Angle-Equivariant Convolutional Neural Networks for Interference Mitigation in Automotive Radar
2023 20th European Radar Conference (EuRAD)(2023)
摘要
In automotive applications, frequency modulated continuous wave (FMCW) radar
is an established technology to determine the distance, velocity and angle of
objects in the vicinity of the vehicle. The quality of predictions might be
seriously impaired if mutual interference between radar sensors occurs.
Previous work processes data from the entire receiver array in parallel to
increase interference mitigation quality using neural networks (NNs). However,
these architectures do not generalize well across different angles of arrival
(AoAs) of interferences and objects. In this paper we introduce fully
convolutional neural network (CNN) with rank-three convolutions which is able
to transfer learned patterns between different AoAs. Our proposed architecture
outperforms previous work while having higher robustness and a lower number of
trainable parameters. We evaluate our network on a diverse data set and
demonstrate its angle equivariance.
更多查看译文
关键词
FMCW radar,convolutional neural networks,interference mitigation,angle-equivariance,deep learning,complex-valued processing
AI 理解论文
溯源树
样例
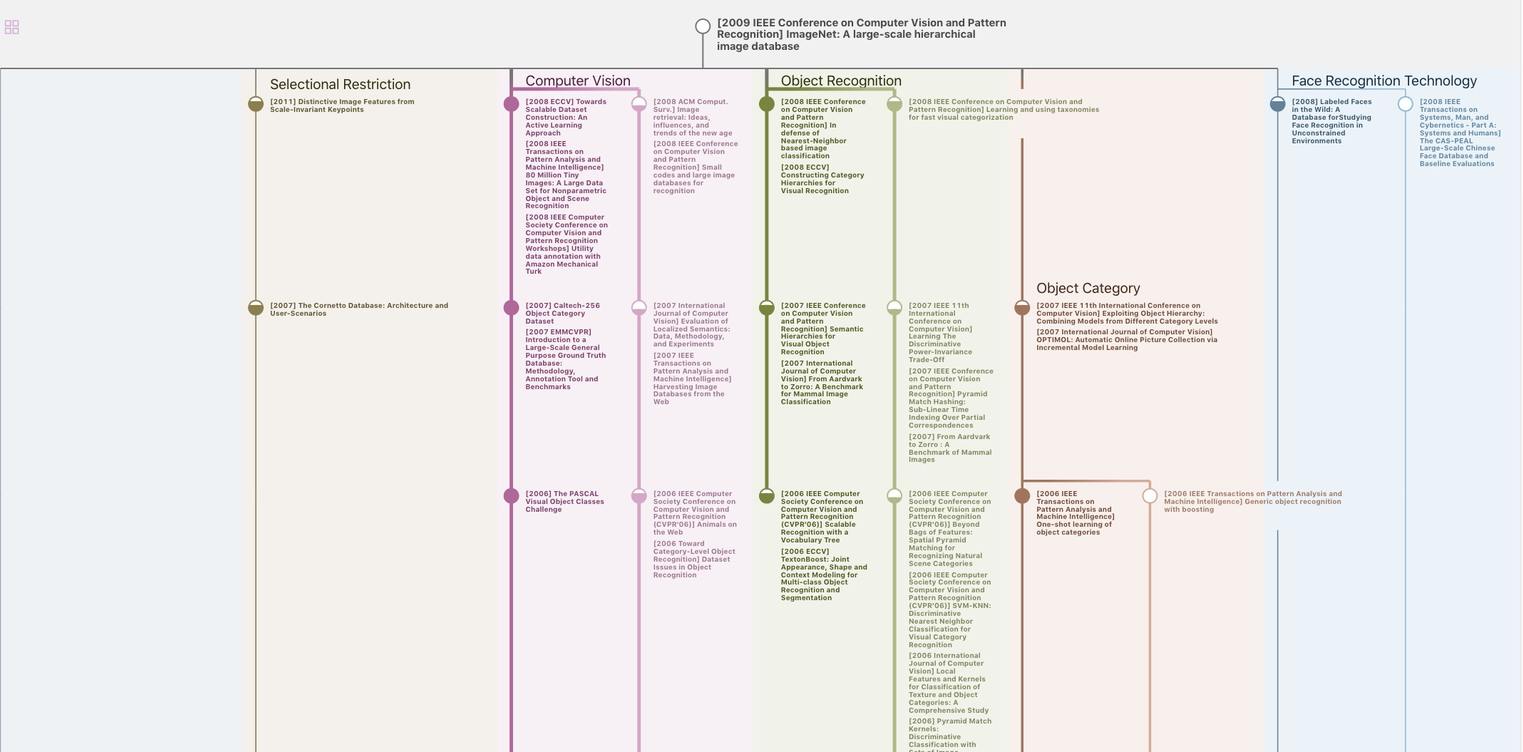
生成溯源树,研究论文发展脉络
Chat Paper
正在生成论文摘要