Mapping Soil Organic Matter Using Different Modeling Techniques in the Dryland Agroecosystem of Huang-Huai-Hai Plain, Eastern China
Remote Sensing(2023)
摘要
Accurately mapping the spatial distribution and variation of soil organic matter (SOM) is of great significance for guiding regional soil management. However, the applicability and prediction performance of machine learning techniques in dryland agroecosystems still needs to be further studied. In this study, we collected a total of 733 topsoil samples from the farmland in Xiao County, Anhui Province, which is a typical dryland agroecosystem in the Huang-Huai-Hai Plain. Then, the environmental covariates were selected, and the ordinary kriging (OK), multiple linear stepwise regression (MLR), regression kriging (RK), radial basis function neural network (RBFNN), and random forest (RF) models were conducted to map the SOM content, and the optimal model was ascertained. The results demonstrated that the alkali-hydrolyzable nitrogen (26.11%), available potassium (17.73%), mean annual precipitation (13.26%), and pH (11.80%) were the main controlling factors affecting the spatial distribution of SOM in the study area. Meanwhile, the introduction of environmental covariates can effectively improve the SOM prediction accuracy, and the RF model (R-2 = 0.48, MAE = 2.38 g kg(-1), MRE = 12.99%, RMSE = 3.14 g kg(-1)) has a better performance than the RFBNN, MLR, RK, and OK methods. Although there are local differences in the spatial distribution of SOM predicted by the five methods, the overall spatial distribution of SOM was characterized by the low concentration area (13.44-20.00 g kg(-1)) distributed in the central and northwest of study area, and the high concentration area (24.00-28.95 g kg(-1)) distributed in the southeast. Overall, our study demonstrated that machine learning-based models could accurately predict the SOM content in dryland agroecosystem, and the produced maps function as baseline maps for sustainable agricultural management.
更多查看译文
关键词
soil organic matter, digital soil mapping, random forest, main control factors, fluvisols
AI 理解论文
溯源树
样例
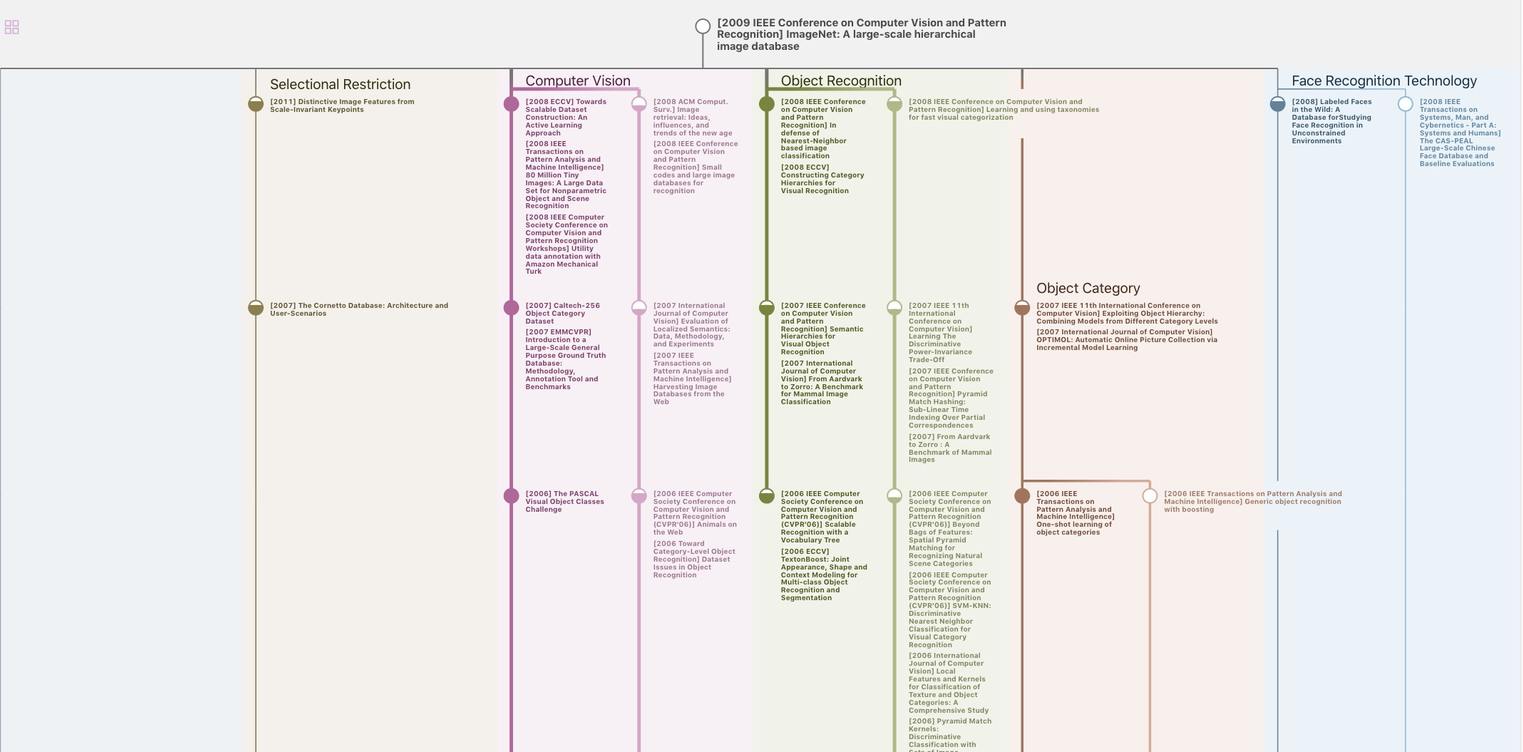
生成溯源树,研究论文发展脉络
Chat Paper
正在生成论文摘要