Multi-view graph convolution network for the recognition of human action with spatial and temporal occlusion problems*
JOURNAL OF VISUAL COMMUNICATION AND IMAGE REPRESENTATION(2023)
摘要
Human action recognition holds great significance in the field of social security. However, the challenges posed by occlusion and changes in viewpoint create specific obstacles in achieving accurate recognition. In this study, we propose a multi-view graph convolution fusion method to effectively address this issue. Specifically, considering the limited availability of public human action datasets that include occlusion, we introduce an adaptive multi-view spatial-temporal occlusion generation method. It allows us to generate occlusion skeleton data from multiple viewpoints, ensuring a close resemblance to real-life scenarios with minimal modifications to existing public datasets. Additionally, we present a plug-and-play multi-view information fusion module, briefed as MGL, which aims to solve the occlusion problem. The MGL combines the capabilities of Graph Convolutional network (GCN) and Long Short-Term Memory network (LSTM), in which GCN is employed to reconstruct human skeleton information using multi-view spatial occlusion data, and LSTM captures the long-term dependencies within the temporal sequences of the multi-view data. Moreover, an attention mask mechanism is introduced to highlight key joint features. Experiment results illustrate the excellent performance of our method on the NTU RGB+D 60 and NTU RGB+D 120 datasets.
更多查看译文
关键词
Human action recognition,Spatial-temporal occlusion,Multi-view,Graph network
AI 理解论文
溯源树
样例
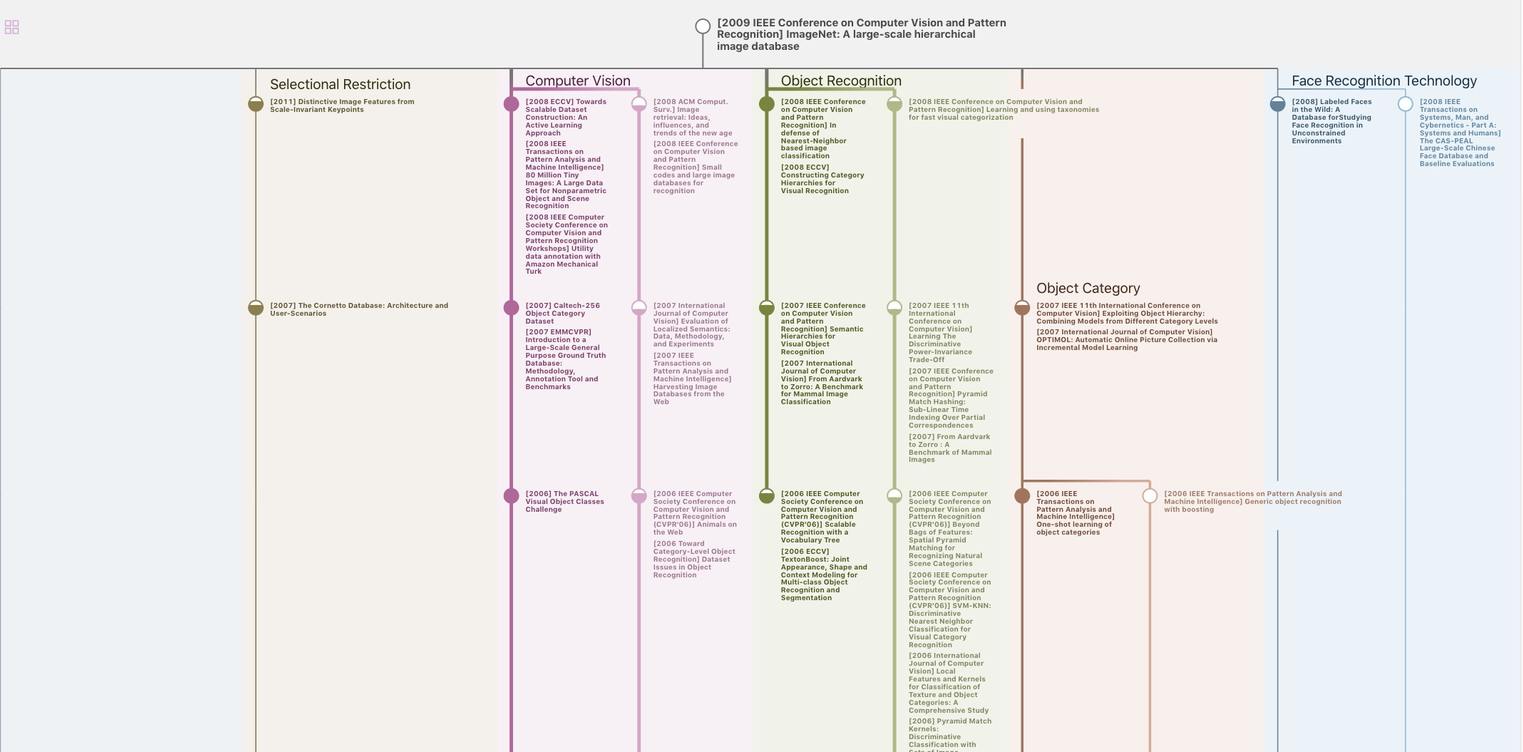
生成溯源树,研究论文发展脉络
Chat Paper
正在生成论文摘要