Structure-preserving feature alignment for old photo colorization
PATTERN RECOGNITION(2024)
摘要
Deep learning techniques have made significant advancements in reference-based colorization by training on large-scale datasets. However, directly applying these methods to the task of colorizing old photos is challenging due to the lack of ground truth and the notorious domain gap between natural gray images and old photos. To address this issue, we propose a novel CNN-based algorithm called SFAC, i.e., Structure-preserving Feature Alignment Colorizer. SFAC is trained on only two images for old photo colorization, eliminating the reliance on big data and allowing direct processing of the old photo itself to overcome the domain gap problem. Our primary objective is to establish semantic correspondence between the two images, ensuring that semantically related objects have similar colors. We achieve this through a feature distribution alignment loss that remains robust to different metric choices. However, utilizing robust semantic correspondence to transfer color from the reference to the old photo can result in inevitable structure distortions. To mitigate this, we introduce a structure-preserving mechanism that incorporates a perceptual constraint at the feature level and a frozen-updated pyramid at the pixel level. Extensive experiments demonstrate the effectiveness of our method for old photo colorization, as confirmed by qualitative and quantitative metrics.
更多查看译文
关键词
Old photo colorization,Structure preservation,Feature alignment,Hierarchical training
AI 理解论文
溯源树
样例
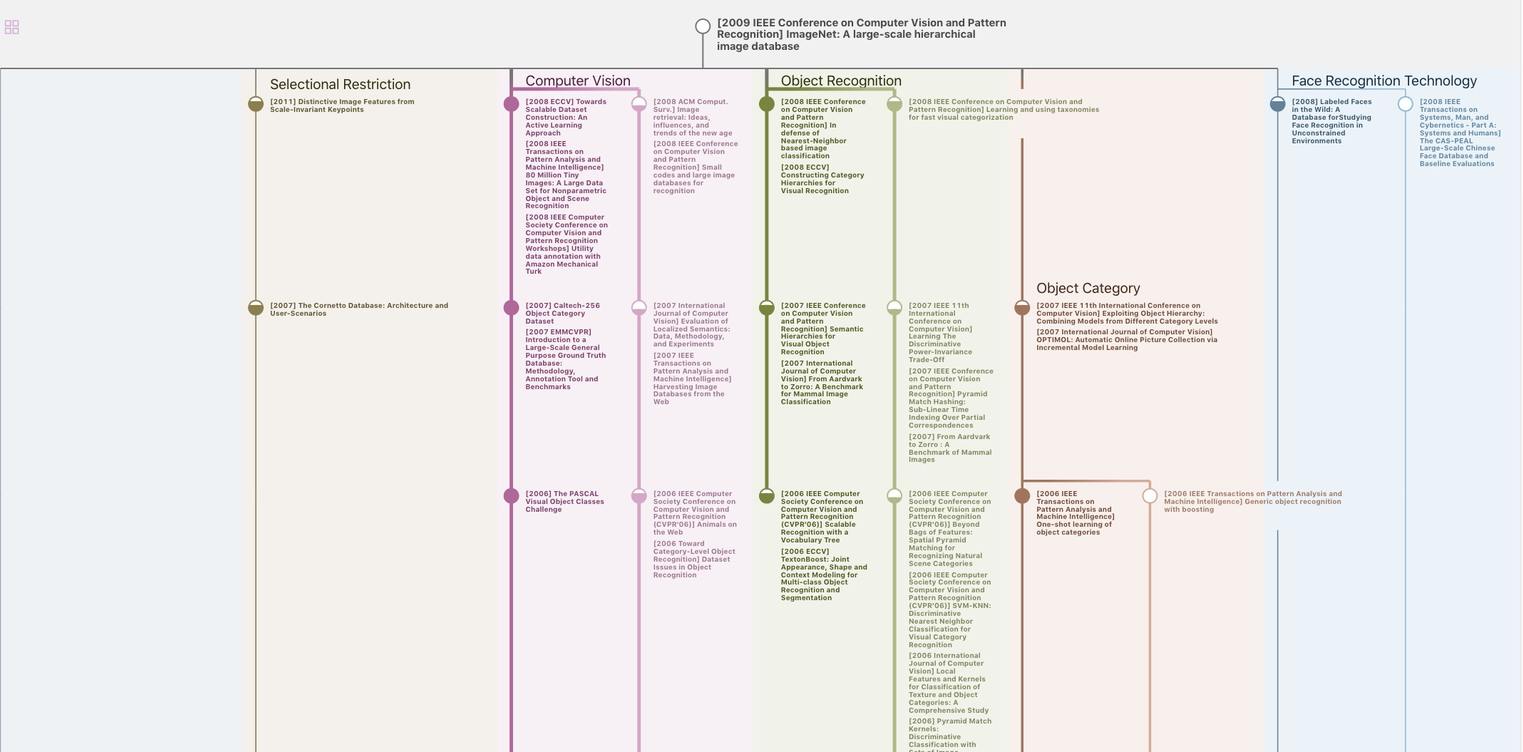
生成溯源树,研究论文发展脉络
Chat Paper
正在生成论文摘要