A novel perspective on forecasting non-ferrous metals’ volatility: Integrating deep learning techniques with econometric models
Finance Research Letters(2023)
摘要
This study puts forward a new perspective on non-ferrous metals’ volatility prediction in the futures market. Two hybrid deep learning architectures are constructed by embedding assorted convolutional neural networks (CNN) into long short-term memory (LSTM) models, and combining the LSTM networks with various generalized autoregressive conditional heteroscedasticity (GARCH)-type models. We illustrate the numerical implementation of all proposed models on four non-ferrous metal indices. Our findings suggest that the GARCH-LSTM model outperforms other alternatives by examining diverse error metrics. This study marks a significant advancement in the application of integrated deep learning models to enhance the prediction performance of commodity volatility.
更多查看译文
关键词
Volatility, Nonferrous metals, GARCH, Deep learning
AI 理解论文
溯源树
样例
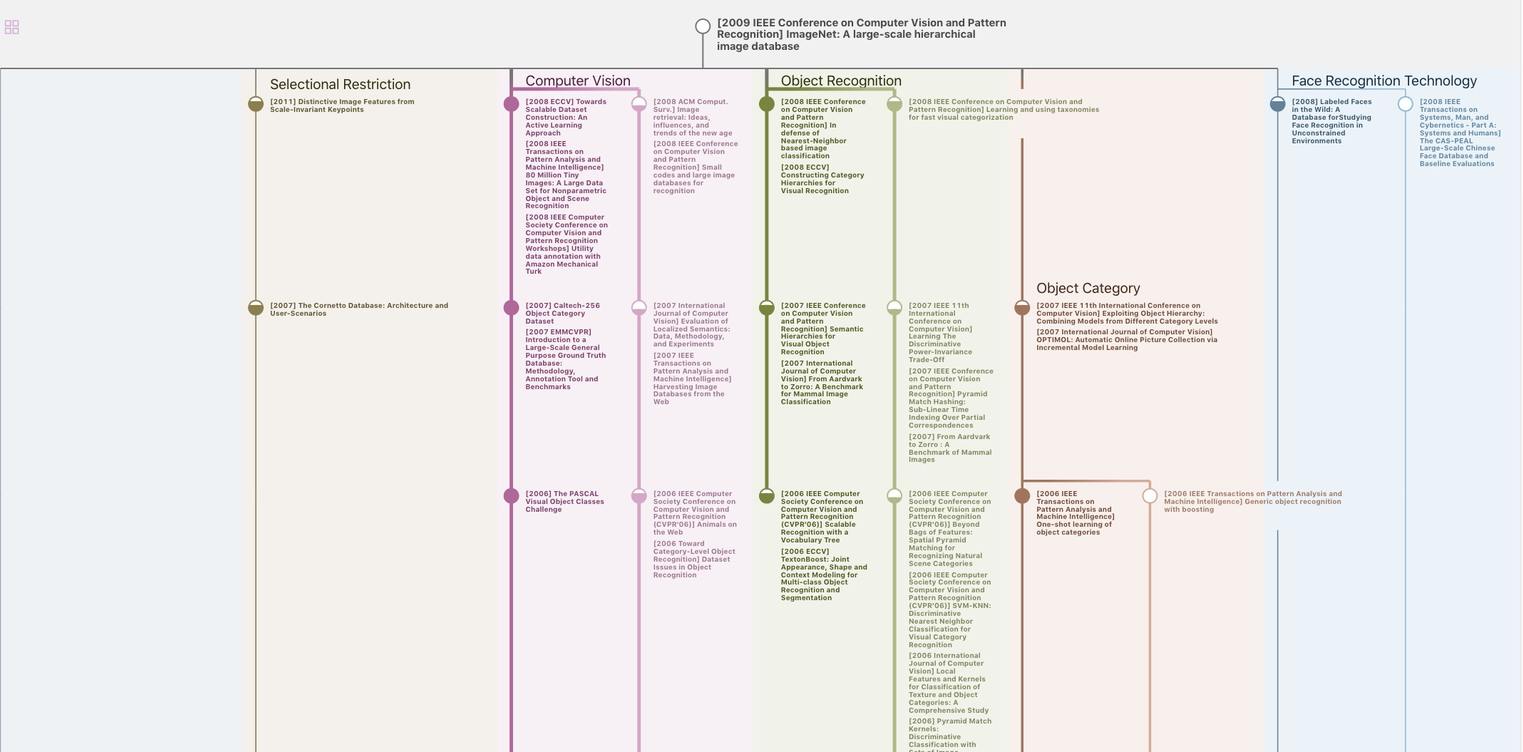
生成溯源树,研究论文发展脉络
Chat Paper
正在生成论文摘要