A Rnyi-Type Limit Theorem on Random Sums and the Accuracy of Likelihood-Based Classification of Random Sequences with Application to Genomics
Mathematics(2023)
摘要
We study classification of random sequences of characters selected from a given alphabet into two classes characterized by distinct character selection probabilities and length distributions. The classification is based on the sign of the log-likelihood score (LLS) consisting of a random sum and a random term depending on the length distributions for the two classes. For long sequences selected from a large alphabet, computing misclassification error rates is not feasible either theoretically or computationally. To mitigate this problem, we computed limiting distributions for two versions of the normalized LLS applicable to long sequences whose class-specific length follows a translated negative binomial distribution (TNBD). The two limiting distributions turned out to be plain or transformed Erlang distributions. This allowed us to establish the asymptotic accuracy of the likelihood-based classification of random sequences with TNBD length distributions. Our limit theorem generalizes a classic theorem on geometric random sums due to Renyi and is closely related to the published results of V. Korolev and coworkers on negative binomial random sums. As an illustration, we applied our limit theorem to the classification of DNA sequences contained in the genome of the bacterium Bacillus subtilis into two classes: protein-coding genes and standard noncoding open reading frames. We found that TNBDs provide an excellent fit to the length distributions for both classes and that the limiting distributions capture essential features of the normalized empirical LLS fairly well.
更多查看译文
关键词
Rényi theorem,sequence classification,classification accuracy,random sum,translated negative binomial distribution,Kullback–Leibler distance
AI 理解论文
溯源树
样例
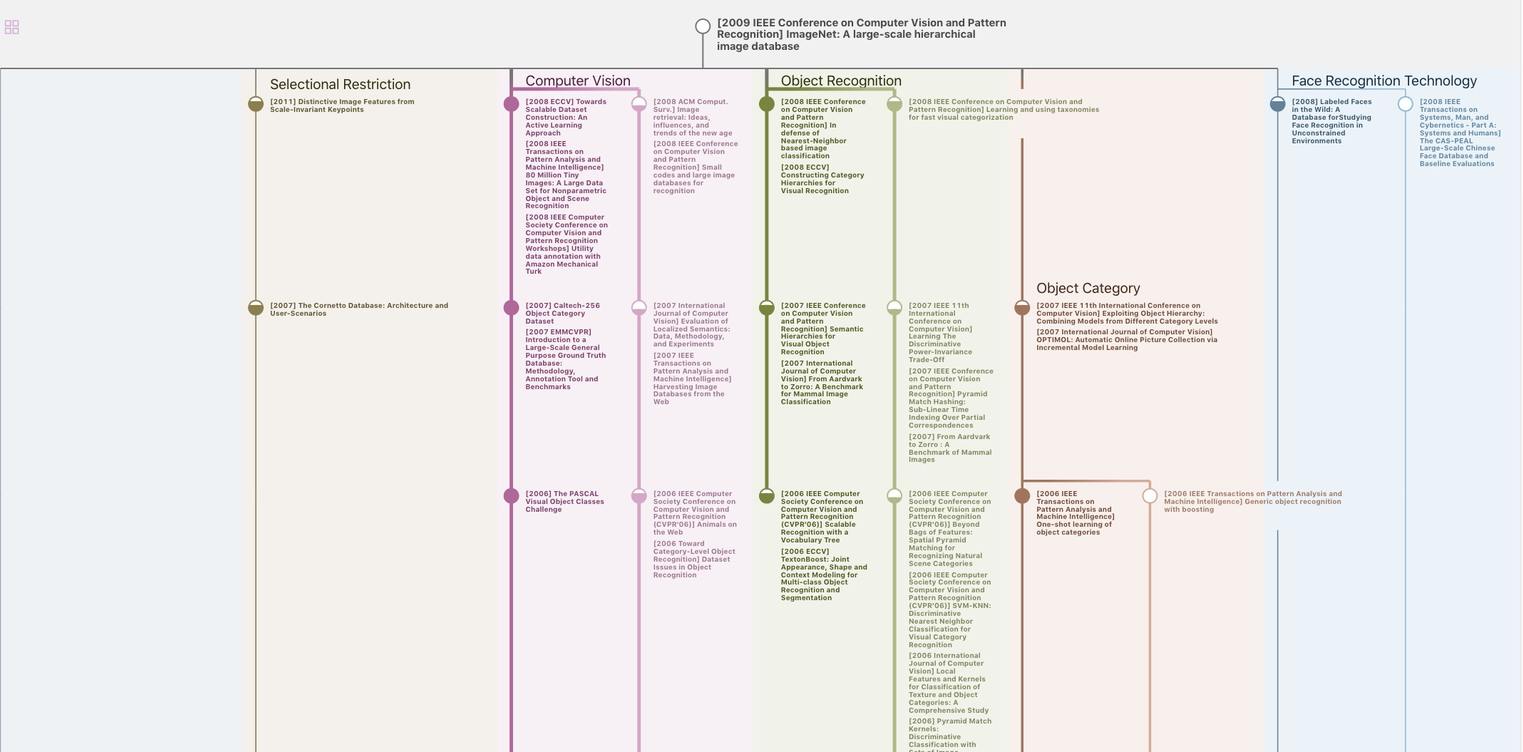
生成溯源树,研究论文发展脉络
Chat Paper
正在生成论文摘要