OpenFL-XAI: Federated learning of explainable artificial intelligence models in Python
SOFTWAREX(2023)
摘要
Artificial Intelligence (AI) systems play a significant role in manifold decision-making processes in our daily lives, making trustworthiness of AI more and more crucial for its widespread acceptance. Among others, privacy and explainability are considered key requirements for enabling trust in AI. Building on these needs, we propose a software for Federated Learning (FL) of Rule-Based Systems (RBSs): on one hand FL prioritizes user data privacy during collaborative model training. On the other hand, RBSs are deemed as interpretable-by-design models and ensure high transparency in the decisionmaking process. The proposed software, developed as an extension to the Intel (R) OpenFL open-source framework, offers a viable solution for developing AI applications balancing accuracy, privacy, and interpretability. (c) 2023 The Author(s). Published by Elsevier B.V. This is an open access article under the CC BY license (http://creativecommons.org/licenses/by/4.0/).
更多查看译文
关键词
Federated learning,Explainable AI,Rule-based systems,Linguistic fuzzy models
AI 理解论文
溯源树
样例
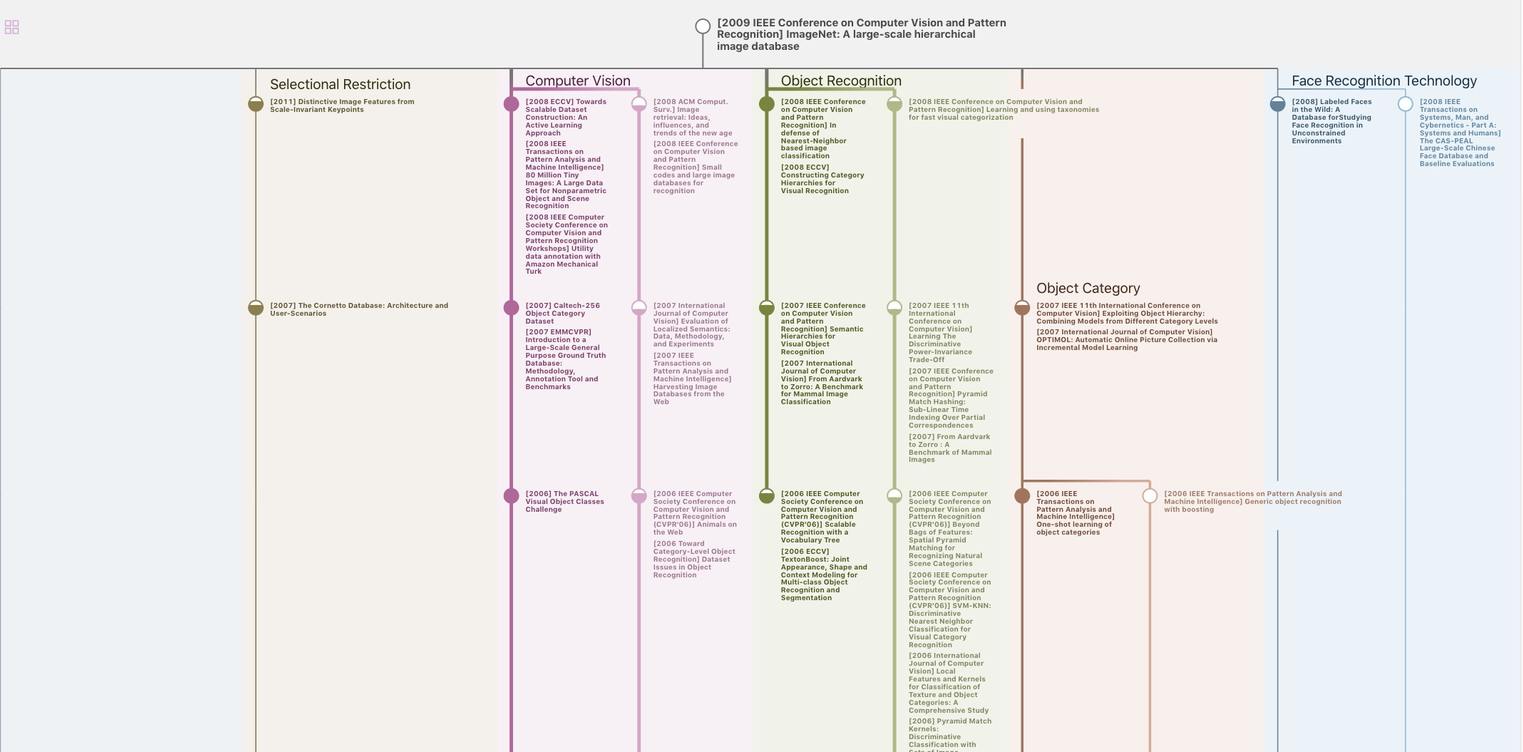
生成溯源树,研究论文发展脉络
Chat Paper
正在生成论文摘要