Structure-Aware Low-Rank Adaptation for Parameter-Efficient Fine-Tuning
Mathematics(2023)
Abstract
With the growing scale of pre-trained language models (PLMs), full parameter fine-tuning becomes prohibitively expensive and practically infeasible. Therefore, parameter-efficient adaptation techniques for PLMs have been proposed to learn through incremental updates of pre-trained weights, such as in low-rank adaptation (LoRA). However, LoRA relies on heuristics to select the modules and layers to which it is applied, and assigns them the same rank. As a consequence, any fine-tuning that ignores the structural information between modules and layers is suboptimal. In this work, we propose structure-aware low-rank adaptation (SaLoRA), which adaptively learns the intrinsic rank of each incremental matrix by removing rank-0 components during training. We conduct comprehensive experiments using pre-trained models of different scales in both task-oriented (GLUE) and task-agnostic (Yelp and GYAFC) settings. The experimental results show that SaLoRA effectively captures the structure-aware intrinsic rank. Moreover, our method consistently outperforms LoRA without significantly compromising training efficiency.
MoreTranslated text
Key words
adaptation,structure-aware,low-rank,parameter-efficient,fine-tuning
AI Read Science
Must-Reading Tree
Example
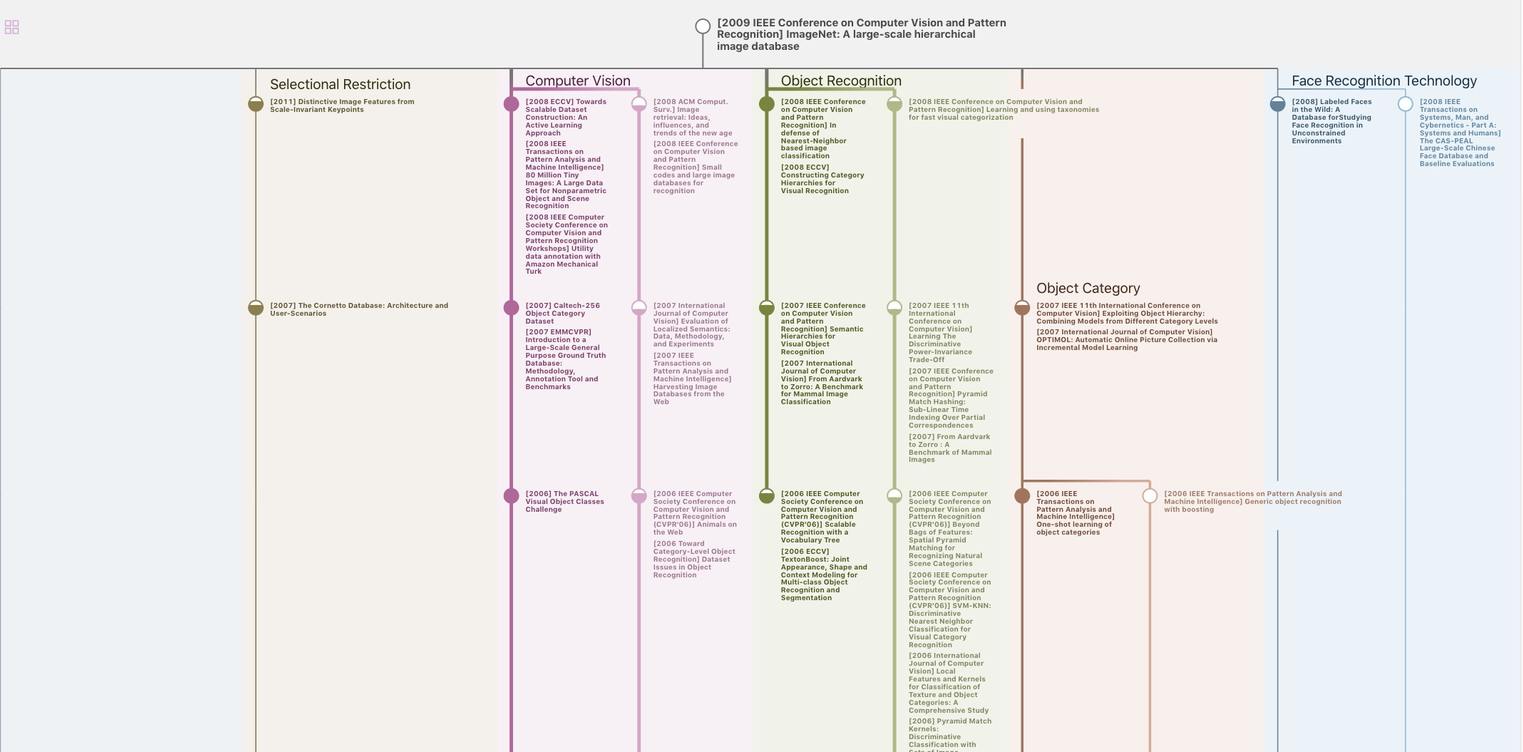
Generate MRT to find the research sequence of this paper
Chat Paper
Summary is being generated by the instructions you defined