Improving Zero-shot Reader by Reducing Distractions from Irrelevant Documents in Open-Domain Question Answering.
CoRR(2023)
摘要
Large language models (LLMs) enable zero-shot approaches in open-domain question answering (ODQA), yet with limited advancements as the reader is compared to the retriever. This study aims at the feasibility of a zero-shot reader that addresses the challenges of computational cost and the need for labeled data. We find that LLMs are distracted due to irrelevant documents in the retrieved set and the overconfidence of the generated answers when they are exploited as zero-shot readers. To tackle these problems, we mitigate the impact of such documents via Distraction-aware Answer Selection (DAS) with a negation-based instruction and score adjustment for proper answer selection. Experimental results show that our approach successfully handles distraction across diverse scenarios, enhancing the performance of zero-shot readers. Furthermore, unlike supervised readers struggling with unseen data, zero-shot readers demonstrate outstanding transferability without any training.
更多查看译文
关键词
distractions,open-domain open-domain question,zero-shot
AI 理解论文
溯源树
样例
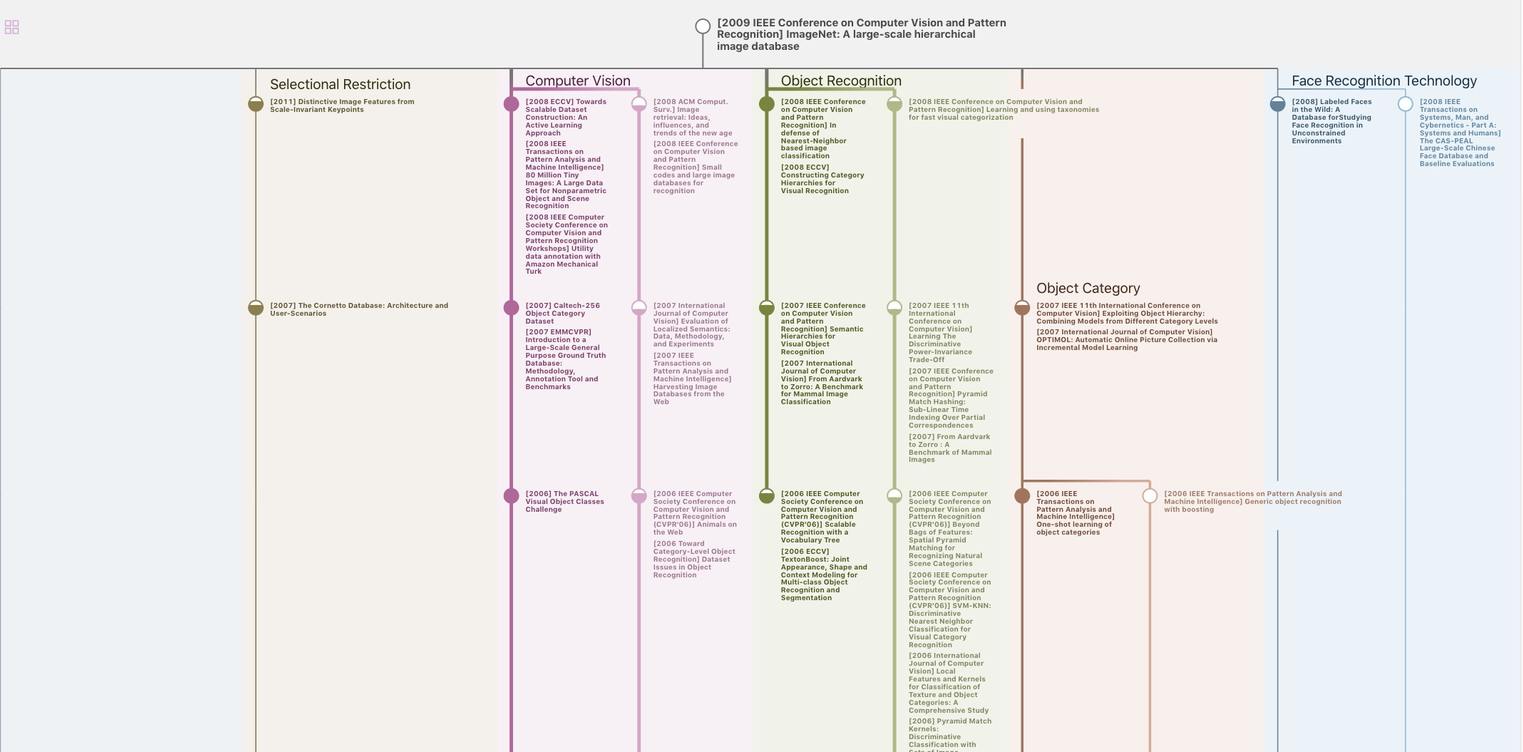
生成溯源树,研究论文发展脉络
Chat Paper
正在生成论文摘要