Hypergraph-Based Resource-Efficient Collaborative Reinforcement Learning for B5G Massive IoT
IEEE OPEN JOURNAL OF THE COMMUNICATIONS SOCIETY(2023)
摘要
Beyond 5G (B5G) networks rapidly growing to connect billions of Internet of Things (IoT) devices and the dense deployment of IoT devices leads the large-scale network conflict and obstacles the resource-efficient, which brings a great challenge for network resource management (NRM). To tackle this problem, hypergraph based resource-efficient collaborative reinforcement learning (CRL) was proposed for B5G massive IoT. Firstly, the hypergraph theory based network conflict model was formulated to quantify the conflict degree of the B5G massive IoT. Then, since the conflict-free resource management problem is a combinatorial optimization problem with NP-hard, the resource management based Markov decision process (MDP) model was built for NRM in B5G massive IoT. To reduce the computational load by distributing the training overhead throughout the entire B5G massive IoT and achieve distributed collaborative learning, the federated averaging advantage Actor-Critic (FedAvg-A2C) based resource management is proposed to handle the network conflict-free resource management problem and accelerate the training process. Simulation results show the proposed scheme has high network throughput and the resource-efficient in B5G massive IoT.
更多查看译文
关键词
Hypergraph,collaborative reinforcement learning,resource-efficient,B5G massive IoT
AI 理解论文
溯源树
样例
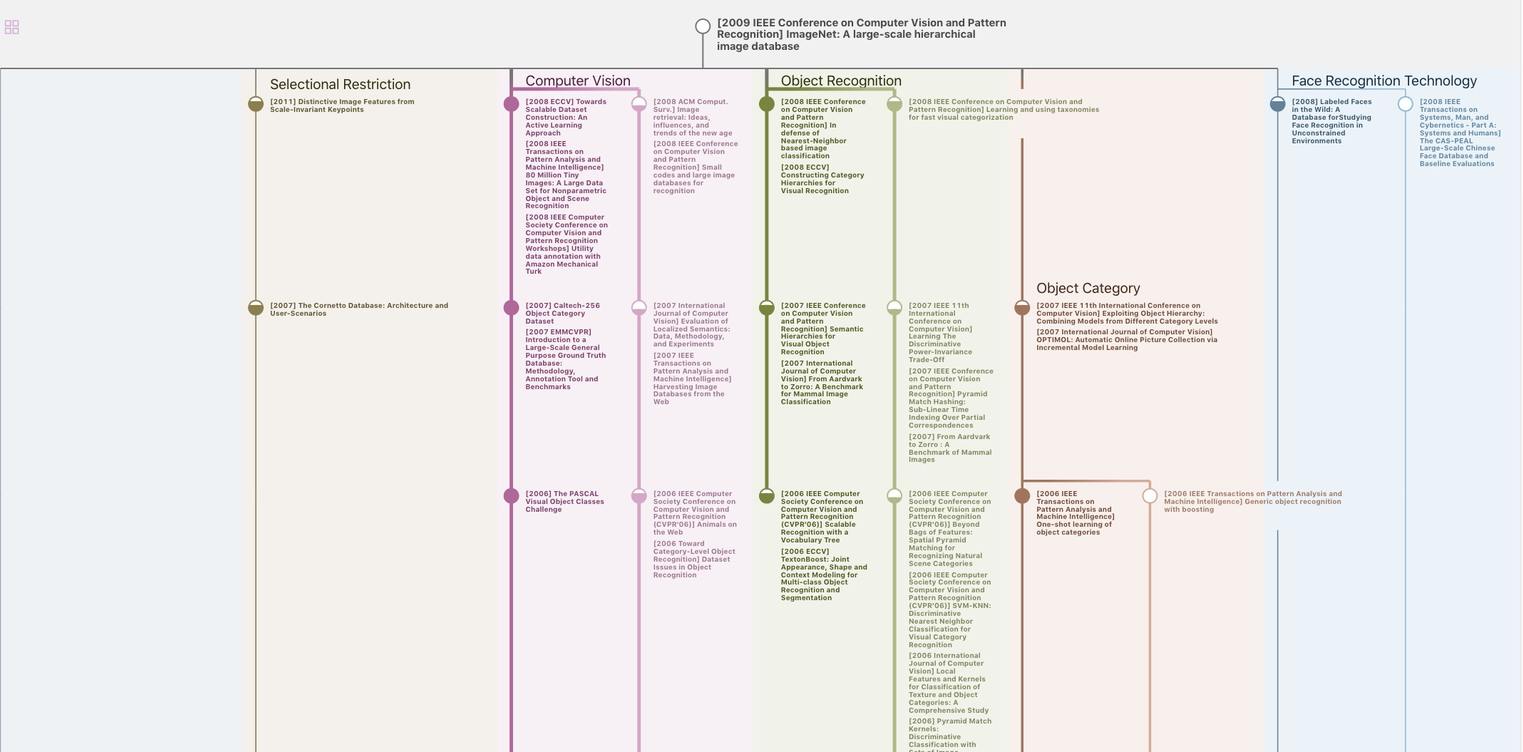
生成溯源树,研究论文发展脉络
Chat Paper
正在生成论文摘要