Long-term PM2.5 concentration prediction based on improved empirical mode decomposition and deep neural network combined with noise reduction auto-encoder- A case study in Beijing
Journal of Cleaner Production(2023)
摘要
Effective prediction of PM2.5 long-term concentration can help reduce exposure risks, but few current studies based on machine learning have been able to credibly predict concentration changing trends after 12 h. To address this issue, this study employed the complementary ensemble empirical mode decomposition (CEEMD) method combined with sample entropy (SE) index, as well as bidirectional long short-term memory (BiLSTM) model stacked denoising auto-encoder (AE) method for better decomposition and prediction of long-term trends in PM2.5 time series data. The new CEEMD-AE-BiLSTM model has the best long-term prediction results at different sites, and the R2 value is 0.871, and the RMSE is 9.28 μg/m3 at T+12 moment in the 1002A site. The new model effectively captures a significant portion of the long-term concentration trends characteristically and has been proven to be an effective tool for long-term predictions. This is attributed to the robust noise resistance of the AE method and the appropriate parameter setting of the CEEMD method.
更多查看译文
关键词
Long-term prediction model, PM2.5, CEEMD method, Sample entropy
AI 理解论文
溯源树
样例
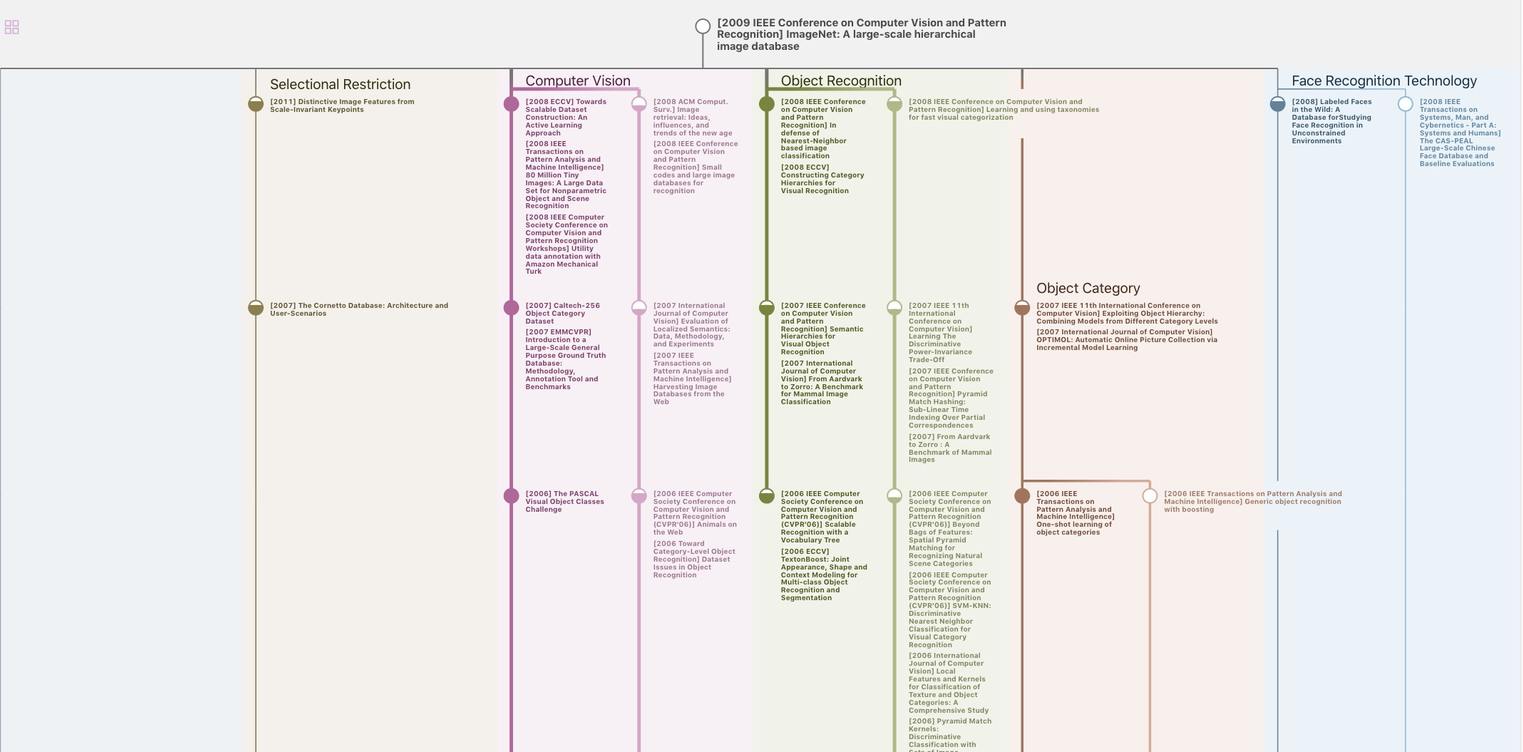
生成溯源树,研究论文发展脉络
Chat Paper
正在生成论文摘要