Harnessing Black-Box Control to Boost Commonsense in LM's Generation.
CoRR(2023)
摘要
Large language models (LLMs) such as GPT-3 have demonstrated a strong capability to generate coherent and contextually relevant text. However, amidst their successes, a crucial issue persists: their generated outputs still lack commonsense at times. Moreover, fine-tuning the entire LLM towards more commonsensical outputs is computationally expensive if not infeasible. In this paper, we present a computation-efficient framework that steers a frozen Pre-Trained Language Model (PTLM) towards more commonsensical generation (i.e., producing a plausible output that incorporates a list of concepts in a meaningful way). Specifically, we first construct a reference-free evaluator that assigns a sentence with a commonsensical score by grounding the sentence to a dynamic commonsense knowledge base from four different relational aspects. We then use the scorer as the oracle for commonsense knowledge, and extend the controllable generation method called NADO to train an auxiliary head that guides a fixed PTLM to better satisfy the oracle. We test our framework on a series of GPT-2-, Flan-T5-, and Alpaca-based language models (LMs) on two constrained concept-to-sentence benchmarks. Human evaluation results demonstrate that our method consistently leads to the most commonsensical outputs.
更多查看译文
关键词
lms
AI 理解论文
溯源树
样例
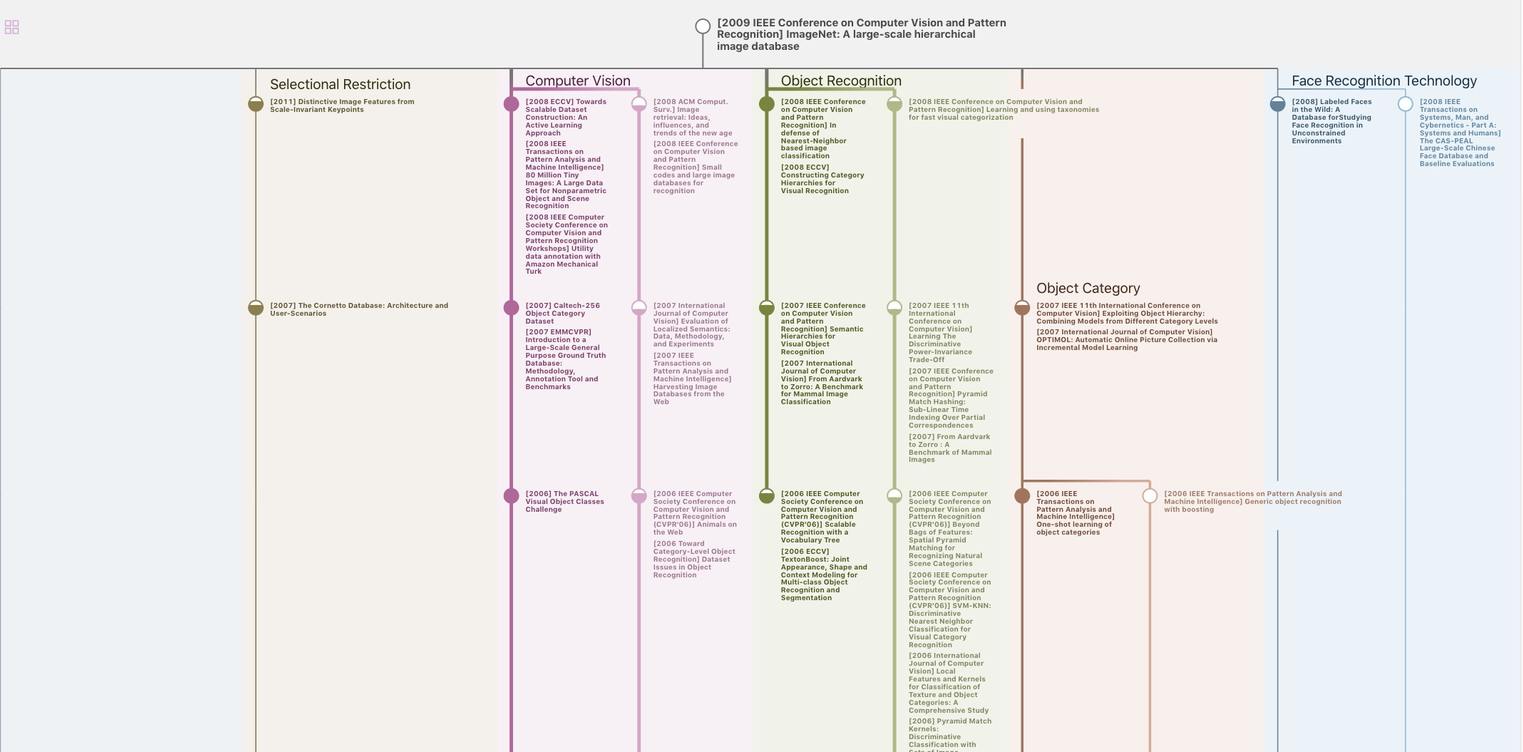
生成溯源树,研究论文发展脉络
Chat Paper
正在生成论文摘要