Adaptive online continual multi-view learning
INFORMATION FUSION(2024)
摘要
Deep neural networks (DNNs) have gained great success in information fusion. However, recent studies report that DNNs are suffering from catastrophic forgetting, i.e., DNNs would forget the knowledge learned from previous tasks when training on the current task. To address this issue, continual learning is proposed to enhance long-term memories for DNNs. Since continual learning is very challenging, existing work simplifies the setting to simulate the sequentially online multi-task learning paradigm. Specifically, existing works commonly split one dataset into multiple disjoint categories to get multiple tasks that follow the same marginal distribution. We argue that this setting is too simple to approximate the real-world applications. In real-world scenarios, the data distributions of sequentially arrived tasks would change significantly from time to time, e.g., the lighting from day to night, and the background from spring to winter. Thus, the real-world applications are in a multi-view manner, yet existing methods ignore this challenge. To tame this, we propose Adaptive Online Continual Multi-view Learning (AOCML) to align distributions and reduce catastrophic forgetting as new tasks arrive. AOCML integrates experience replay and adversarial learning in an end-to-end framework, which stores samples in a memory buffer to replay previous tasks, while leveraging a discriminator to adaptively align distributions across views on-the-fly. In addition to common replay buffer, we also incorporate a soft label-based replay and an entropy-based reweighting to further prevent forgetting. Extensive experiments on four datasets verify that our method is able to significantly outperform previous CL methods and our method pushes CL one step forward towards practical multi-view orientation.
更多查看译文
关键词
Domain adaptation,Continual learning,Multi-view learning,Lifelong learning
AI 理解论文
溯源树
样例
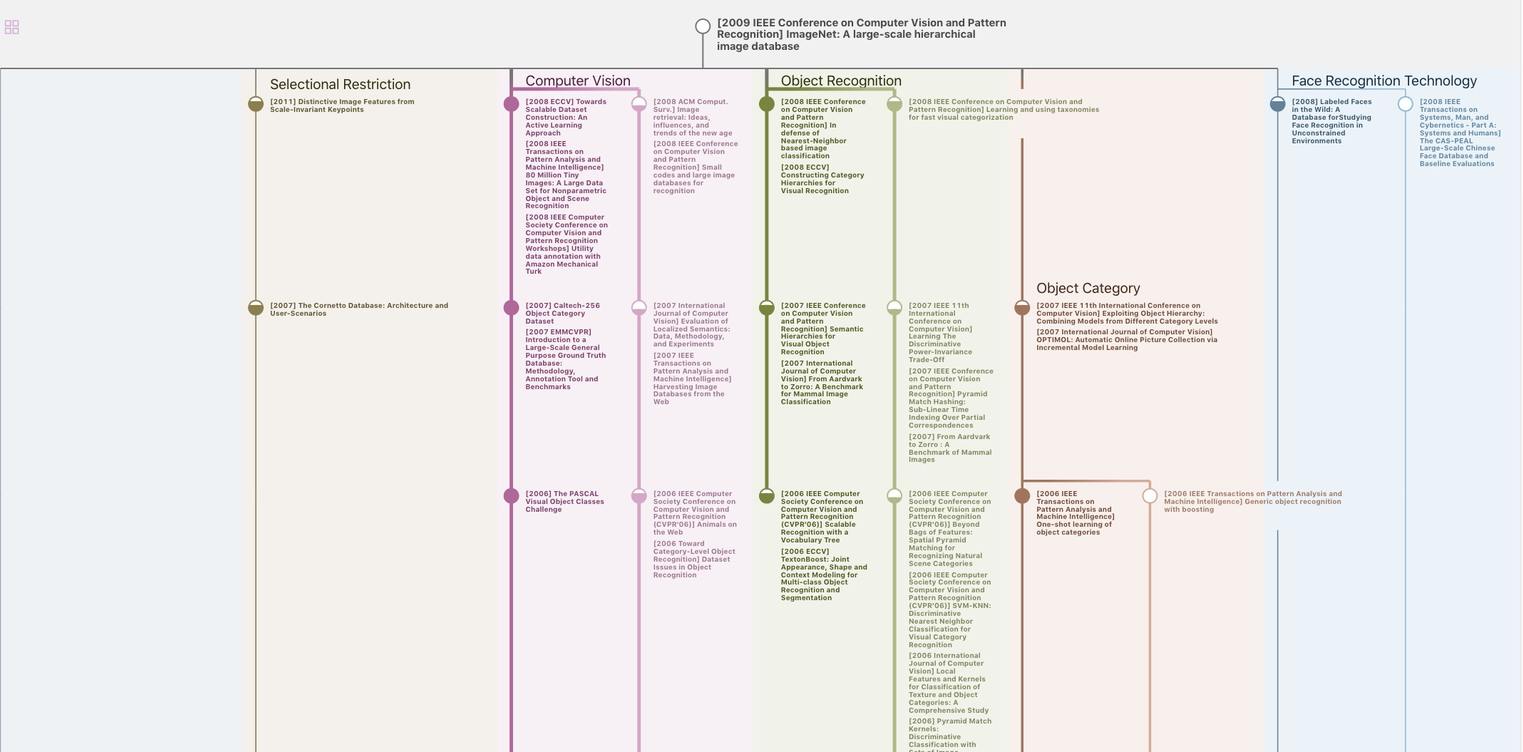
生成溯源树,研究论文发展脉络
Chat Paper
正在生成论文摘要