Comparing effectiveness of image perturbation and test retest imaging in improving radiomic model reliability
Scientific reports(2023)
摘要
Image perturbation is a promising technique to assess radiomic feature repeatability, but whether it can achieve the same effect as test–retest imaging on model reliability is unknown. This study aimed to compare radiomic model reliability based on repeatable features determined by the two methods using four different classifiers. A 191-patient public breast cancer dataset with 71 test–retest scans was used with pre-determined 117 training and 74 testing samples. We collected apparent diffusion coefficient images and manual tumor segmentations for radiomic feature extraction. Random translations, rotations, and contour randomizations were performed on the training images, and intra-class correlation coefficient (ICC) was used to filter high repeatable features. We evaluated model reliability in both internal generalizability and robustness, which were quantified by training and testing AUC and prediction ICC. Higher testing performance was found at higher feature ICC thresholds, but it dropped significantly at ICC = 0.95 for the test–retest model. Similar optimal reliability can be achieved with testing AUC = 0.7–0.8 and prediction ICC > 0.9 at the ICC threshold of 0.9. It is recommended to include feature repeatability analysis using image perturbation in any radiomic study when test–retest is not feasible, but care should be taken when deciding the optimal feature repeatability criteria.
更多查看译文
关键词
radiomic model reliability,retest imaging,image perturbation
AI 理解论文
溯源树
样例
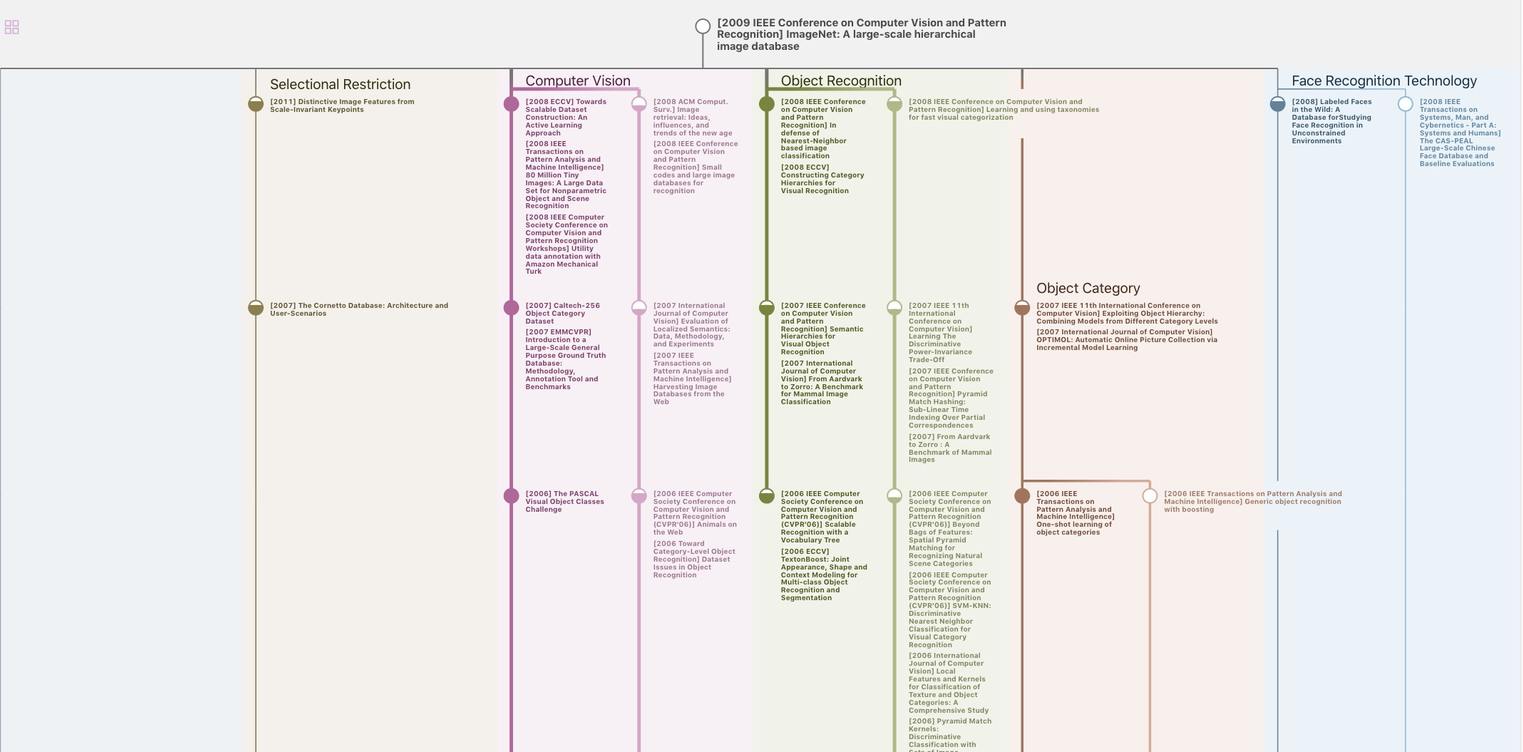
生成溯源树,研究论文发展脉络
Chat Paper
正在生成论文摘要