DNN controlled adaptive front-end for replay attack detection systems
Speech Communication(2023)
摘要
Developing robust countermeasures to protect automatic speaker verification systems against replay spoofing attacks is a well-recognized challenge. Current approaches to spoofing detection are generally based on a fixed front-end, typically a time-invariant filter bank, followed by a machine learning back-end. In this paper, we propose a novel approach whereby the front-end comprises an adaptive filter bank with a deep neural networkbased controller, which is jointly trained along with a neural network back-end. Specifically, the deep neural network-based adaptive filter controller tunes the selectivity and sensitivity of the front-end filter bank at every frame to capture replay-related artefacts. We demonstrate the effectiveness of the proposed framework in spoofing attack detection on a synthesized dataset and ASVSpoof 2019 and ASVSpoof 2021 challenge datasets in terms of equal error rate and its ability to capture artefacts that differentiate replayed signals from genuine ones in comparison to conventional non-adaptive front-ends.
更多查看译文
关键词
Adaptive feature extraction,Anti-spoofing,Auditory system modeling,Deep learning,Automatic speaker verification
AI 理解论文
溯源树
样例
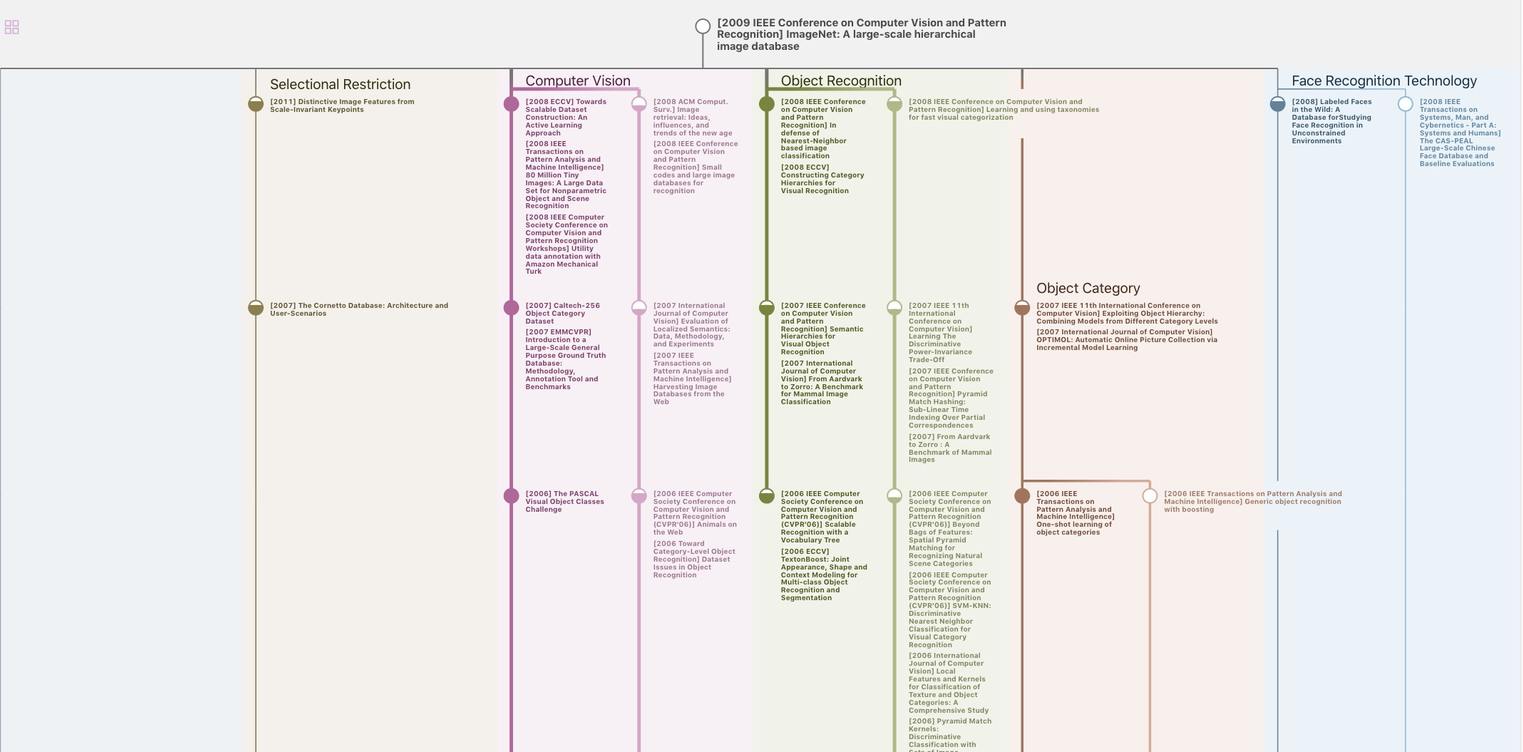
生成溯源树,研究论文发展脉络
Chat Paper
正在生成论文摘要