Machine Learning for Simulation of Urban Heat Island Dynamics Based on Large-Scale Meteorological Conditions
Climate(2023)
摘要
This study considers the problem of approximating the temporal dynamics of the urban-rural temperature difference (Delta T) in Moscow megacity using machine learning (ML) models and predictors characterizing large-scale weather conditions. We compare several ML models, including random forests, gradient boosting, support vectors, and multi-layer perceptrons. These models, trained on a 21-year (2001-2021) dataset, successfully capture the diurnal, synoptic-scale, and seasonal variations of the observed Delta T based on predictors derived from rural weather observations or ERA5 reanalysis. Evaluation scores are further improved when using both sources of predictors simultaneously and involving additional features characterizing their temporal dynamics (tendencies and moving averages). Boosting models and support vectors demonstrate the best quality, with RMSE of 0.7 K and R-2 > 0.8 on average over 21 years. For three selected summer and winter months, the best ML models forced only by reanalysis outperform the comprehensive hydrodynamic mesoscale model COSMO, supplied by an urban canopy scheme with detailed city-descriptive parameters and forced by the same reanalysis. However, for a longer period (1977-2023), the ML models are not able to fully reproduce the observed trend of Delta T increase, confirming that this trend is largely (by 60-70%) driven by megacity growth. Feature importance assessment indicates the atmospheric boundary layer height as the most important control factor for the Delta T and highlights the relevance of temperature tendencies as additional predictors.
更多查看译文
关键词
statistical modeling,regression,urban climate,urban heat island,climate change,urbanization,Moscow
AI 理解论文
溯源树
样例
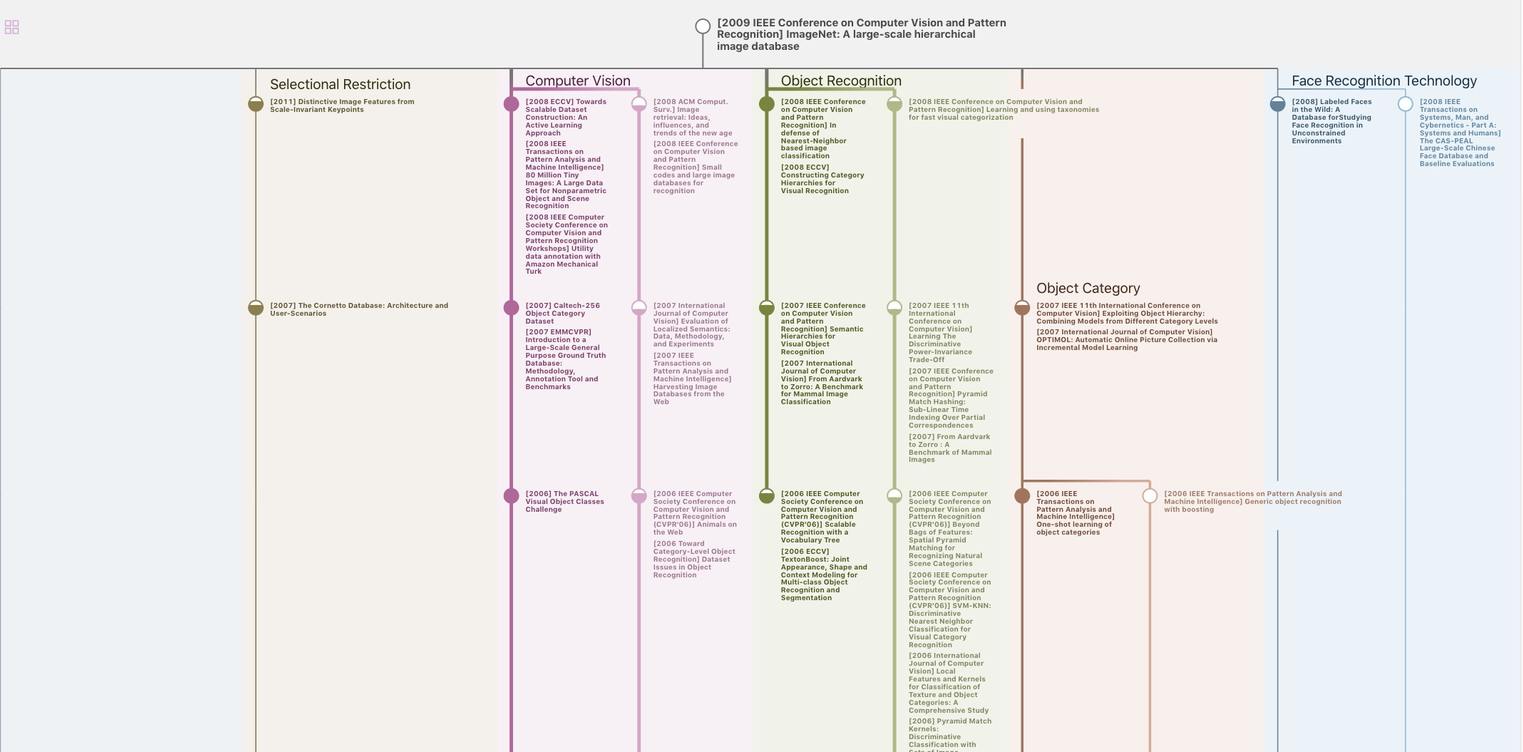
生成溯源树,研究论文发展脉络
Chat Paper
正在生成论文摘要