Leveraging Deep Learning and Knowledge Distillation for Enhanced Traffic Anomaly Detection in Transportation Systems
2023 International Conference on Multimedia Analysis and Pattern Recognition (MAPR)(2023)
摘要
This paper introduces an innovative approach to enhance traffic anomaly detection in transportation systems using deep learning and knowledge distillation. We create a robust dataset from 427 videos containing 1,415 accident-related events, spanning various anomalies like accidents, car crashes, and pedestrian violations. To address real-time anomaly detection challenges, we propose a novel lightweight neural network architecture inspired by EfficientNet-B0, designed for efficient video anomaly detection. Through knowledge distillation, a student model learns from a teacher model’s predictions, resulting in heightened anomaly detection accuracy. Experimental results highlight the approach’s efficacy, with the knowledge-distilled student model consistently outperforming the standalone lightweight network, achieving an accuracy of 94.83% compared to 94.16%. This research offers a practical solution for real-time traffic anomaly detection, which is especially valuable in resource-constrained environments. Fusing a unique dataset, EfficientNet-B0-like structure, lightweight architecture, and knowledge distillation holds significant potential for fostering safer and more efficient transportation systems.
更多查看译文
关键词
Traffic Anomaly Detection,Knowledge Distillation,Video Analysis,Transportation Systems
AI 理解论文
溯源树
样例
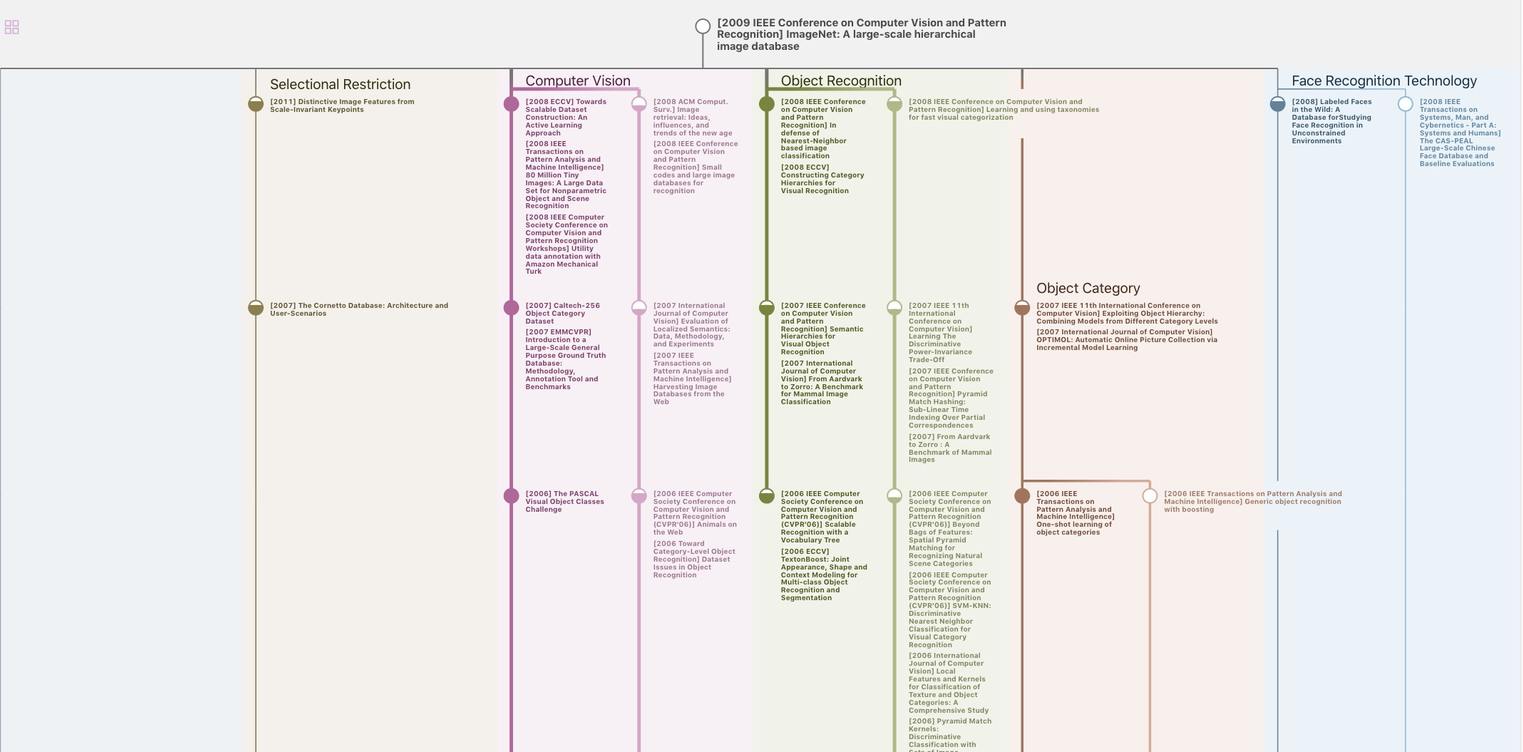
生成溯源树,研究论文发展脉络
Chat Paper
正在生成论文摘要