A Comparison of Monte Carlo-Based and PINN Parameter Estimation Methods for Malware Identification in IoT Networks
Technologies(2023)
摘要
Malware propagation is a growing concern due to its potential impact on the security and integrity of connected devices in Internet of Things (IoT) network environments. This study investigates parameter estimation for Susceptible-Infectious-Recovered (SIR) and Susceptible-Infectious-Recovered-Susceptible (SIRS) models modeling malware propagation in an IoT network. Synthetic data of malware propagation in the IoT network is generated and a comprehensive comparison is made between two approaches: algorithms based on Monte Carlo methods and Physics-Informed Neural Networks (PINNs). The results show that, based on the infection curve measured in the IoT network, both methods are able to provide accurate estimates of the parameters of the malware propagation model. Furthermore, the results show that the choice of the appropriate method depends on the dynamics of the spreading malware and computational constraints. This work highlights the importance of considering both classical and AI-based approaches and provides a basis for future research on parameter estimation in epidemiological models applied to malware propagation in IoT networks.
更多查看译文
关键词
PINN,Monte Carlo,parameter estimation,malware propagation,IoT networks
AI 理解论文
溯源树
样例
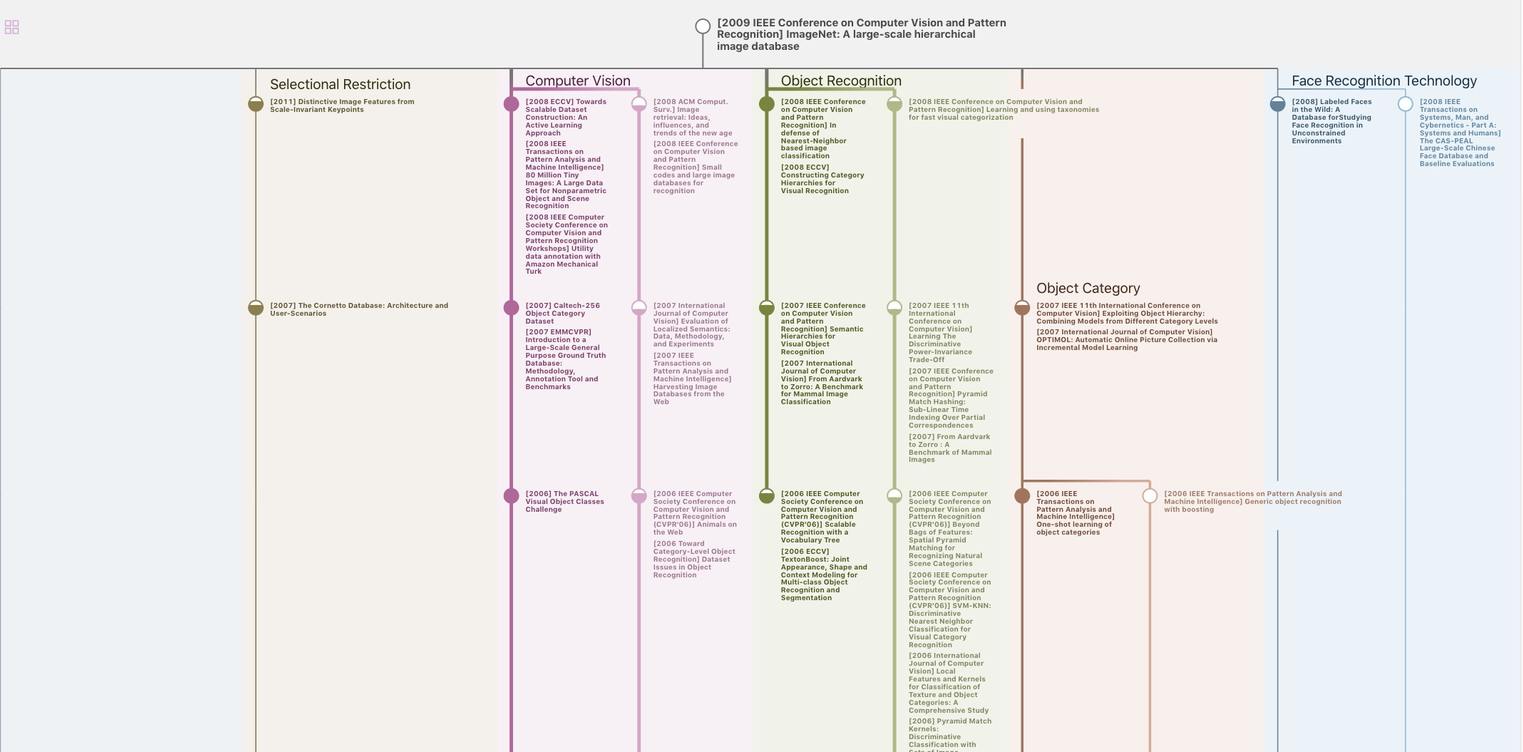
生成溯源树,研究论文发展脉络
Chat Paper
正在生成论文摘要