Introducing instance label correlation in multiple instance learning. Application to cancer detection on histopathological images
PATTERN RECOGNITION(2024)
摘要
In the last years, the weakly supervised paradigm of multiple instance learning (MIL) has become very popular in many different areas. A paradigmatic example is computational pathology, where the lack of patch-level labels for whole-slide images prevents the application of supervised models. Probabilistic MIL methods based on Gaussian Processes (GPs) have obtained promising results due to their excellent uncertainty estimation capabilities. However, these are general-purpose MIL methods that do not take into account one important fact: in (histopathological) images, the labels of neighboring patches are expected to be correlated. In this work, we extend a state-of-the-art GP-based MIL method, which is called VGPMIL-PR, to exploit such correlation. To do so, we develop a novel coupling term inspired by the statistical physics Ising model. We use variational inference to estimate all the model parameters. Interestingly, the VGPMIL-PR formulation is recovered when the weight that regulates the strength of the Ising term vanishes. The performance of the proposed method is assessed in two real-world problems of prostate cancer detection. We show that our model achieves better results than other state-of-the-art probabilistic MIL methods. We also provide different visualizations and analysis to gain insights into the influence of the novel Ising term. These insights are expected to facilitate the application of the proposed model to other research areas.
更多查看译文
关键词
Multiple instance learning,Gaussian processes,Ising model,Variational inference,Whole slide images,Histopathology
AI 理解论文
溯源树
样例
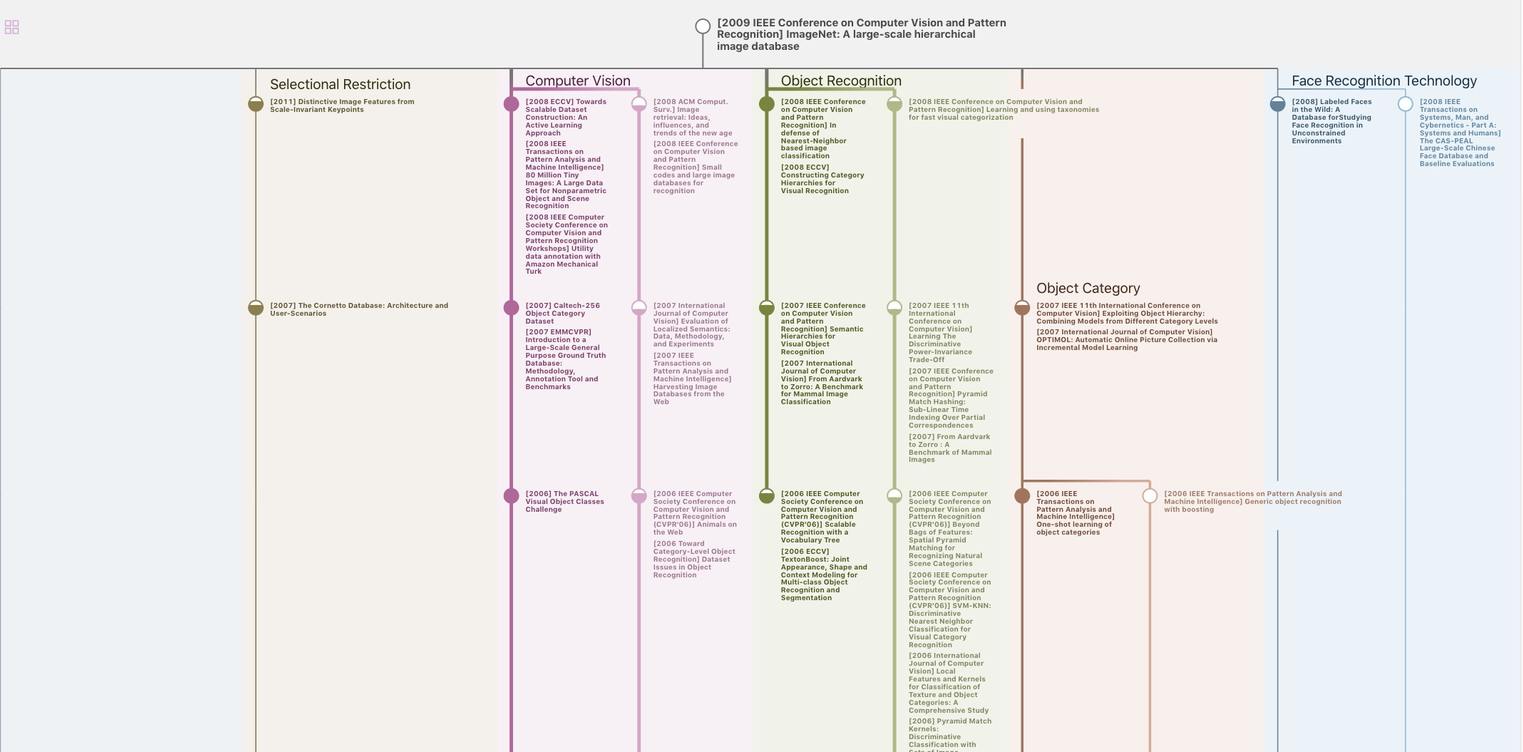
生成溯源树,研究论文发展脉络
Chat Paper
正在生成论文摘要