A novel ship trajectory clustering analysis and anomaly detection method based on AIS data
Ocean Engineering(2023)
Abstract
The increasing volume of ship traffic has resulted in new challenges for the supervision of maritime safety administration. The conventional manual monitoring approach for maritime traffic is inefficient and lacks specifics, particularly for supervising ships with abnormal trajectories. To address this issue, this study proposes utilizing the minimum description length criterion to extract features from ship trajectory data provided by the automatic identification system (AIS). This approach simplifies the compression of ship trajectories. Additionally, the dynamic time warping trajectory similarity measurement algorithm is employed to optimize the density-based spatial clustering of applications with noise algorithm. This optimization enables the clustering of ship trajectories and the acquisition of normalized ship motion trajectories. Furthermore, a ship trajectory prediction method based on a transformer model is proposed, and the normalized motion trajectory is used as the training set for model training. The trained ship trajectory prediction model is subsequently utilized to predict the target ship trajectory. The AIS ship trajectory data in the vicinity of Yantai Port were used for experimental verification. The results demonstrate the effectiveness of the proposed approach in identifying abnormal ship trajectories.
MoreTranslated text
Key words
Ship trajectory,Minimum description length,Dynamic time warping,Transformer
AI Read Science
Must-Reading Tree
Example
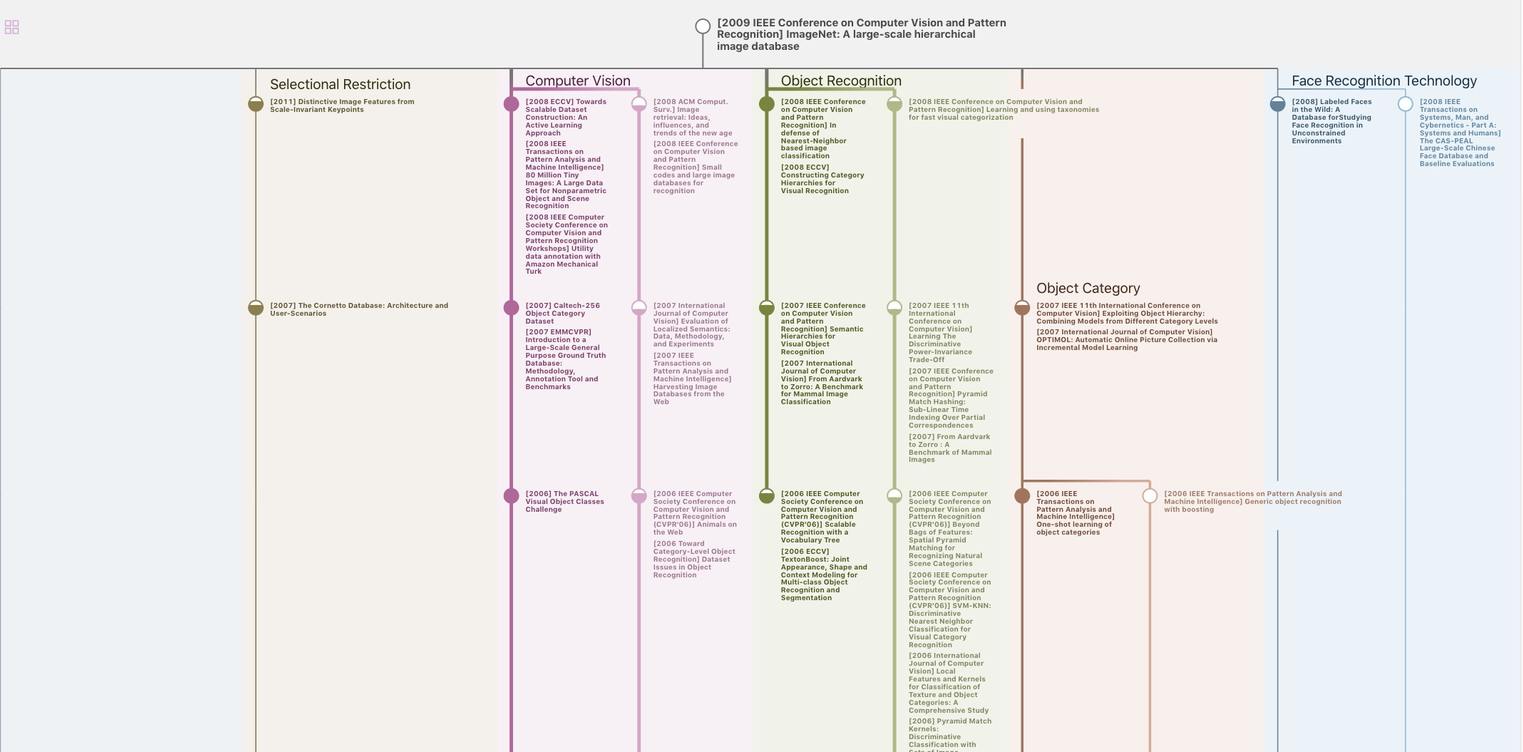
Generate MRT to find the research sequence of this paper
Chat Paper
Summary is being generated by the instructions you defined