NT-DPTC: A non-negative temporal dimension preserved tensor completion model for missing traffic data imputation
INFORMATION SCIENCES(2024)
摘要
Missing traffic data imputation is an important step in the intelligent transportation systems. Low rank approximation is an important method for the missing traffic data imputation, especially the low rank matrix/tensor completion. However, the low-rank matrix completion has a huge time cost and the low-rank tensor completion cannot fully extract the information and maintain non-negativity. Therefore, we propose a novel non-negative temporal dimension preserved tensor completion (NT-DPTC) model with ideas: a) proposing a novel dimension preserved (DP) tensor decomposition method, which decomposes a tensor into three latent factor tensors to fully extract the intrinsic feature, b) using a Sigmoid mapper for releasing non-negative constraint from the training process and increasing flexibility, c) exploiting temporal constrains and AdamW schemes for obtaining a higher accuracy and faster convergence. Experiments on four industrial application scenarios highlight the superiority of our proposed model when compared with the existing state-of-the-art models.
更多查看译文
关键词
High-dimensional and sparse tensor,Tensor completion,Traffic data,Missing traffic data imputation
AI 理解论文
溯源树
样例
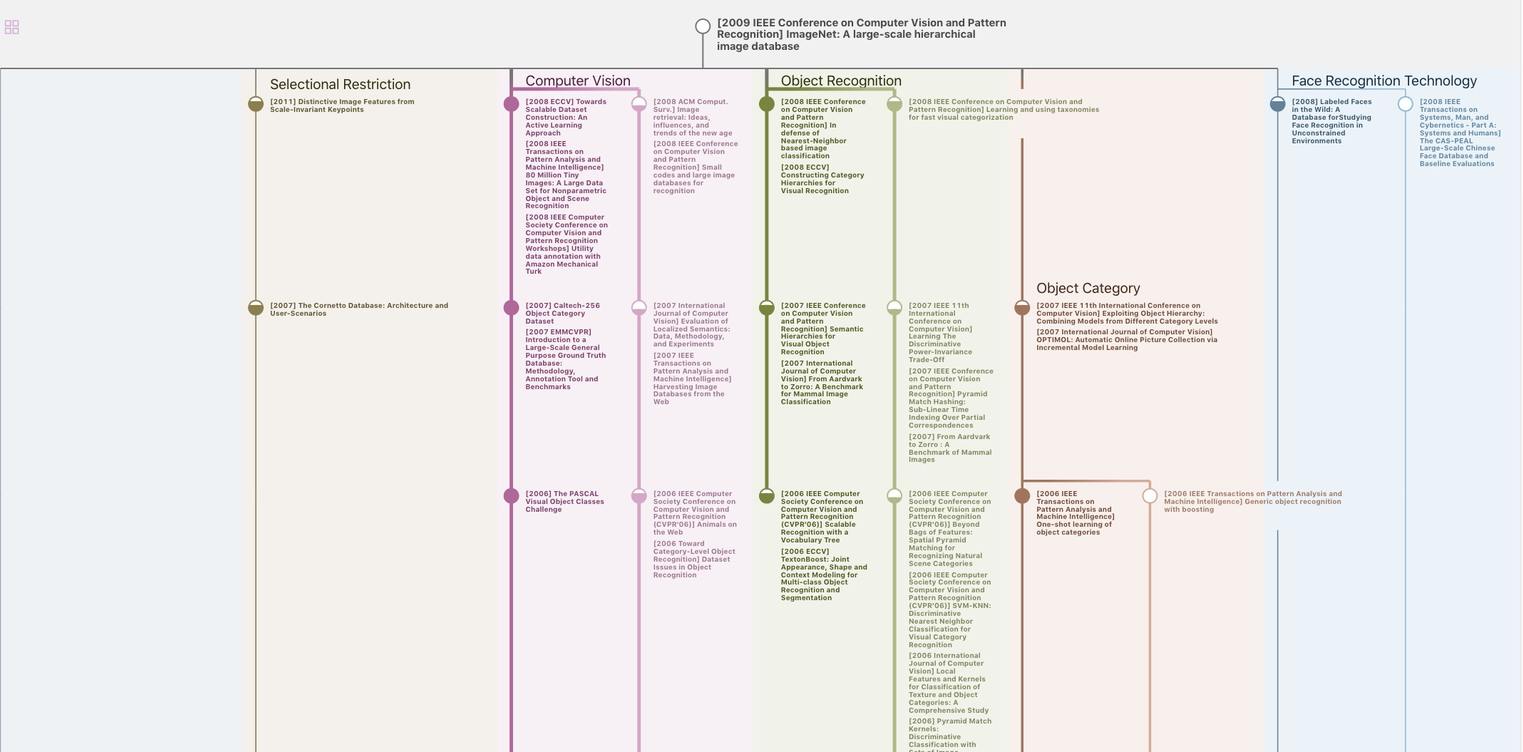
生成溯源树,研究论文发展脉络
Chat Paper
正在生成论文摘要