Robust inference for subgroup analysis with general transformation models
JOURNAL OF STATISTICAL PLANNING AND INFERENCE(2024)
摘要
A crucial step in developing personalized strategies in precision medicine or precision marketing is to identify the latent subgroups of patients or customers of a heterogeneous population. In this article, we consider a general class of heterogeneous transformation models for subgroup identification, under which an unknown monotonic transformation of the response is linearly related to the covariates via subject-specific regression coefficients with unknown error distribution. This class of models is broad enough to cover many popular models, including the heterogeneous linear model, the heteroge-neous Cox's proportional hazard model and the heterogeneous proportional odds model. Without any priori grouping information, we propose a robust method based on the maximum rank correlation and a concave fusion to automatically determine the number of subgroups, identify the latent subgroup structure, and estimate the subgroup-specific covariate effects simultaneously. We establish the theoretical properties of our proposed estimate under regularity conditions. A random weighting resampling scheme is used for variance estimation. The proposed procedure can be easily extended to handle censored data. Numerical studies including simulations and two real data analysis demonstrate that the proposed method performs reasonably well in practical situations.(c) 2023 Elsevier B.V. All rights reserved.
更多查看译文
关键词
Censored data,Heterogeneity,Subgroup analysis,Transformation models
AI 理解论文
溯源树
样例
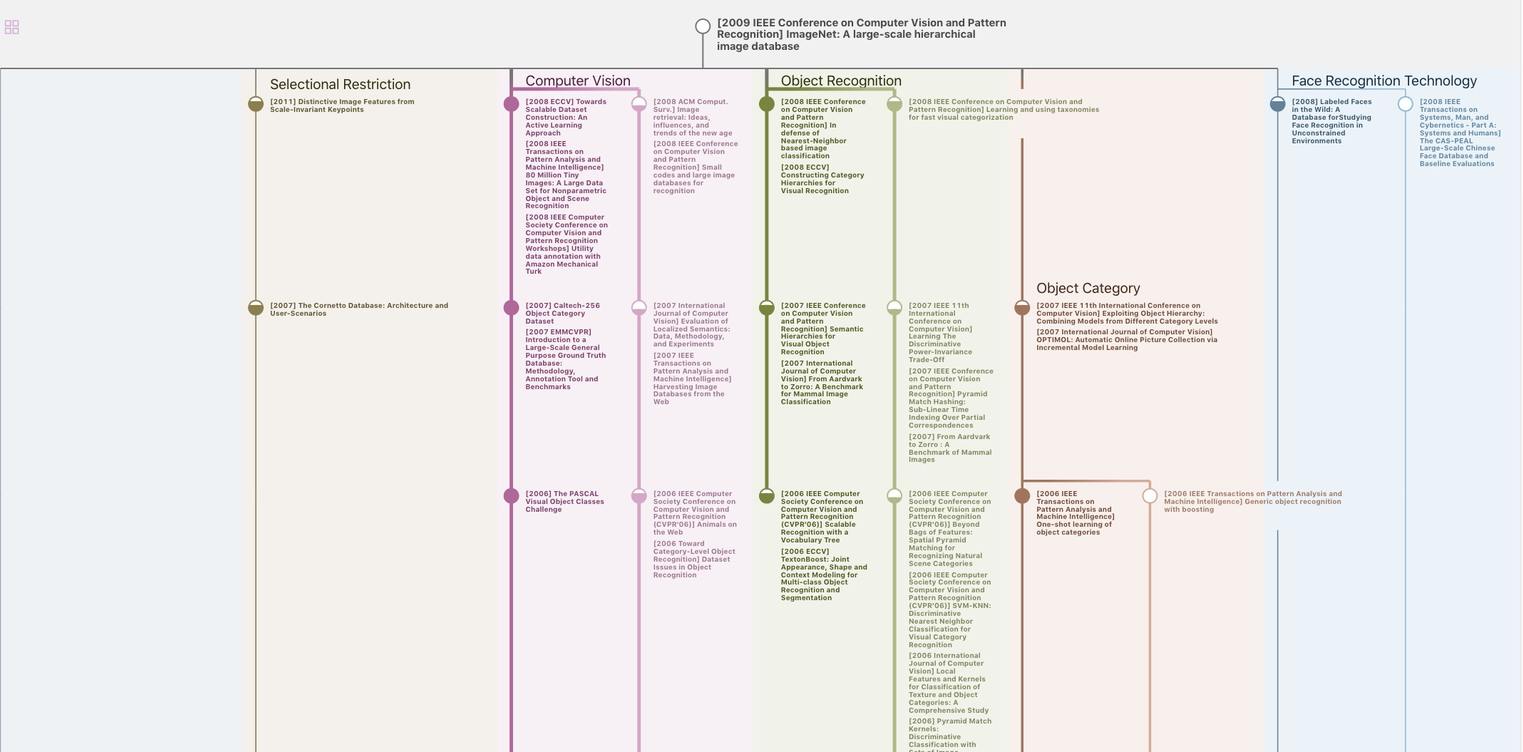
生成溯源树,研究论文发展脉络
Chat Paper
正在生成论文摘要