A Grammar-based multi-objective neuroevolutionary algorithm to generate fully convolutional networks with novel topologies
APPLIED SOFT COMPUTING(2023)
摘要
The design of complex and deep neural networks is often performed by identifying and combining building blocks and progressively selecting the most promising combination. Neuroevolution automates this process by employing evolutionary algorithms to guide the search. Within this field, grammar-based evolutionary algorithms have been demonstrated to be powerful tools to describe and thus encode complex neural architectures effectively. Following this trend, the present work proposes a novel grammar-based multi-objective neuroevolutionary for generating Fully Convolutional Networks. The proposed method, named Multi-Objective gRammatical Evolution for FUlly convolutional Networks (MOREFUN), includes a new efficient way to encode skip connections, facilitating the description of complex search spaces and the injection of domain knowledge in the search procedure, generation of fully convolutional networks, upsampling of lower-resolution inputs in multi-input layers, usage of multi-objective fitness, and inclusion of data augmentation and optimiser settings to the grammar. Our best networks outperformed previous grammar evolution algorithms, achieving 90.5% accuracy on CIFAR-10 without using transfer learning, ensembles, or test-time data augmentation. Our best models had 13.39 +/- 5.25 trainable parameters and the evolutionary process required 90 min per generation.
更多查看译文
关键词
Grammatical evolution,Fully convolutional neural networks,Image classification,Neuroevolution
AI 理解论文
溯源树
样例
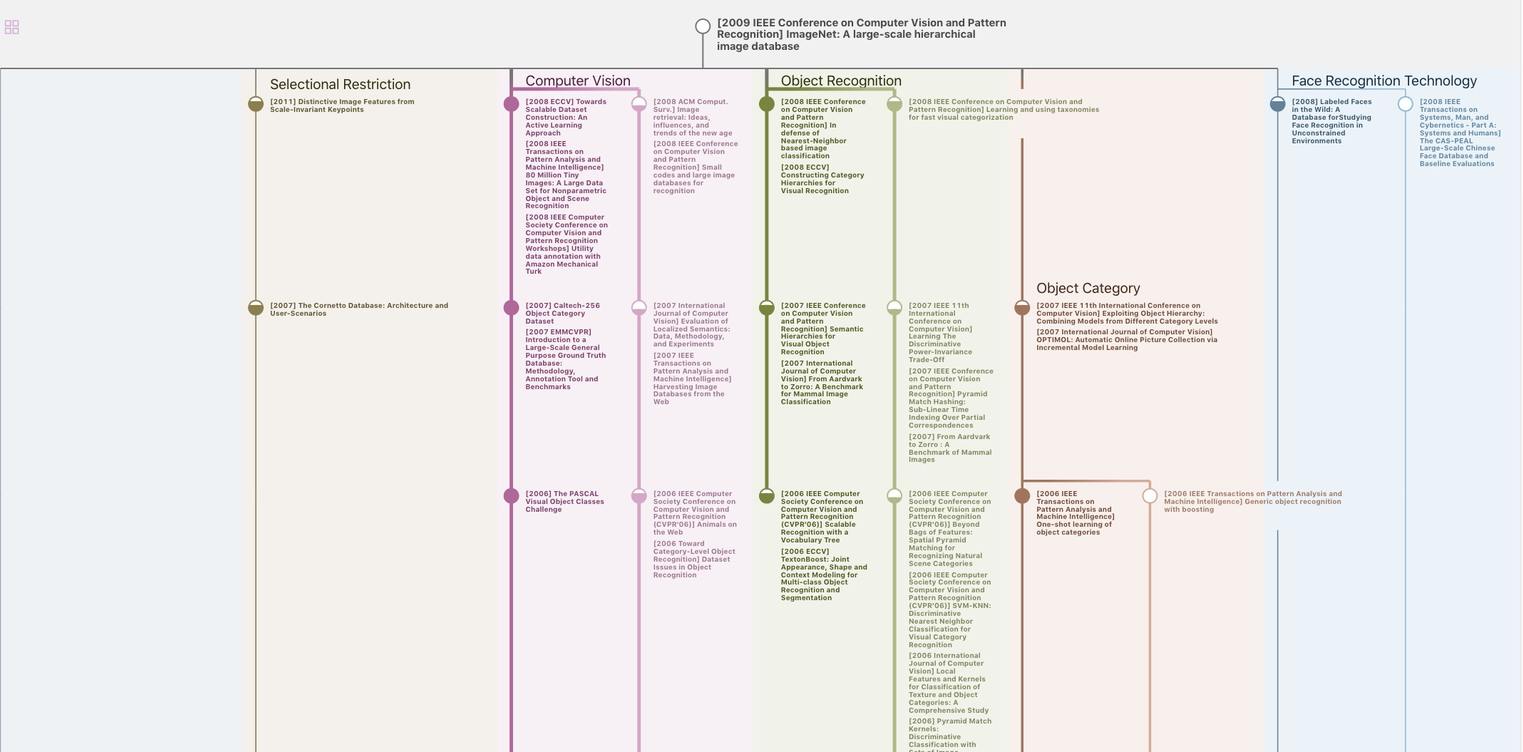
生成溯源树,研究论文发展脉络
Chat Paper
正在生成论文摘要