Residential water and energy consumption prediction at hourly resolution based on a hybrid machine learning approach
WATER RESEARCH(2023)
摘要
Predicting water and energy consumption at high resolution over a short-term horizon is critical for water and energy resource management. Water and energy are shown to be closely interlinked in household consumption. However, hourly predictions are often based only on historical consumption data for the resource being predicted, with activity or appliance information and household attribution as additional information. Few studies have used aggregated water and energy consumption for predictions. Within this context, the current study proposed a novel hybrid machine learning model based on the Prophet time-series model, Gated Recurrent Unit network, and self-adaptive weights, called the Prophet-GRU model, which could jointly include historical water and electricity consumption as inputs for hourly water or electricity prediction. Data on hourly water and electricity consumption in six households in Beijing during January-March 2020 were used to train and validate the Prophet-GRU model. The goodness of fit indicator (R2) and prediction accuracy (mean squared error and mean absolute error) for the water and electricity predictions were evaluated. Compared with the single input of water or electricity, with the combined input of data of these two resources, the proposed Prophet-GRU model achieved improvements of 29.2 % and 48.5 % in R2, for water and electricity consumption prediction, respectively. Our results could help better understand water-energy linkages and promote collaborative water and energy management practices.
更多查看译文
关键词
Deep learning,Water-energy nexus,Hourly resolution,Residential consumption
AI 理解论文
溯源树
样例
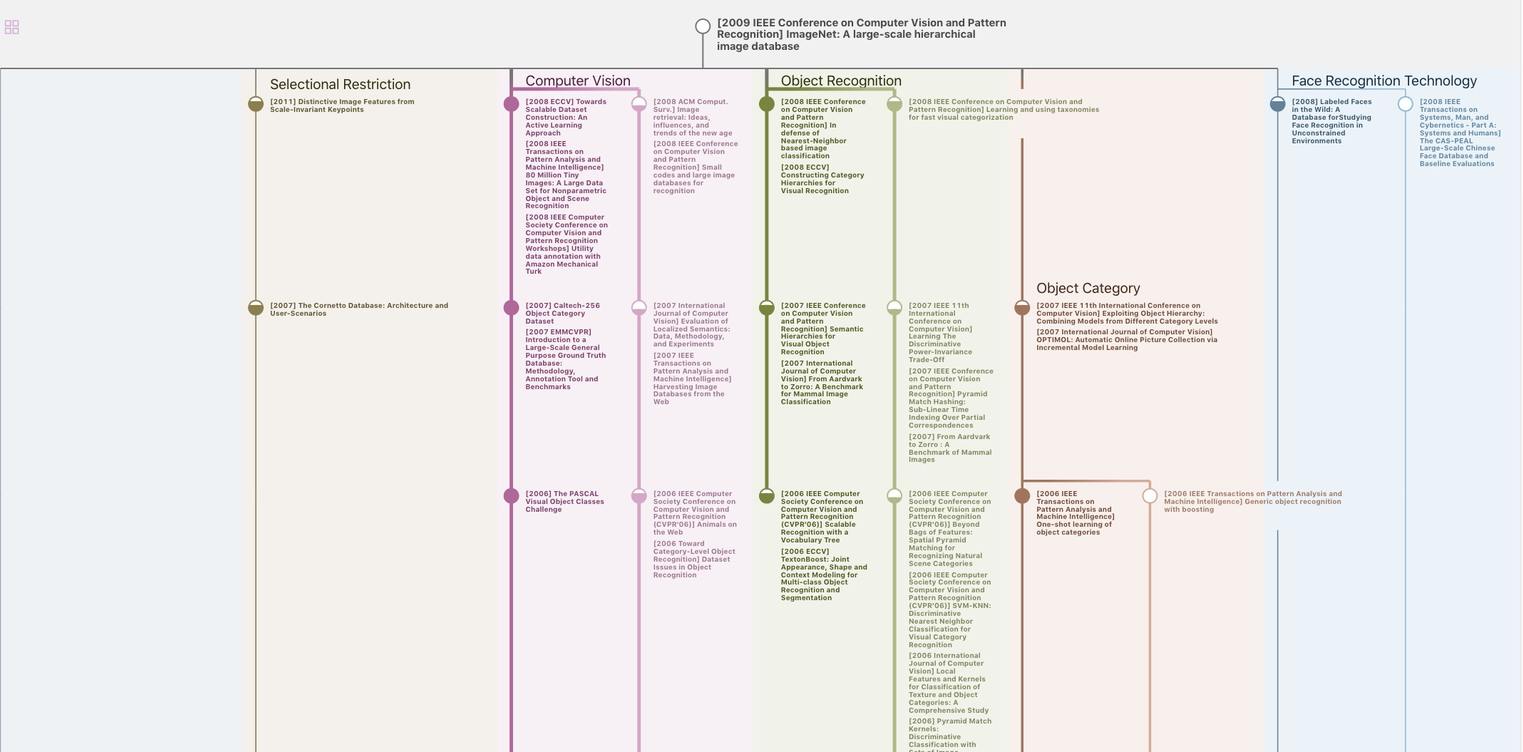
生成溯源树,研究论文发展脉络
Chat Paper
正在生成论文摘要