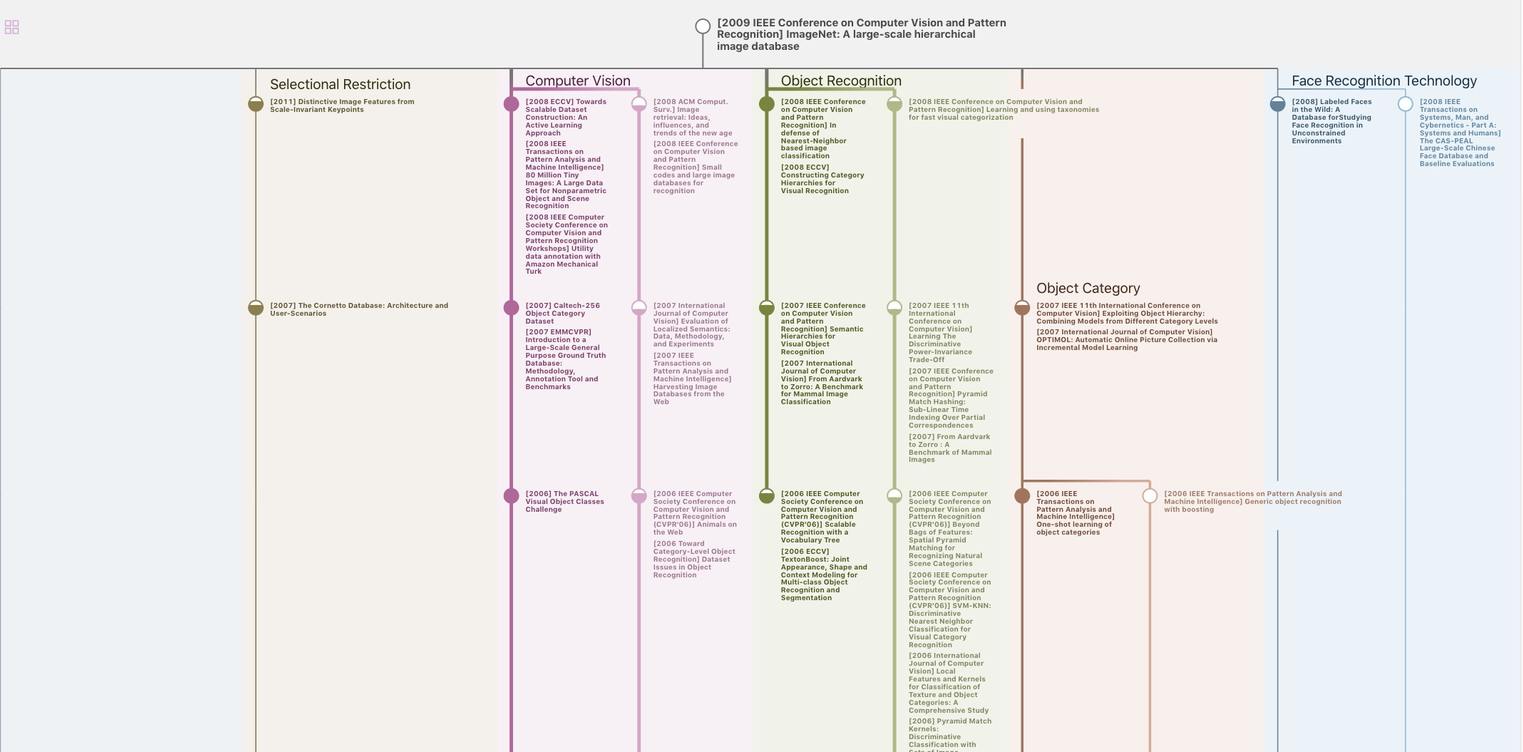
Generate MRT to find the research sequence of this paper
Chat Paper
Summary is being generated by the instructions you defined