MaxEnt Loss: Constrained Maximum Entropy for Calibration under Out-of-Distribution Shift
AAAI 2024(2024)
摘要
We present a new loss function that addresses the out-of-distribution (OOD) network calibration problem. While many objective functions have been proposed to effectively calibrate models in-distribution, our findings show that they do not always fare well OOD. Based on the Principle of Maximum Entropy, we incorporate helpful statistical constraints observed during training, delivering better model calibration without sacrificing accuracy. We provide theoretical analysis and show empirically that our method works well in practice, achieving state-of-the-art calibration on both synthetic and real-world benchmarks. Our code is available at https://github.com/dexterdley/MaxEnt-Loss.
更多查看译文
关键词
General
AI 理解论文
溯源树
样例
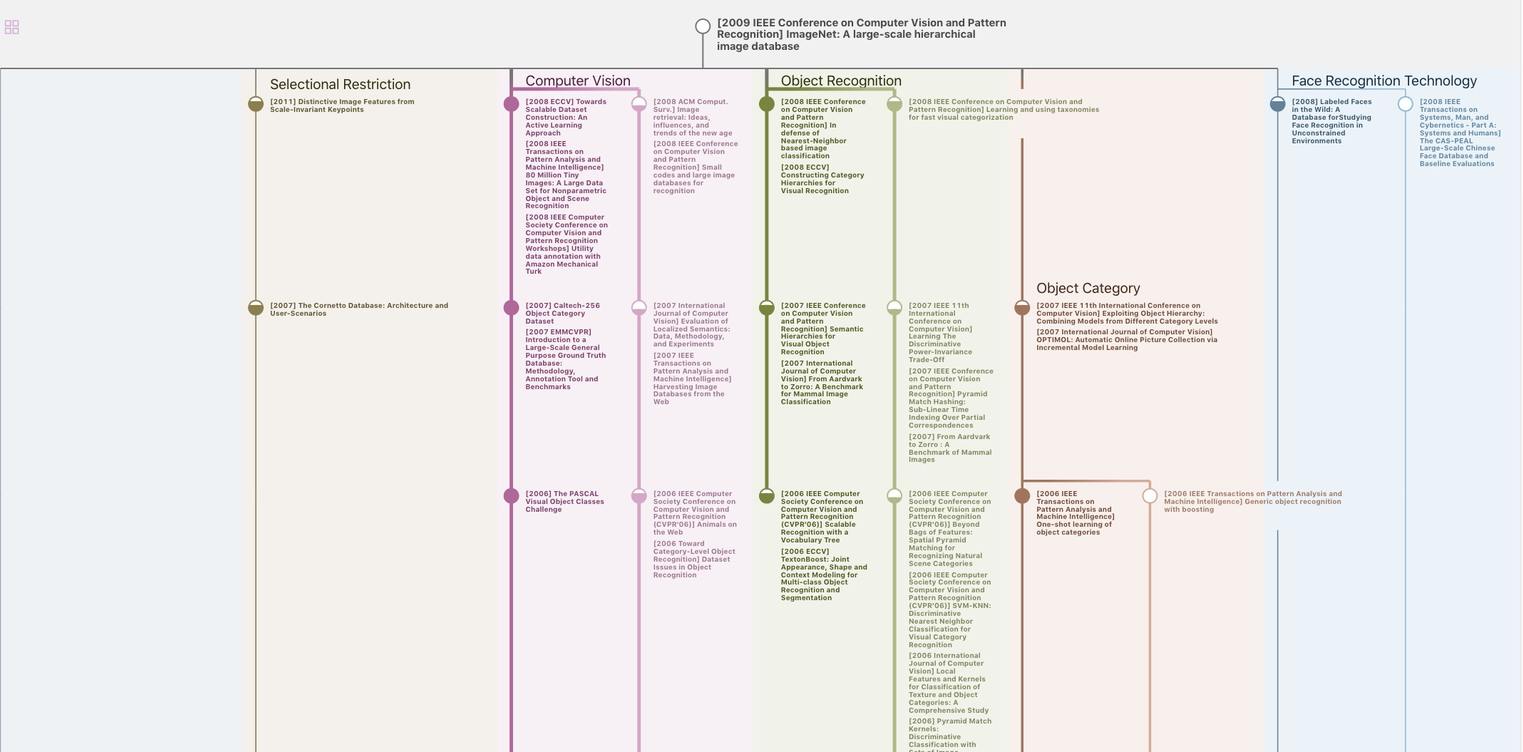
生成溯源树,研究论文发展脉络
Chat Paper
正在生成论文摘要