Optimization of large portfolio allocation for new-energy stocks: Evidence from China
Energy(2023)
摘要
Investment in the fast-growing new-energy of China has gradually attracted great attention. At present, more than 100 new-energy stocks are listed on China’s stock markets. How to effectively manage the investment risk of these stocks and achieve better returns is of great interest to investors and policymakers. In finance, mean–variance portfolio (MVP) is often applied to solve portfolio allocation problems. However, when investing in numerous stocks, the classical MVP is no longer applicable. For large portfolio allocation, this study constructs a comprehensive MVP model, called an unconstrained regression model with latent factors (URELAF). It applies a factor structure with latent factors to modify the covariance matrix estimation in the classical MVP, using an unconstrained penalized regression to estimate the allocation. In addition, to more realistically simulate the investment of stock markets, transaction costs are considered. The empirical results show that URELAF model can control the risk with Sharpe ratio reaching 6 times as many as MVP model, and even improve about 26% compared with the sub-optimal portfolio model. URELAF also outperforms other portfolios with transaction costs. Finally, hypothesis tests further verify that URELAF can significantly improve portfolio performance compared with other portfolio methods for China’s new-energy market.
更多查看译文
关键词
large portfolio allocation,optimization,new-energy
AI 理解论文
溯源树
样例
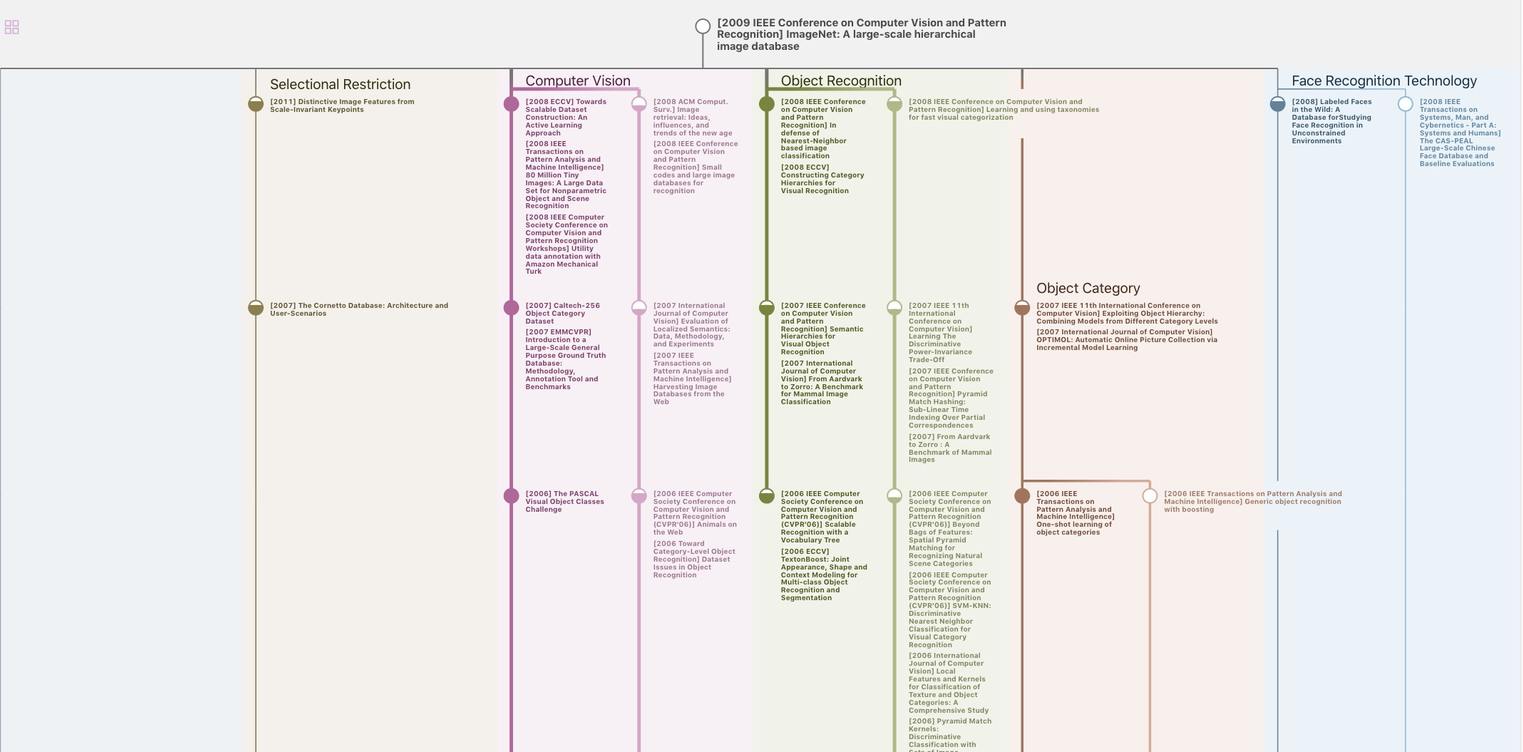
生成溯源树,研究论文发展脉络
Chat Paper
正在生成论文摘要