Detecting stealthy cyberattacks on adaptive cruise control vehicles: A machine learning approach
CoRR(2023)
摘要
With the advent of vehicles equipped with advanced driver-assistance systems, such as adaptive cruise control (ACC) and other automated driving features, the potential for cyberattacks on these automated vehicles (AVs) has emerged. While overt attacks that force vehicles to collide may be easily identified, more insidious attacks, which only slightly alter driving behavior, can result in network-wide increases in congestion, fuel consumption, and even crash risk without being easily detected. To address the detection of such attacks, we first present a traffic model framework for three types of potential cyberattacks: malicious manipulation of vehicle control commands, false data injection attacks on sensor measurements, and denial-of-service (DoS) attacks. We then investigate the impacts of these attacks at both the individual vehicle (micro) and traffic flow (macro) levels. A novel generative adversarial network (GAN)-based anomaly detection model is proposed for real-time identification of such attacks using vehicle trajectory data. We provide numerical evidence {to demonstrate} the efficacy of our machine learning approach in detecting cyberattacks on ACC-equipped vehicles. The proposed method is compared against some recently proposed neural network models and observed to have higher accuracy in identifying anomalous driving behaviors of ACC vehicles.
更多查看译文
关键词
stealthy cyberattacks,adaptive cruise control vehicles
AI 理解论文
溯源树
样例
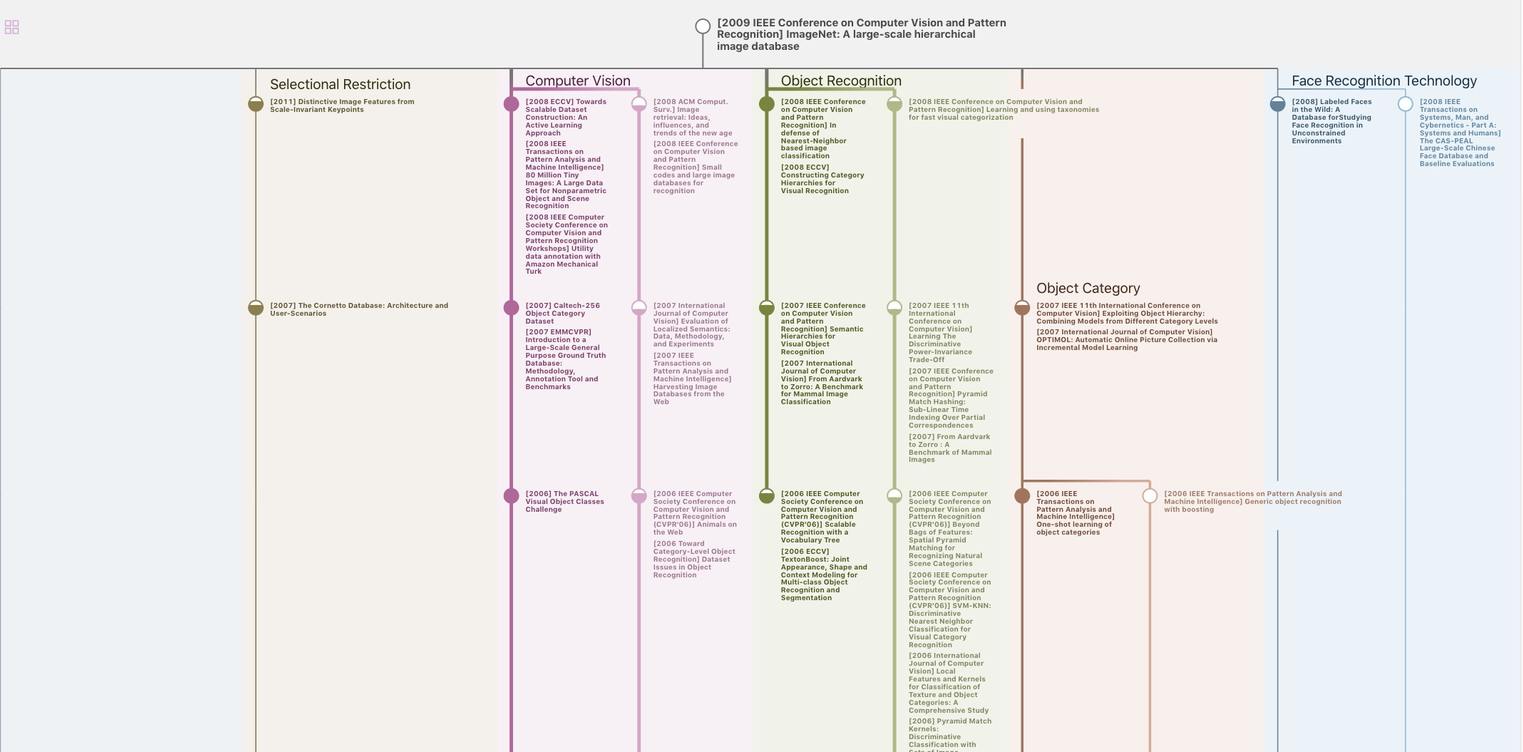
生成溯源树,研究论文发展脉络
Chat Paper
正在生成论文摘要