Hierarchical Ensemble-Based Feature Selection for Time Series Forecasting
CoRR(2023)
摘要
We introduce a novel ensemble approach for feature selection based on
hierarchical stacking for non-stationarity and/or a limited number of samples
with a large number of features. Our approach exploits the co-dependency
between features using a hierarchical structure. Initially, a machine learning
model is trained using a subset of features, and then the output of the model
is updated using other algorithms in a hierarchical manner with the remaining
features to minimize the target loss. This hierarchical structure allows for
flexible depth and feature selection. By exploiting feature co-dependency
hierarchically, our proposed approach overcomes the limitations of traditional
feature selection methods and feature importance scores. The effectiveness of
the approach is demonstrated on synthetic and well-known real-life datasets,
providing significant scalable and stable performance improvements compared to
the traditional methods and the state-of-the-art approaches. We also provide
the source code of our approach to facilitate further research and
replicability of our results.
更多查看译文
关键词
feature selection,forecasting,time series
AI 理解论文
溯源树
样例
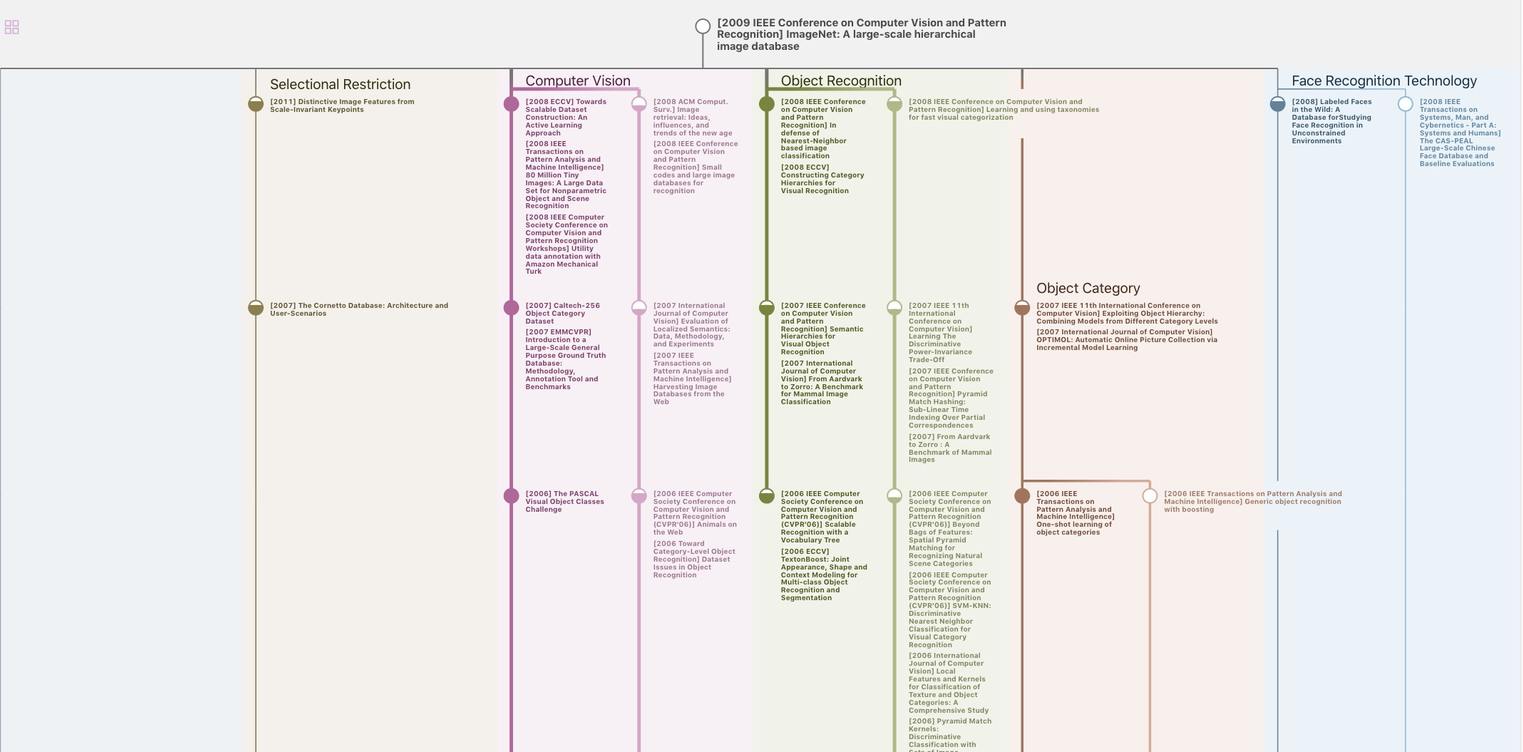
生成溯源树,研究论文发展脉络
Chat Paper
正在生成论文摘要