Nonlinear directed acyclic graph estimation based on the kernel partial correlation coefficient
INFORMATION SCIENCES(2024)
摘要
Directed acyclic graphs (DAGs) are powerful tools for detecting causality among variables and thus, they have attracted increasing interest in recent years. In most previous studies, DAGs were estimated under the assumption of linearity between variables; however, this assumption is not usually true in real-world applications. Therefore, developing DAG estimation methods for nonlinear scenarios is of great interest. In this work, we propose a DAG estimation method based on the kernel partial correlation (KPC) coefficient to identify nonlinear interactions among variables. The method consists of three steps: neighborhood selection, skeleton estimation and direction detection. While all the steps are grounded on the KPC coefficient, we develop a threshold-based criterion to address the falsely discovered edges in the skeleton estimation step and utilize the asymmetric property of the KPC coefficient in the direction detection step. Numerical simulations demonstrate the advantages of the proposed method over various competing methods. Finally, we apply the proposed method to construct networks in three real -world structural equation modeling examples in the social sciences and on one DNA binding site dataset to demonstrate its effectiveness.
更多查看译文
关键词
Directed acyclic graph,Nonlinearity,Reproducing kernel Hilbert space,Kernel partial correlation coefficient,Causality detection
AI 理解论文
溯源树
样例
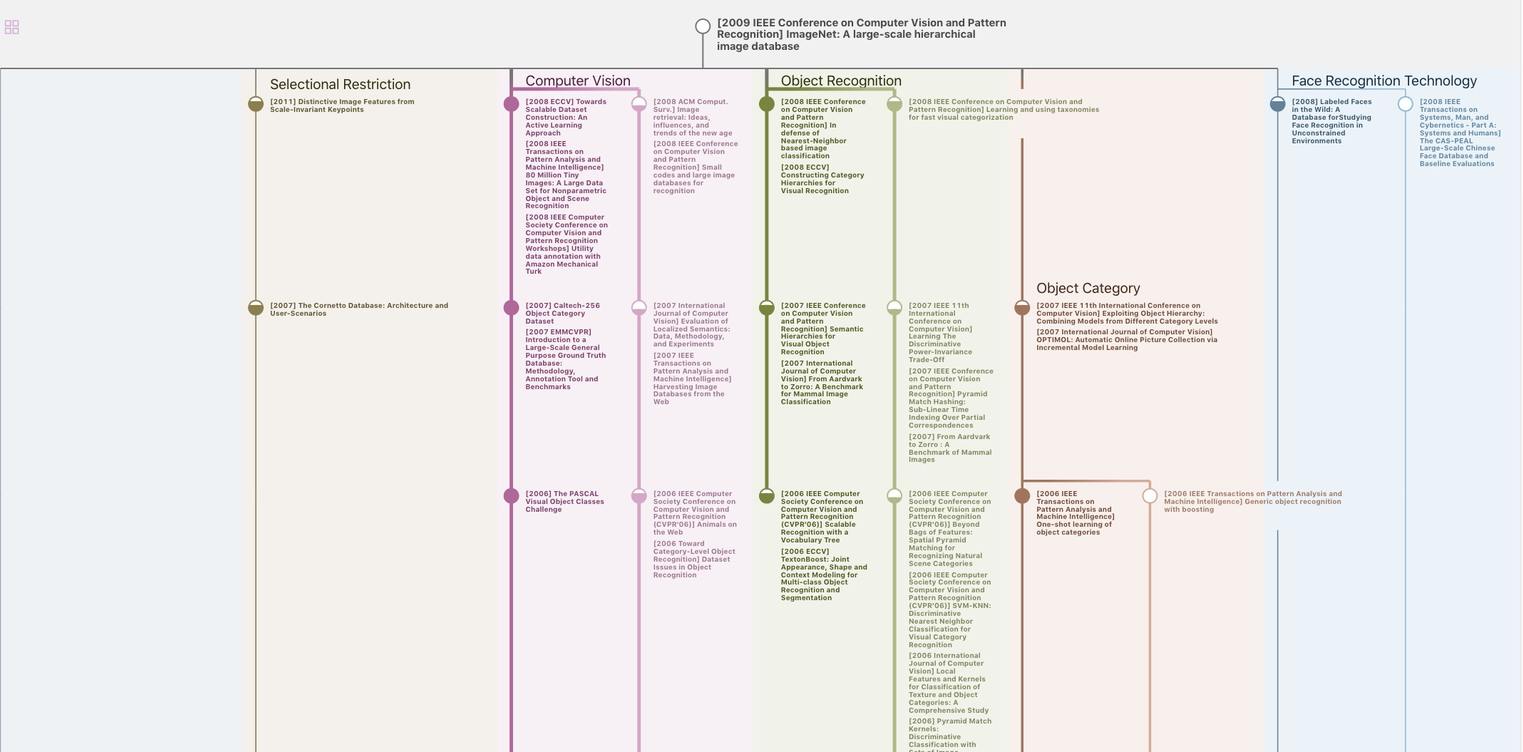
生成溯源树,研究论文发展脉络
Chat Paper
正在生成论文摘要